A Two-Stage Deep-Learning Model for Detection and Occlusion-Based Classification of Kashmiri Orchard Apples for Robotic Harvesting
Journal of Biosystems Engineering(2023)
摘要
Purpose The process of robotic harvesting has revolutionized the agricultural industry, allowing for more efficient and cost-effective fruit picking. Developing algorithms for accurate fruit detection is essential for vision-based robotic harvesting of apples. Although deep-learning techniques are popularly used for apple detection, the development of robust models that can accord information about the fruit’s occlusion condition is important to plan a suitable strategy for end-effector manipulation. Apples on the tree experience occlusions due to leaves, stems (branches), trellis wire, or other fruits during robotic harvesting. Methods A novel two-stage deep-learning-based approach is proposed and successfully demonstrated for detecting on-tree apples and identifying their occlusion condition. In the first stage, the system employs a cutting-edge YOLOv7 model, meticulously trained on a custom Kashmiri apple orchard image dataset. The second stage of the approach utilize the powerful EfficientNet-B0 model; the system is able to classify the apples into four distinct categories based on their occlusion condition, namely, non-occluded, leaf-occluded, stem/wire-occluded, and apple-occluded apples. Results The YOLOv7 model achieved an average precision of 0.902 and an F1-score of 0.905 on a test set for detecting apples. The size of the trained weights and detection speed were observed to be 284 MB and 0.128 s per image. The classification model produced an overall accuracy of 92.22% with F1-scores of 94.64%, 90.91%, 86.87%, and 90.25% for non-occluded, leaf-occluded, stem/wire-occluded, and apple-occluded apple classes, respectively. Conclusion This study proposes a novel two-stage model for the simultaneous detection of on-tree apples and classify them based on occlusion conditions, which could improve the effectiveness of autonomous apple harvesting and avoid potential damage to the end-effector due to the objects causing the occlusion.
更多查看译文
关键词
Fruit detection,YOLOv7,Classification,EfficientNet-B0,Robotic harvesting
AI 理解论文
溯源树
样例
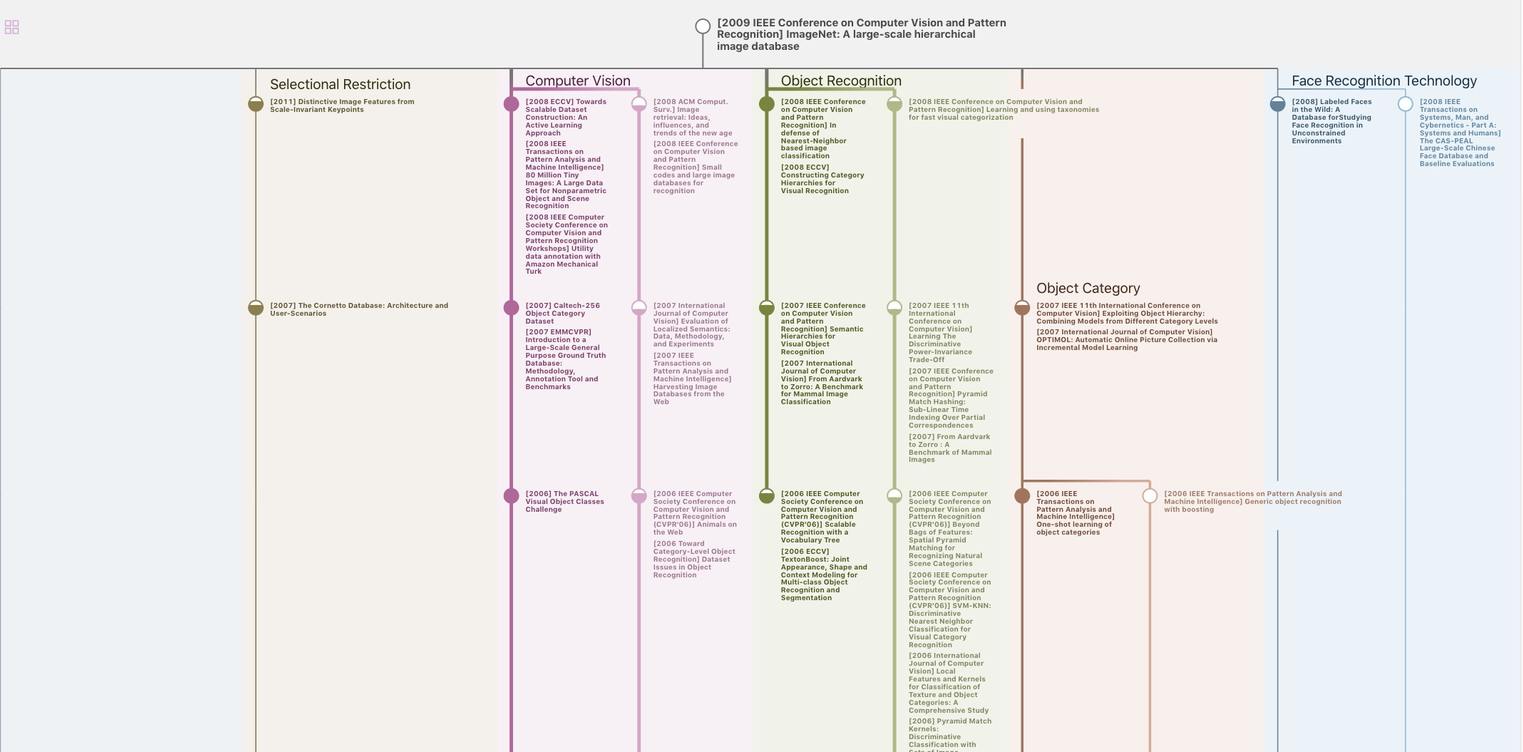
生成溯源树,研究论文发展脉络
Chat Paper
正在生成论文摘要