Improving Adversarial Robustness of Masked Autoencoders via Test-time Frequency-domain Prompting
CoRR(2023)
摘要
In this paper, we investigate the adversarial robustness of vision transformers that are equipped with BERT pretraining (\eg, BEiT, MAE). A surprising observation is that MAE has significantly worse adversarial robustness than other BERT pretraining methods. This observation drives us to rethink the basic differences between these BERT pretraining methods and how these differences affect the robustness against adversarial perturbations. Our empirical analysis reveals that the adversarial robustness of BERT pretraining is highly related to the reconstruction target, \ie, predicting the raw pixels of masked image patches will degrade more adversarial robustness of the model than predicting the semantic context, since it guides the model to concentrate more on medium-/high-frequency components of images. Based on our analysis, we provide a simple yet effective way to boost the adversarial robustness of MAE. The basic idea is using the dataset-extracted domain knowledge to occupy the medium-/high-frequency of images, thus narrowing the optimization space of adversarial perturbations. Specifically, we group the distribution of pretraining data and optimize a set of cluster-specific visual prompts on frequency domain. These prompts are incorporated with input images through prototype-based prompt selection during test period. Extensive evaluation shows that our method clearly boost MAE's adversarial robustness while maintaining its clean performance on ImageNet-1k classification. Our code is available at: \href{https://github.com/shikiw/RobustMAE}{https://github.com/shikiw/RobustMAE}.
更多查看译文
关键词
masked autoencoders,adversarial robustness,test-time,frequency-domain
AI 理解论文
溯源树
样例
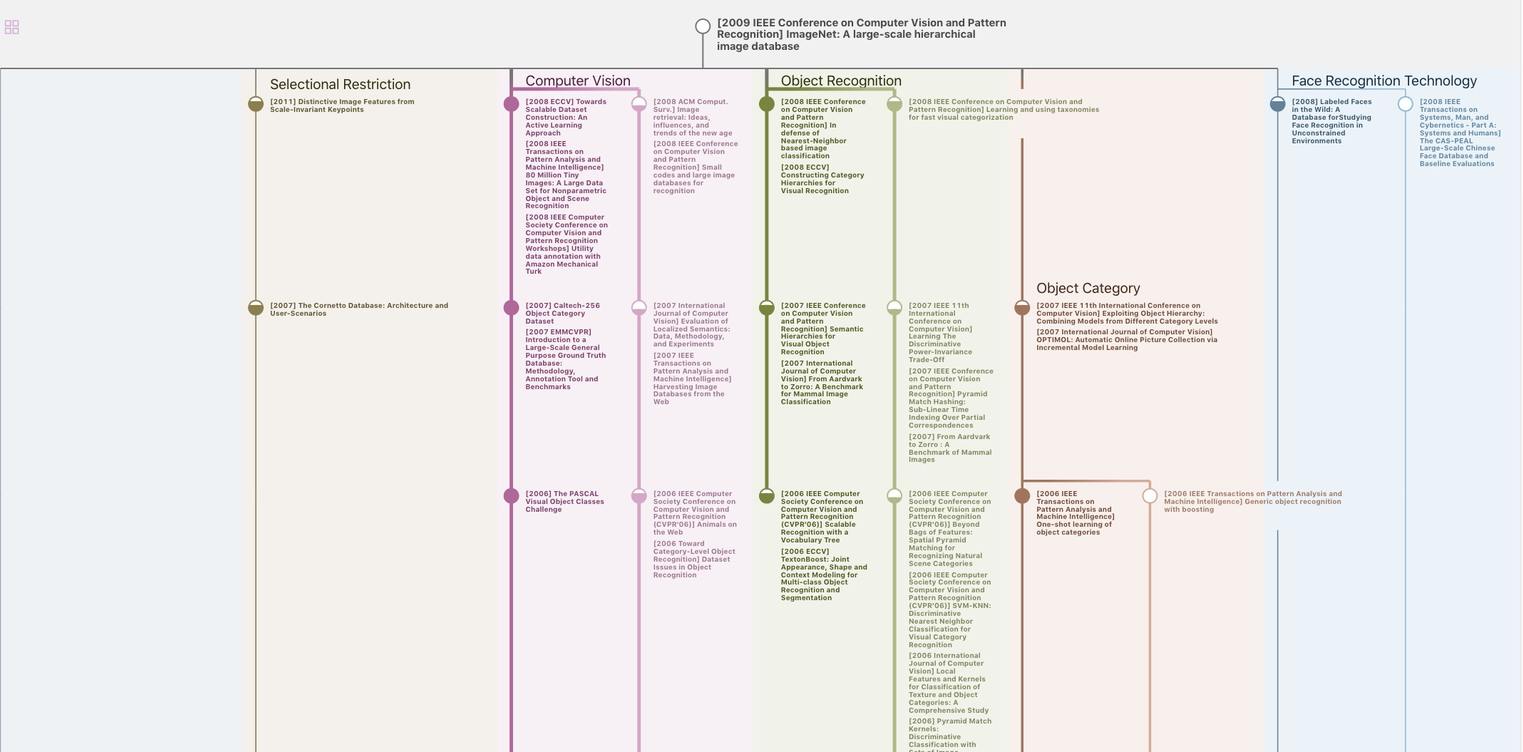
生成溯源树,研究论文发展脉络
Chat Paper
正在生成论文摘要