PUMGPT: A Large Vision-Language Model for Product Understanding
CoRR(2023)
摘要
Recent developments of multi-modal large language models have demonstrated its strong ability in solving vision-language tasks. In this paper, we focus on the product understanding task, which plays an essential role in enhancing online shopping experience. Product understanding task includes a variety of sub-tasks, which require models to respond diverse queries based on multi-modal product information. Traditional methods design distinct model architectures for each sub-task. On the contrary, we present PUMGPT, a large vision-language model aims at unifying all product understanding tasks under a singular model structure. To bridge the gap between vision and text representations, we propose Layer-wise Adapters (LA), an approach that provides enhanced alignment with fewer visual tokens and enables parameter-efficient fine-tuning. Moreover, the inherent parameter-efficient fine-tuning ability allows PUMGPT to be readily adapted to new product understanding tasks and emerging products. We design instruction templates to generate diverse product instruction datasets. Simultaneously, we utilize open-domain datasets during training to improve the performance of PUMGPT and its generalization ability. Through extensive evaluations, PUMGPT demonstrates its superior performance across multiple product understanding tasks, including product captioning, category question-answering, attribute extraction, attribute question-answering, and even free-form question-answering about products.
更多查看译文
关键词
product understanding,vision-language
AI 理解论文
溯源树
样例
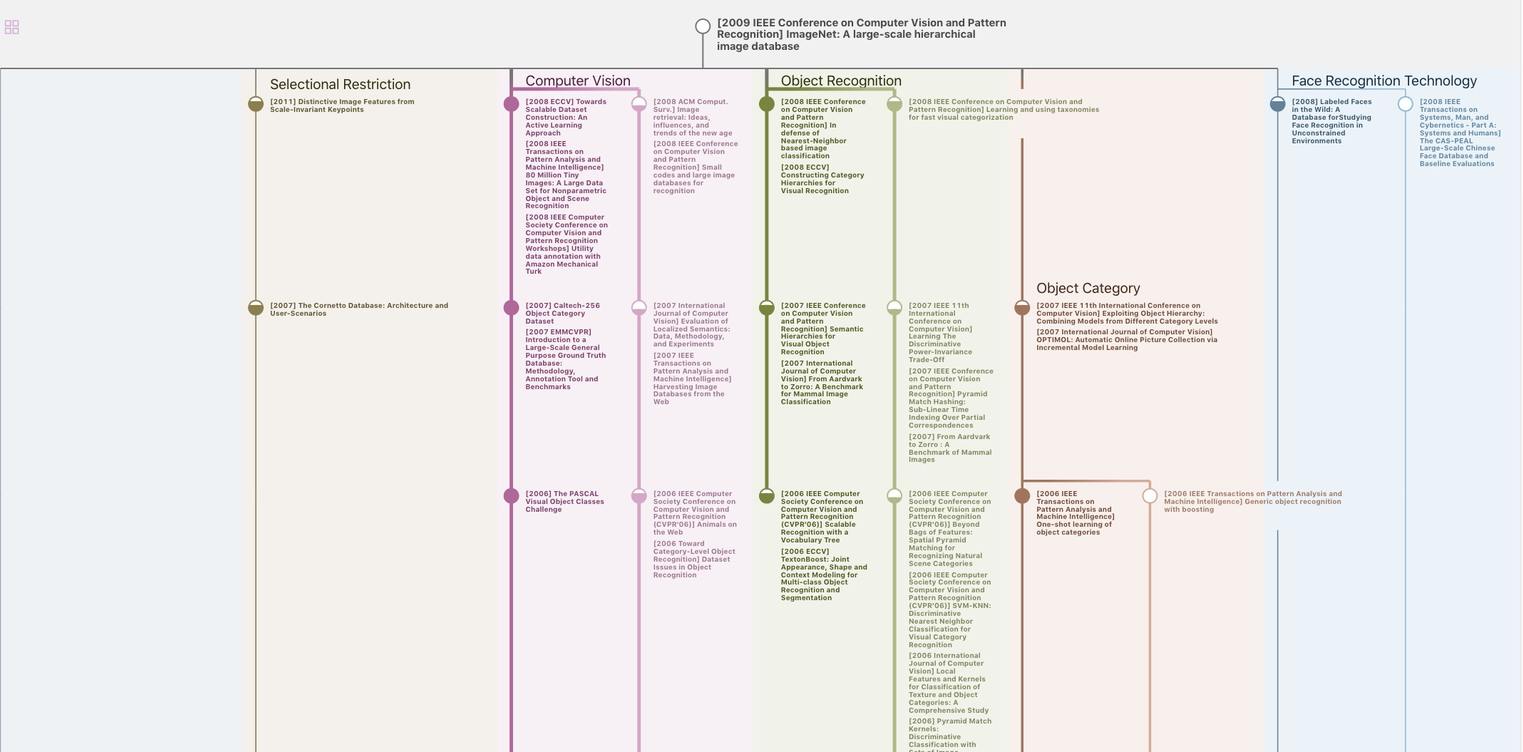
生成溯源树,研究论文发展脉络
Chat Paper
正在生成论文摘要