The Multivariate Bernoulli detector: Change point estimation in discrete survival analysis
arXiv (Cornell University)(2023)
摘要
Time-to-event data are often recorded on a discrete scale with multiple, competing risks as potential causes for the event. In this context, application of continuous survival analysis methods with a single risk suffer from biased estimation. Therefore, we propose the Multivariate Bernoulli detector for competing risks with discrete times involving a multivariate change point model on the cause-specific baseline hazards. Through the prior on the number of change points and their location, we impose dependence between change points across risks, as well as allowing for data-driven learning of their number. Then, conditionally on these change points, a Multivariate Bernoulli prior is used to infer which risks are involved. Focus of posterior inference is cause-specific hazard rates and dependence across risks. Such dependence is often present due to subject-specific changes across time that affect all risks. Full posterior inference is performed through a tailored local-global Markov chain Monte Carlo (MCMC) algorithm, which exploits a data augmentation trick and MCMC updates from non-conjugate Bayesian nonparametric methods. We illustrate our model in simulations and on prostate cancer data, comparing its performance with existing approaches.
更多查看译文
关键词
multivariate bernoulli detector,change point estimation,survival
AI 理解论文
溯源树
样例
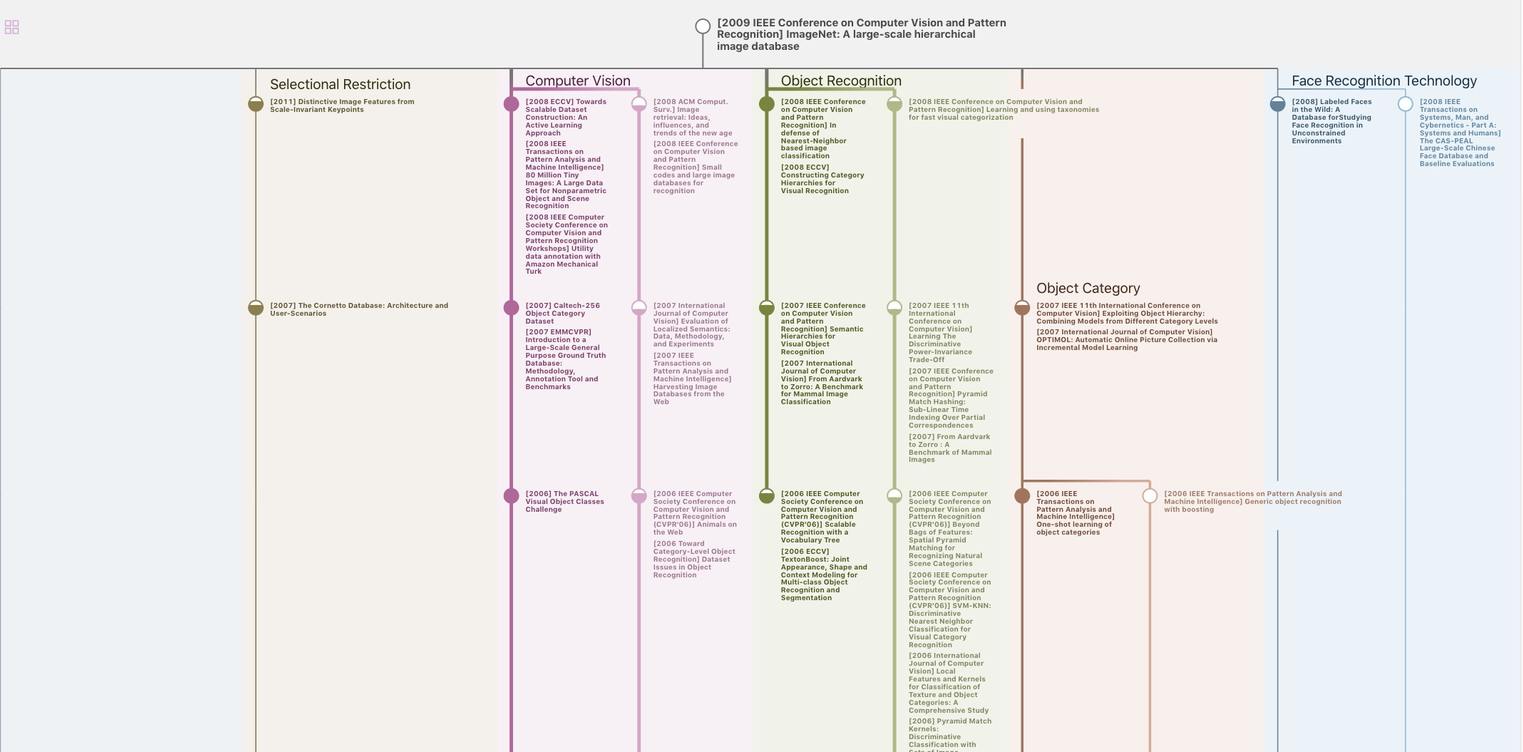
生成溯源树,研究论文发展脉络
Chat Paper
正在生成论文摘要