Learning in Cooperative Multiagent Systems Using Cognitive and Machine Models
ACM TRANSACTIONS ON AUTONOMOUS AND ADAPTIVE SYSTEMS(2023)
摘要
Developing effective multi-agent systems (MASs) is critical for many applications requiring collaboration and coordination with humans. Despite the rapid advance of multi-agent deep reinforcement learning (MADRL) in cooperative MASs, one of the major challenges that remain is the simultaneous learning and interaction of independent agents in dynamic environments in the presence of stochastic rewards. State-of-the-art MADRL models struggle to perform well in Coordinated Multi-agent Object Transportation Problems (CMOTPs) wherein agents must coordinate with each other and learn from stochastic rewards. In contrast, humans often learn rapidly to adapt to non-stationary environments that require coordination among people. In this article, motivated by the demonstrated ability of cognitive models based on Instance-based Learning Theory (IBLT) to capture human decisions in many dynamic decision-making tasks, we propose three variants of multi-agent IBL models (MAIBLs). The idea of these MAIBL algorithms is to combine the cognitive mechanisms of IBLT and the techniques of MADRL models to deal with coordination MASs in stochastic environments from the perspective of independent learners. We demonstrate that the MAIBL models exhibit faster learning and achieve better coordination in a dynamic CMOTP task with various settings of stochastic rewards compared to current MADRL models. We discuss the benefits of integrating cognitive insights into MADRL models.
更多查看译文
关键词
Coordination problems,instance-based learning theory,multi-agent reinforcement learning,multi-agent instance-based learning
AI 理解论文
溯源树
样例
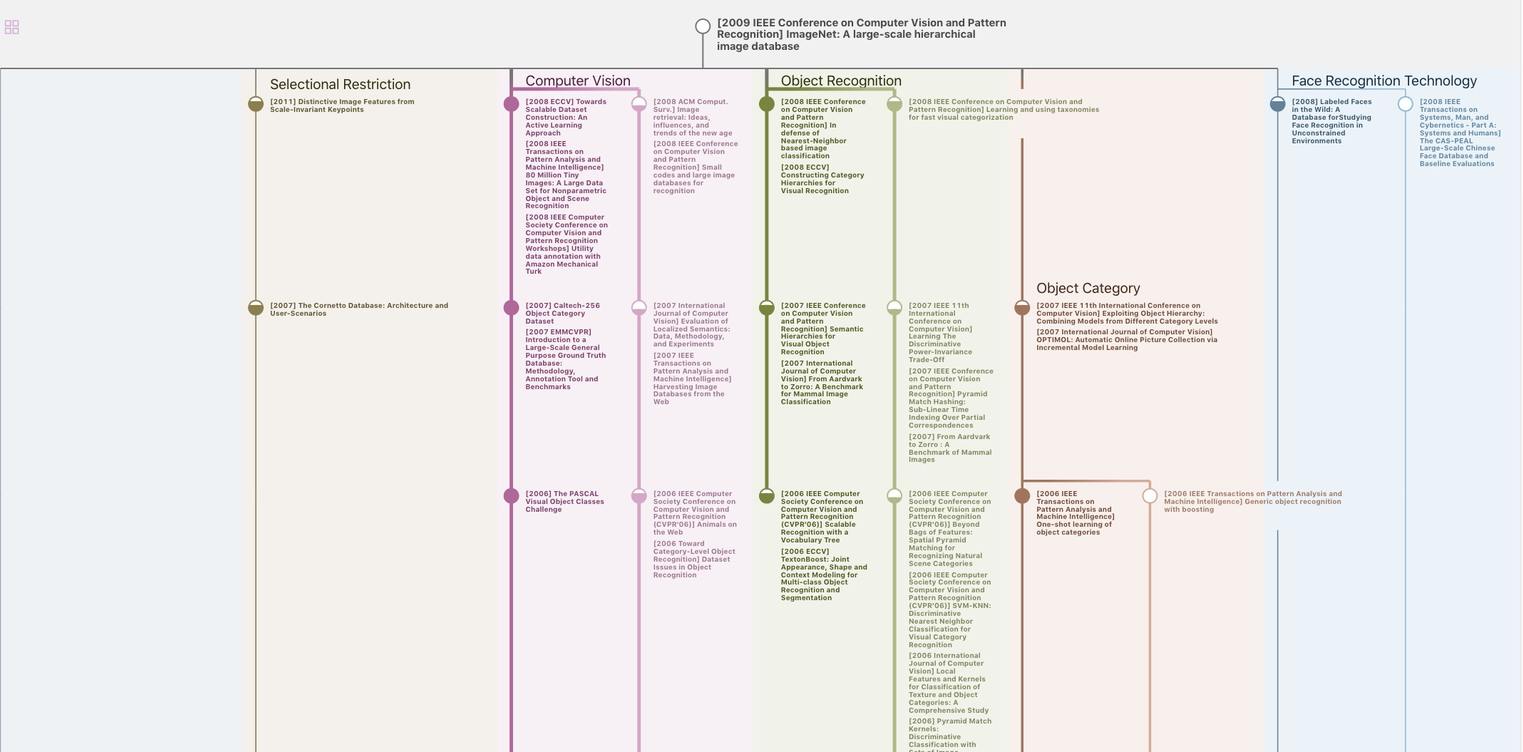
生成溯源树,研究论文发展脉络
Chat Paper
正在生成论文摘要