GPFL: Simultaneously Learning Global and Personalized Feature Information for Personalized Federated Learning
CoRR(2023)
摘要
Federated Learning (FL) is popular for its privacy-preserving and collaborative learning capabilities. Recently, personalized FL (pFL) has received attention for its ability to address statistical heterogeneity and achieve personalization in FL. However, from the perspective of feature extraction, most existing pFL methods only focus on extracting global or personalized feature information during local training, which fails to meet the collaborative learning and personalization goals of pFL. To address this, we propose a new pFL method, named GPFL, to simultaneously learn global and personalized feature information on each client. We conduct extensive experiments on six datasets in three statistically heterogeneous settings and show the superiority of GPFL over ten state-of-the-art methods regarding effectiveness, scalability, fairness, stability, and privacy. Besides, GPFL mitigates overfitting and outperforms the baselines by up to 8.99% in accuracy.
更多查看译文
关键词
personalized feature,global,learning
AI 理解论文
溯源树
样例
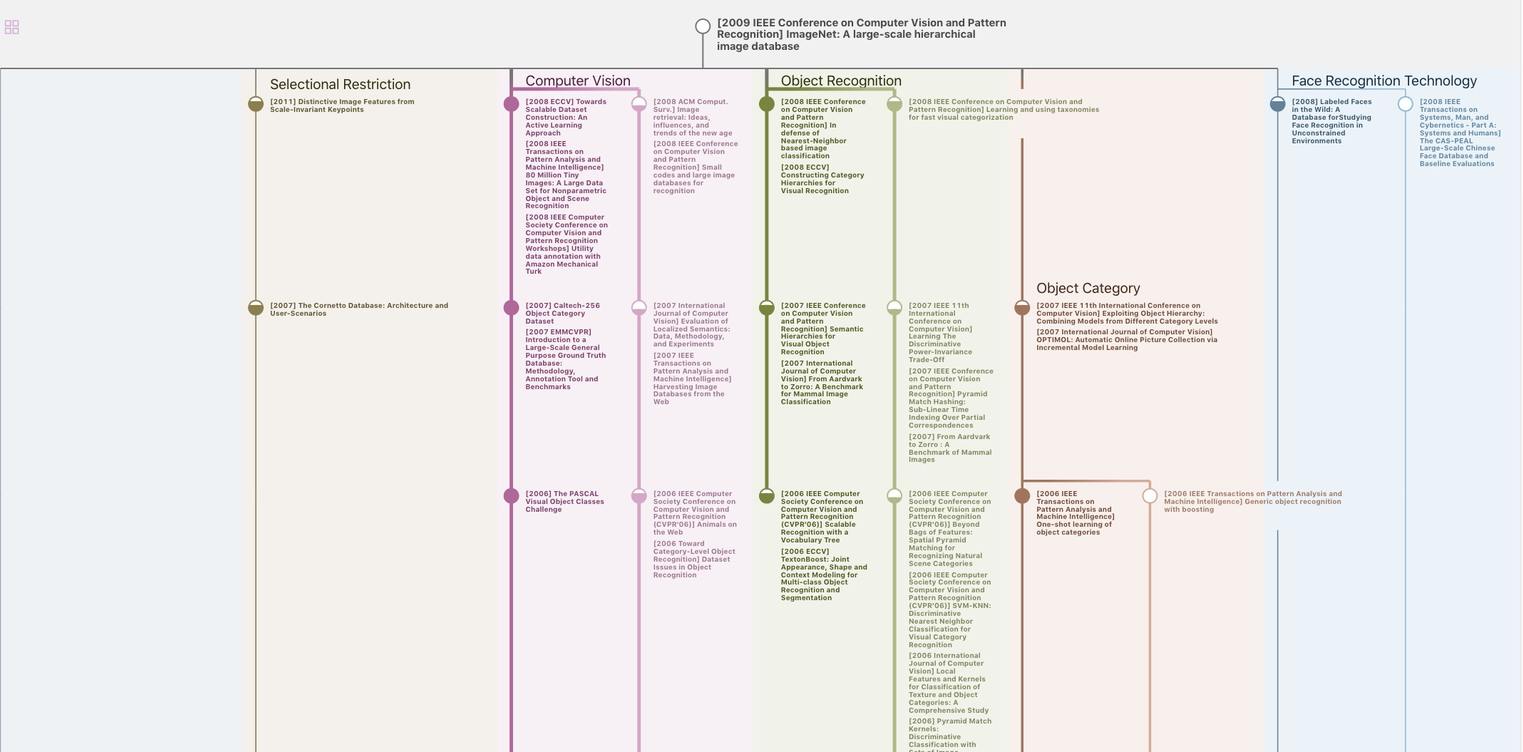
生成溯源树,研究论文发展脉络
Chat Paper
正在生成论文摘要