Audiovisual Moments in Time: A large-scale annotated dataset of audiovisual actions.
PloS one(2024)
摘要
We present Audiovisual Moments in Time (AVMIT), a large-scale dataset of audiovisual action events. In an extensive annotation task 11 participants labelled a subset of 3-second audiovisual videos from the Moments in Time dataset (MIT). For each trial, participants assessed whether the labelled audiovisual action event was present and whether it was the most prominent feature of the video. The dataset includes the annotation of 57,177 audiovisual videos, each independently evaluated by 3 of 11 trained participants. From this initial collection, we created a curated test set of 16 distinct action classes, with 60 videos each (960 videos). We also offer 2 sets of pre-computed audiovisual feature embeddings, using VGGish/YamNet for audio data and VGG16/EfficientNetB0 for visual data, thereby lowering the barrier to entry for audiovisual DNN research. We explored the advantages of AVMIT annotations and feature embeddings to improve performance on audiovisual event recognition. A series of 6 Recurrent Neural Networks (RNNs) were trained on either AVMIT-filtered audiovisual events or modality-agnostic events from MIT, and then tested on our audiovisual test set. In all RNNs, top 1 accuracy was increased by 2.71-5.94% by training exclusively on audiovisual events, even outweighing a three-fold increase in training data. Additionally, we introduce the Supervised Audiovisual Correspondence (SAVC) task whereby a classifier must discern whether audio and visual streams correspond to the same action label. We trained 6 RNNs on the SAVC task, with or without AVMIT-filtering, to explore whether AVMIT is helpful for cross-modal learning. In all RNNs, accuracy improved by 2.09-19.16% with AVMIT-filtered data. We anticipate that the newly annotated AVMIT dataset will serve as a valuable resource for research and comparative experiments involving computational models and human participants, specifically when addressing research questions where audiovisual correspondence is of critical importance.
更多查看译文
AI 理解论文
溯源树
样例
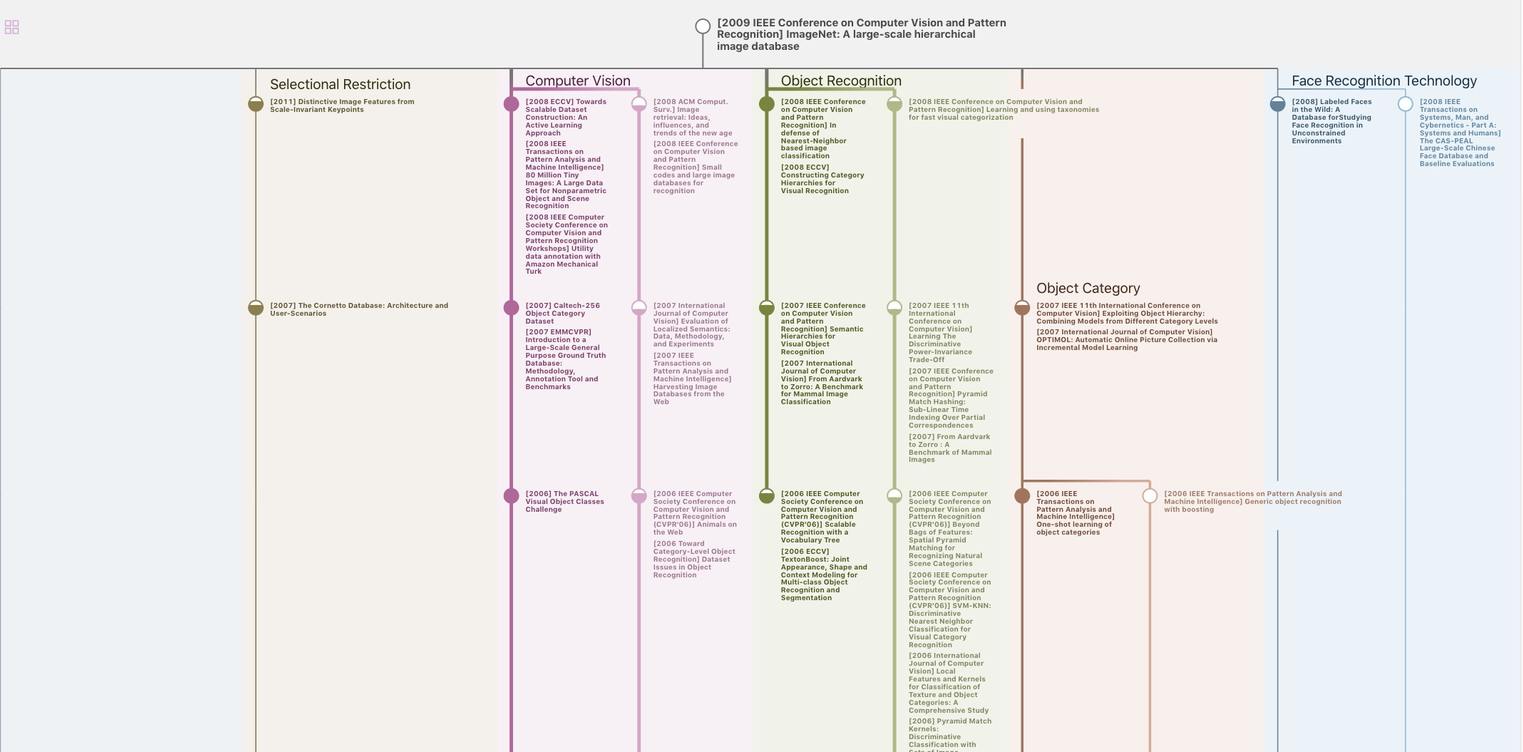
生成溯源树,研究论文发展脉络
Chat Paper
正在生成论文摘要