The Unreasonable Effectiveness of Large Language-Vision Models for Source-free Video Domain Adaptation
CoRR(2023)
摘要
Source-Free Video Unsupervised Domain Adaptation (SFVUDA) methods consists in the task of adapting an action recognition model, trained on a labelled source dataset, to an unlabelled target dataset, without accessing the actual source data. Previous approaches have attempted to address SFVUDA by leveraging self-supervision (e.g., enforcing temporal consistency) derived from the target data itself. In this work we take an orthogonal approach by exploiting "web-supervision" from Large Language-Vision Models (LLVMs), driven by the rationale that LLVMs contain rich world prior, which is surprisingly robust to domain-shift. We showcase the unreasonable effectiveness of integrating LLVMs for SFVUDA by devising an intuitive and parameter efficient method, which we name as Domain Adaptation with Large Language-Vision models (DALL-V), that distills the world prior and complementary source model information into a student network tailored for the target. Despite the simplicity, DALL-V achieves significant improvement over state-of-the-art SFVUDA methods.
更多查看译文
关键词
adaptation,language-vision,source-free
AI 理解论文
溯源树
样例
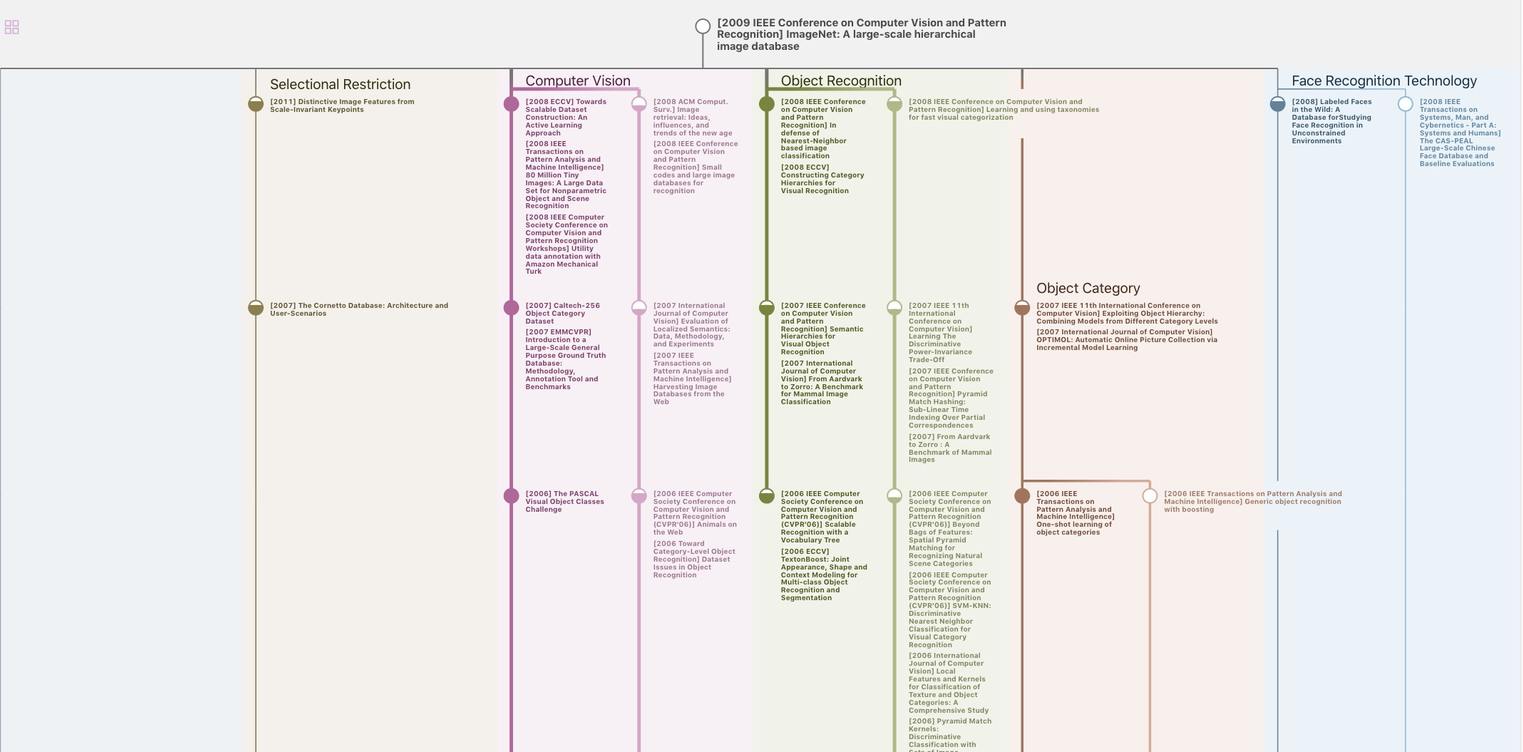
生成溯源树,研究论文发展脉络
Chat Paper
正在生成论文摘要