Computable Bounds on Convergence of Markov Chains in Wasserstein Distance
arXiv (Cornell University)(2023)
摘要
We introduce a unified framework to estimate the convergence of Markov chains to equilibrium using Wasserstein distance. The framework provides convergence bounds with various rates, ranging from polynomial to exponential, all derived from a single contractive drift condition. This approach removes the need for finding a specific set with drift outside and contraction inside. The convergence bounds are explicit, as they can be estimated based on one-step expectations and do not rely on equilibrium-related quantities. To enhance the applicability of the framework, we introduce the large M technique and the boundary removal technique. We illustrate these methods in queueing models and algorithms in stochastic optimization.
更多查看译文
关键词
computable bounds,wasserstein
AI 理解论文
溯源树
样例
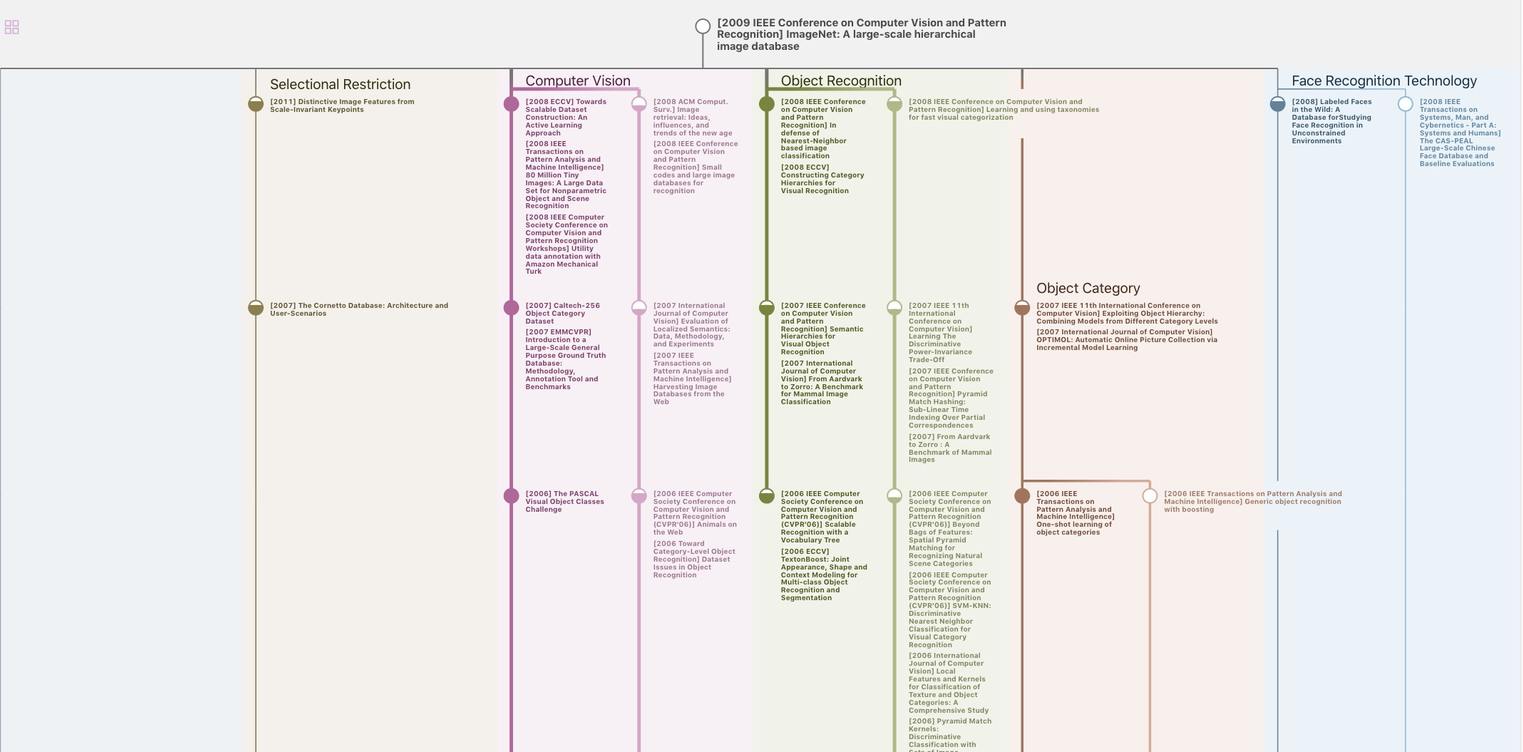
生成溯源树,研究论文发展脉络
Chat Paper
正在生成论文摘要