Unsupervised video rain streaks removal with deep foreground-background modeling
JOURNAL OF COMPUTATIONAL AND APPLIED MATHEMATICS(2024)
摘要
Outdoor video rain streaks removal is an important inverse problem in video processing that benefits subsequent applications. Traditional methods utilize prior information with interpretable domain knowledge while they are not tenable to capture complex structures of real-world videos. Deep learning methods learn a deraining mapping with a large model capacity brought by deep neural networks and their performances highly depend on the volume and diversity of training data. To address the challenging video deraining problem, we suggest an unsupervised video rain streaks removal method by solely using the observed rainy video. For the complex clean video, inspired by the classical foreground-background decomposition, we employ a deep convolutional neural network to capture the moving foreground and a disentangled deep spatial-temporal network with an affine operator to capture the underlying low-rank structure of the dynamic background. The foreground and background components are well balanced by a learnable probability mask. For structured rain streaks, we introduce a learnable total variation regularization whose parameters (i.e., rain directions) can be unsupervisedly learned. The deep modeling of the complex clean video and the simple yet effective modeling of structured rain streaks under the physical interpretable decomposition framework, which benefit each other in nature, are organically integrated to boost the deraining performance. Extensive experiments on synthetic and real-world rainy videos demonstrate the superiority of our method over state-of-the-art traditional and deep learning-based video deraining methods.& COPY; 2023 Elsevier B.V. All rights reserved.
更多查看译文
关键词
Video processing,Rain streaks removal,Inverse problem,Unsupervised,Deep convolutional neural network
AI 理解论文
溯源树
样例
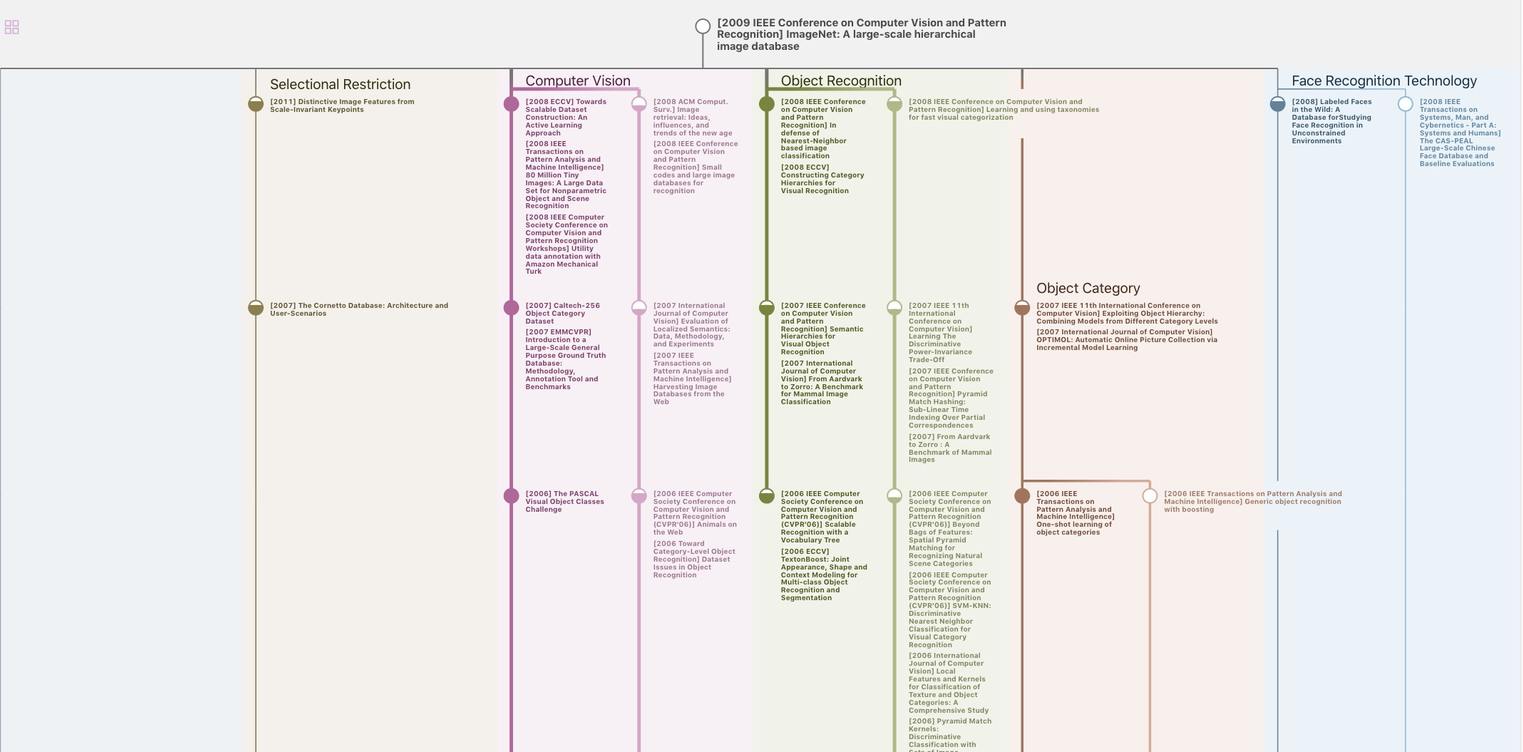
生成溯源树,研究论文发展脉络
Chat Paper
正在生成论文摘要