Investigation of Architectures and Receptive Fields for Appearance-based Gaze Estimation
CoRR(2023)
摘要
With the rapid development of deep learning technology in the past decade, appearance-based gaze estimation has attracted great attention from both computer vision and human-computer interaction research communities. Fascinating methods were proposed with variant mechanisms including soft attention, hard attention, two-eye asymmetry, feature disentanglement, rotation consistency, and contrastive learning. Most of these methods take the single-face or multi-region as input, yet the basic architecture of gaze estimation has not been fully explored. In this paper, we reveal the fact that tuning a few simple parameters of a ResNet architecture can outperform most of the existing state-of-the-art methods for the gaze estimation task on three popular datasets. With our extensive experiments, we conclude that the stride number, input image resolution, and multi-region architecture are critical for the gaze estimation performance while their effectiveness dependent on the quality of the input face image. We obtain the state-of-the-art performances on three datasets with 3.64 on ETH-XGaze, 4.50 on MPIIFaceGaze, and 9.13 on Gaze360 degrees gaze estimation error by taking ResNet-50 as the backbone.
更多查看译文
关键词
receptive fields,architectures,appearance-based
AI 理解论文
溯源树
样例
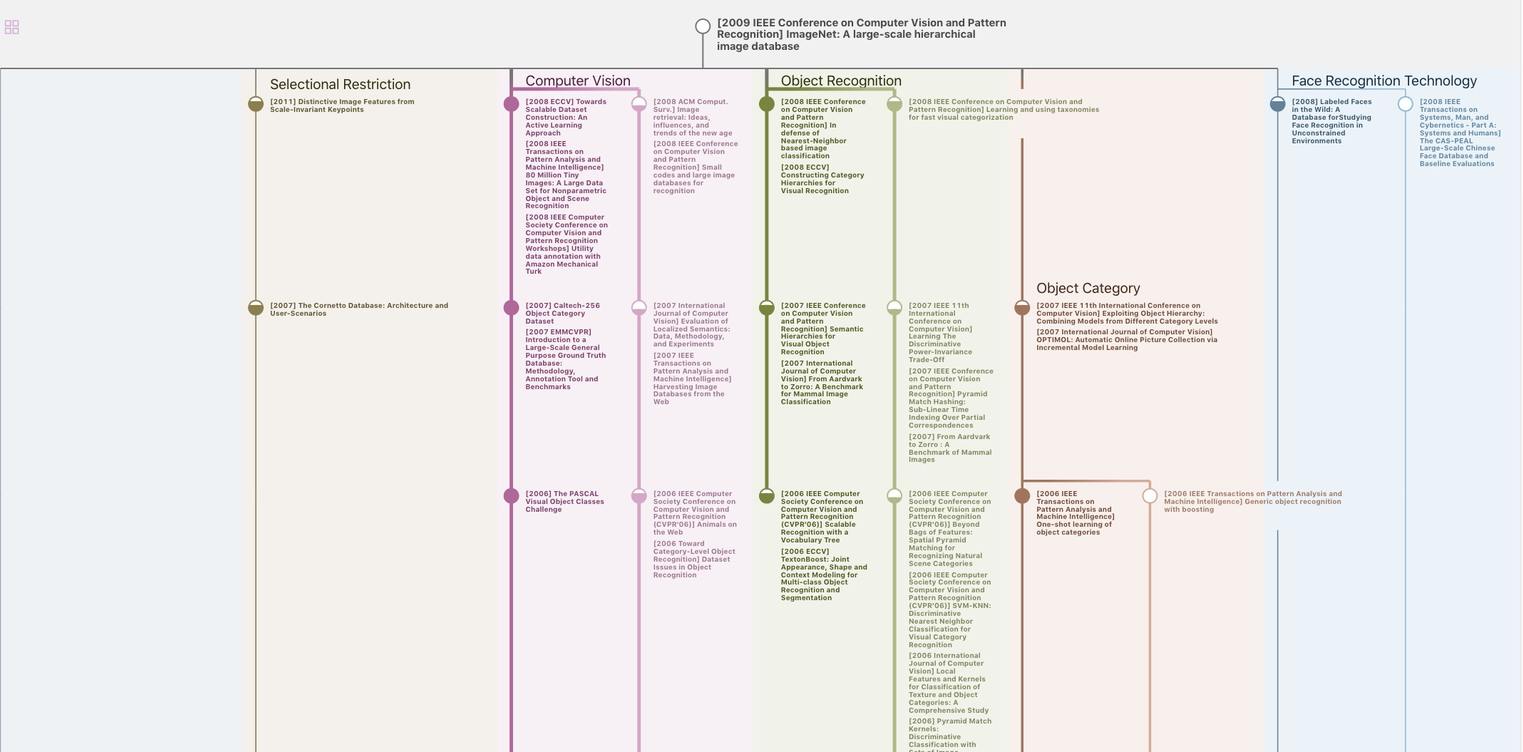
生成溯源树,研究论文发展脉络
Chat Paper
正在生成论文摘要