SegRNN: Segment Recurrent Neural Network for Long-Term Time Series Forecasting
CoRR(2023)
摘要
RNN-based methods have faced challenges in the Long-term Time Series Forecasting (LTSF) domain when dealing with excessively long look-back windows and forecast horizons. Consequently, the dominance in this domain has shifted towards Transformer, MLP, and CNN approaches. The substantial number of recurrent iterations are the fundamental reasons behind the limitations of RNNs in LTSF. To address these issues, we propose two novel strategies to reduce the number of iterations in RNNs for LTSF tasks: Segment-wise Iterations and Parallel Multi-step Forecasting (PMF). RNNs that combine these strategies, namely SegRNN, significantly reduce the required recurrent iterations for LTSF, resulting in notable improvements in forecast accuracy and inference speed. Extensive experiments demonstrate that SegRNN not only outperforms SOTA Transformer-based models but also reduces runtime and memory usage by more than 78%. These achievements provide strong evidence that RNNs continue to excel in LTSF tasks and encourage further exploration of this domain with more RNN-based approaches. The source code is coming soon.
更多查看译文
关键词
segment recurrent neural network,forecasting,neural network,time series
AI 理解论文
溯源树
样例
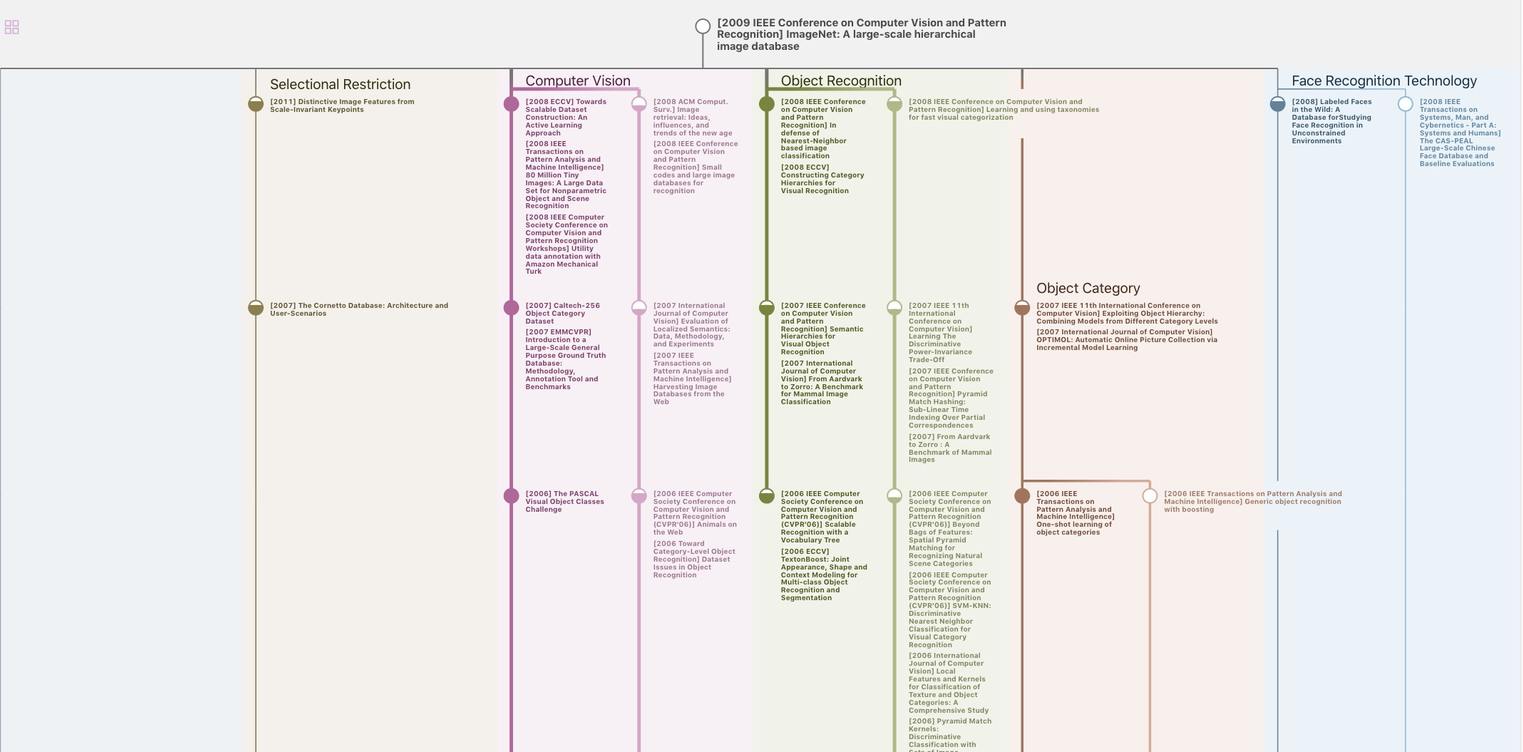
生成溯源树,研究论文发展脉络
Chat Paper
正在生成论文摘要