Interpretable Graph Neural Networks for Tabular Data
CoRR(2023)
摘要
Data in tabular format is frequently occurring in real-world applications. Graph Neural Networks (GNNs) have recently been extended to effectively handle such data, allowing feature interactions to be captured through representation learning. However, these approaches essentially produce black-box models, in the form of deep neural networks, precluding users from following the logic behind the model predictions. We propose an approach, called IGNNet (Interpretable Graph Neural Network for tabular data), which constrains the learning algorithm to produce an interpretable model, where the model shows how the predictions are exactly computed from the original input features. A large-scale empirical investigation is presented, showing that IGNNet is performing on par with state-of-the-art machine-learning algorithms that target tabular data, including XGBoost, Random Forests, and TabNet. At the same time, the results show that the explanations obtained from IGNNet are aligned with the true Shapley values of the features without incurring any additional computational overhead.
更多查看译文
关键词
interpretable graph neural networks,neural networks,data
AI 理解论文
溯源树
样例
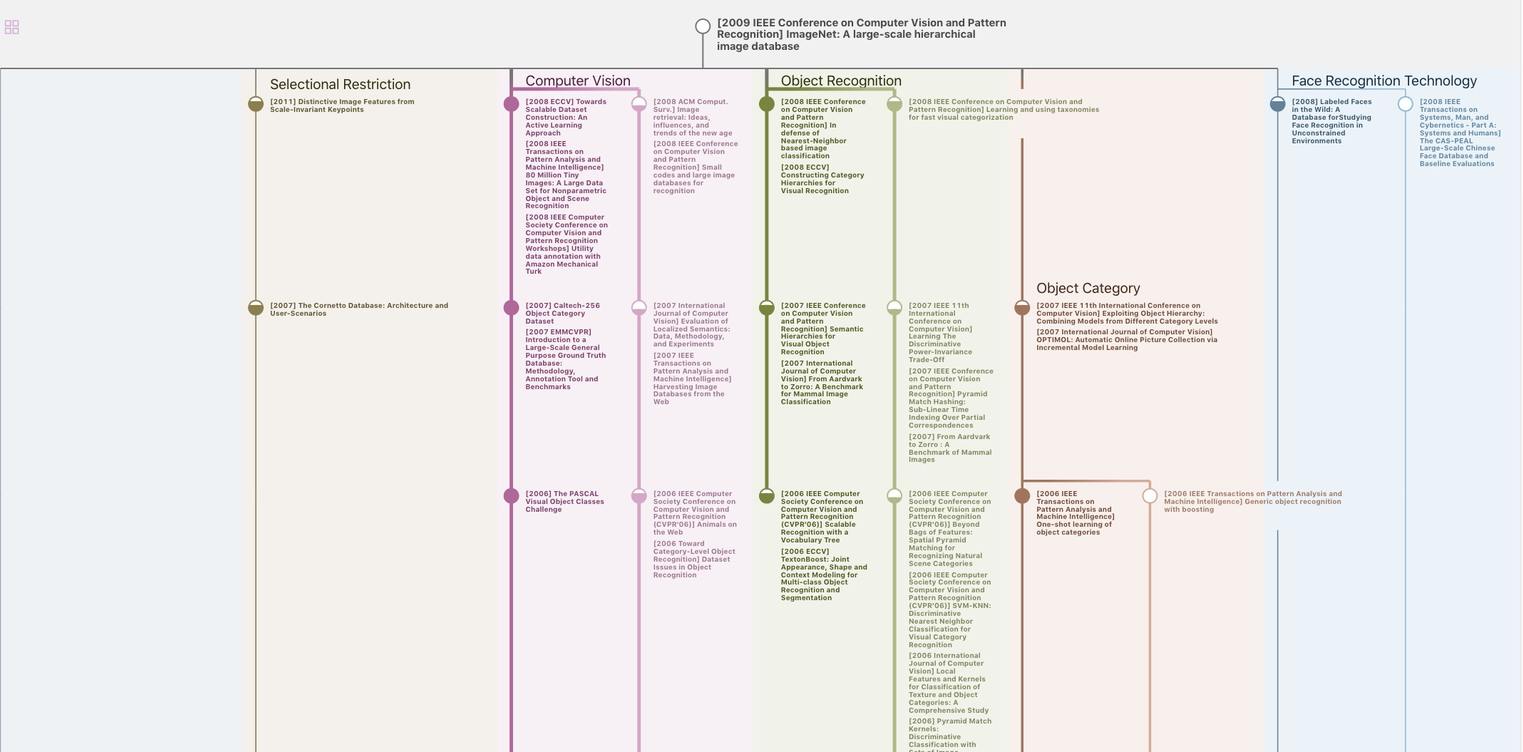
生成溯源树,研究论文发展脉络
Chat Paper
正在生成论文摘要