Polynomial Bounds for Learning Noisy Optical Physical Unclonable Functions and Connections to Learning With Errors
CoRR(2023)
摘要
It is shown that a class of optical physical unclonable functions (PUFs) can be learned to arbitrary precision with arbitrarily high probability, even in the presence of noise, given access to polynomially many challenge-response pairs and polynomially bounded computational power, under mild assumptions about the distributions of the noise and challenge vectors. This extends the results of Rh\"uramir et al. (2013), who showed a subset of this class of PUFs to be learnable in polynomial time in the absence of noise, under the assumption that the optics of the PUF were either linear or had negligible nonlinear effects. We derive polynomial bounds for the required number of samples and the computational complexity of a linear regression algorithm, based on size parameters of the PUF, the distributions of the challenge and noise vectors, and the probability and accuracy of the regression algorithm, with a similar analysis to one done by Bootle et al. (2018), who demonstrated a learning attack on a poorly implemented version of the Learning With Errors problem.
更多查看译文
关键词
polynomial bounds,learning,functions
AI 理解论文
溯源树
样例
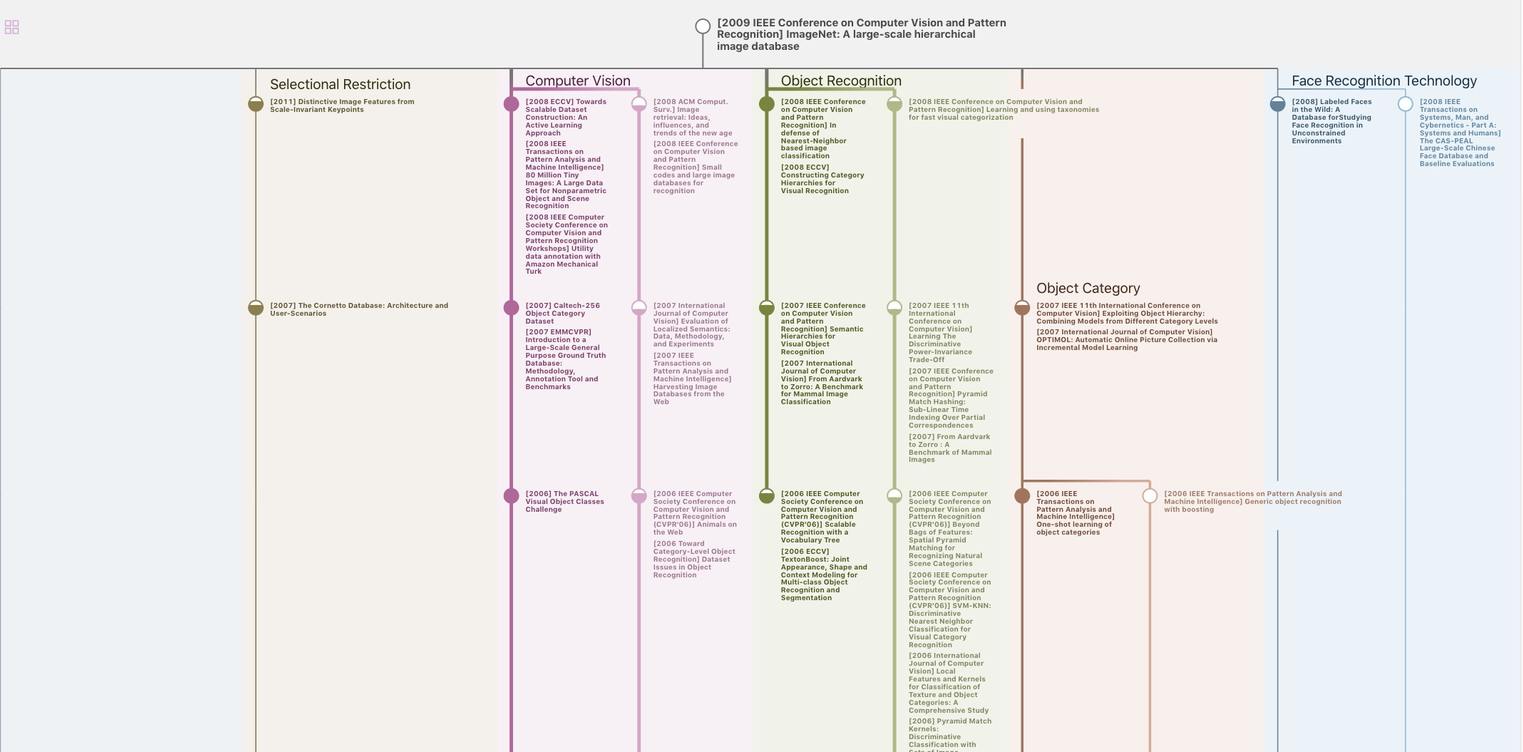
生成溯源树,研究论文发展脉络
Chat Paper
正在生成论文摘要