MATLABER: Material-Aware Text-to-3D via LAtent BRDF auto-EncodeR
CoRR(2023)
摘要
Based on powerful text-to-image diffusion models, text-to-3D generation has made significant progress in generating compelling geometry and appearance. However, existing methods still struggle to recover high-fidelity object materials, either only considering Lambertian reflectance, or failing to disentangle BRDF materials from the environment lights. In this work, we propose Material-Aware Text-to-3D via LAtent BRDF auto-EncodeR (\textbf{MATLABER}) that leverages a novel latent BRDF auto-encoder for material generation. We train this auto-encoder with large-scale real-world BRDF collections and ensure the smoothness of its latent space, which implicitly acts as a natural distribution of materials. During appearance modeling in text-to-3D generation, the latent BRDF embeddings, rather than BRDF parameters, are predicted via a material network. Through exhaustive experiments, our approach demonstrates the superiority over existing ones in generating realistic and coherent object materials. Moreover, high-quality materials naturally enable multiple downstream tasks such as relighting and material editing. Code and model will be publicly available at \url{https://sheldontsui.github.io/projects/Matlaber}.
更多查看译文
关键词
matlaber,material-aware,auto-encoder
AI 理解论文
溯源树
样例
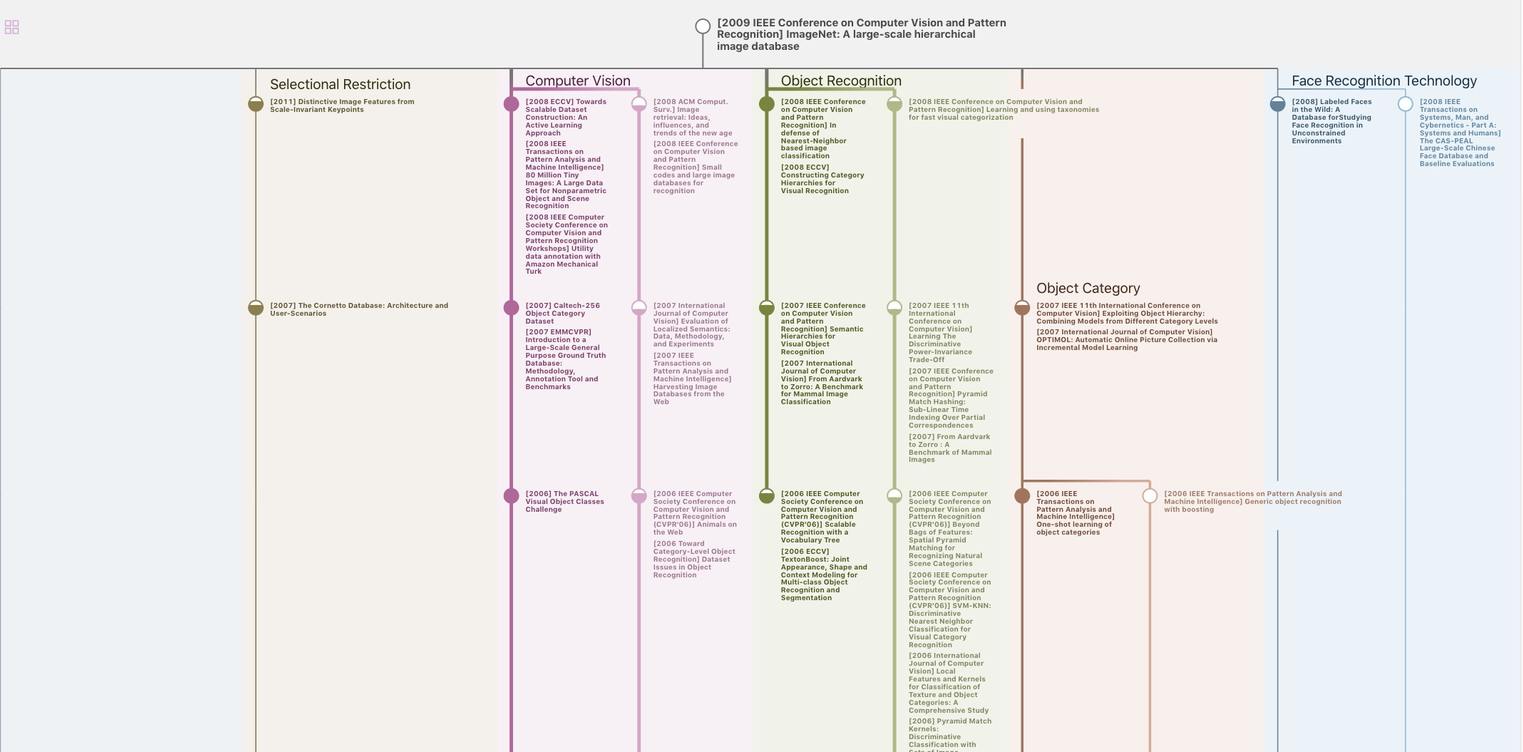
生成溯源树,研究论文发展脉络
Chat Paper
正在生成论文摘要