Pre-training with Aspect-Content Text Mutual Prediction for Multi-Aspect Dense Retrieval
PROCEEDINGS OF THE 32ND ACM INTERNATIONAL CONFERENCE ON INFORMATION AND KNOWLEDGE MANAGEMENT, CIKM 2023(2023)
摘要
Grounded on pre-trained language models (PLMs), dense retrieval has been studied extensively on plain text. In contrast, there has been little research on retrieving data with multiple aspects using dense models. In the scenarios such as product search, the aspect information plays an essential role in relevance matching, e.g., category: Electronics, Computers, and Pet Supplies. A common way of leveraging aspect information for multi-aspect retrieval is to introduce an auxiliary classification objective, i.e., using item contents to predict the annotated value IDs of item aspects. However, by learning the value embeddings from scratch, this approach may not capture the various semantic similarities between the values sufficiently. To address this limitation, we leverage the aspect information as text strings rather than class IDs during pre-training so that their semantic similarities can be naturally captured in the PLMs. To facilitate effective retrieval with the aspect strings, we propose mutual prediction objectives between the text of the item aspect and content. In this way, our model makes more sufficient use of aspect information than conducting undifferentiated masked language modeling (MLM) on the concatenated text of aspects and content. Extensive experiments on two real-world datasets (product and mini-program search) show that our approach can outperform competitive baselines both treating aspect values as classes and conducting the same MLM for aspect and content strings. Code and related dataset will be available at the URL (1).
更多查看译文
关键词
Dense Retrieval,Multi-Aspect,Pre-training
AI 理解论文
溯源树
样例
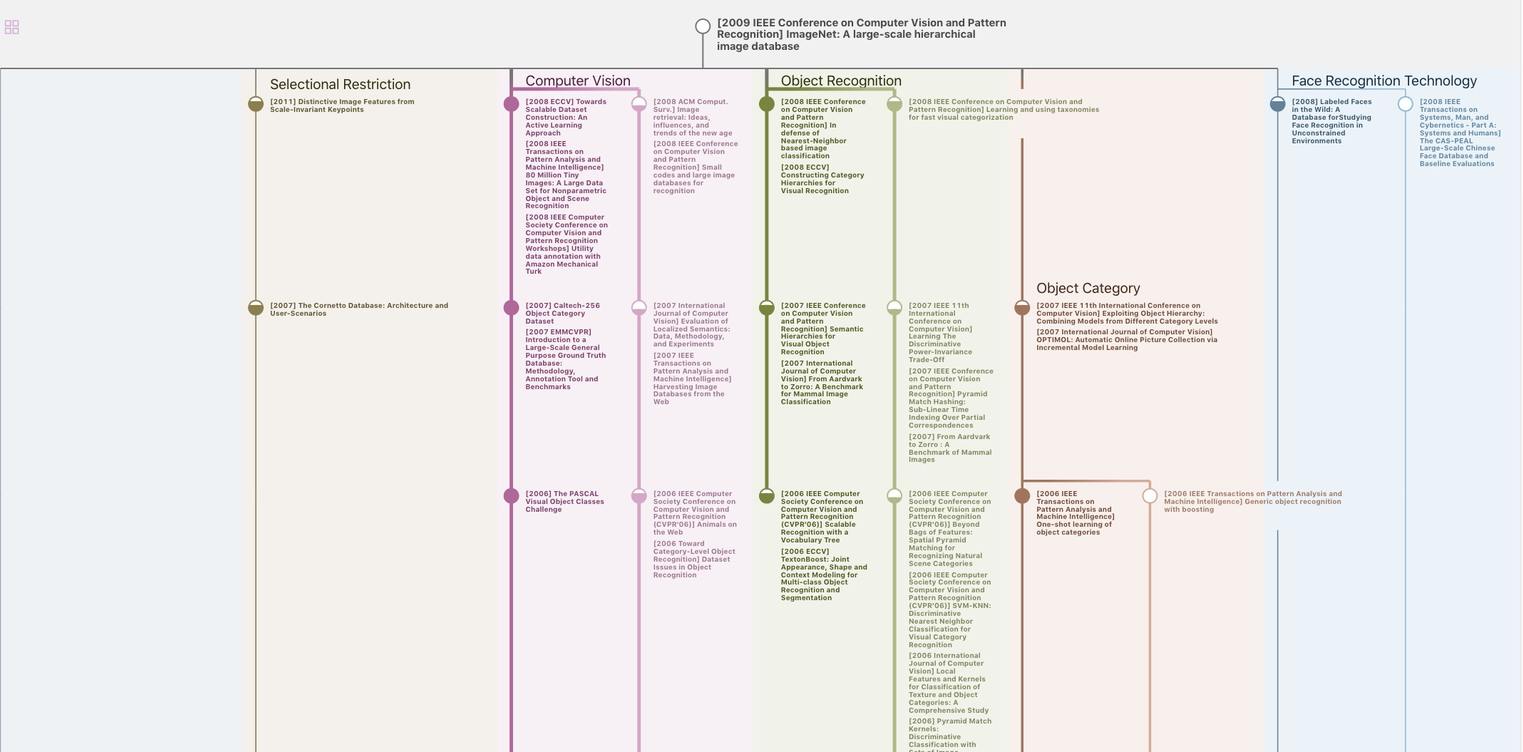
生成溯源树,研究论文发展脉络
Chat Paper
正在生成论文摘要