Dual Gauss-Newton Directions for Deep Learning
CoRR(2023)
摘要
Inspired by Gauss-Newton-like methods, we study the benefit of leveraging the structure of deep learning objectives, namely, the composition of a convex loss function and of a nonlinear network, in order to derive better direction oracles than stochastic gradients, based on the idea of partial linearization. In a departure from previous works, we propose to compute such direction oracles via their dual formulation, leading to both computational benefits and new insights. We demonstrate that the resulting oracles define descent directions that can be used as a drop-in replacement for stochastic gradients, in existing optimization algorithms. We empirically study the advantage of using the dual formulation as well as the computational trade-offs involved in the computation of such oracles.
更多查看译文
关键词
deep learning,dual,gauss-newton
AI 理解论文
溯源树
样例
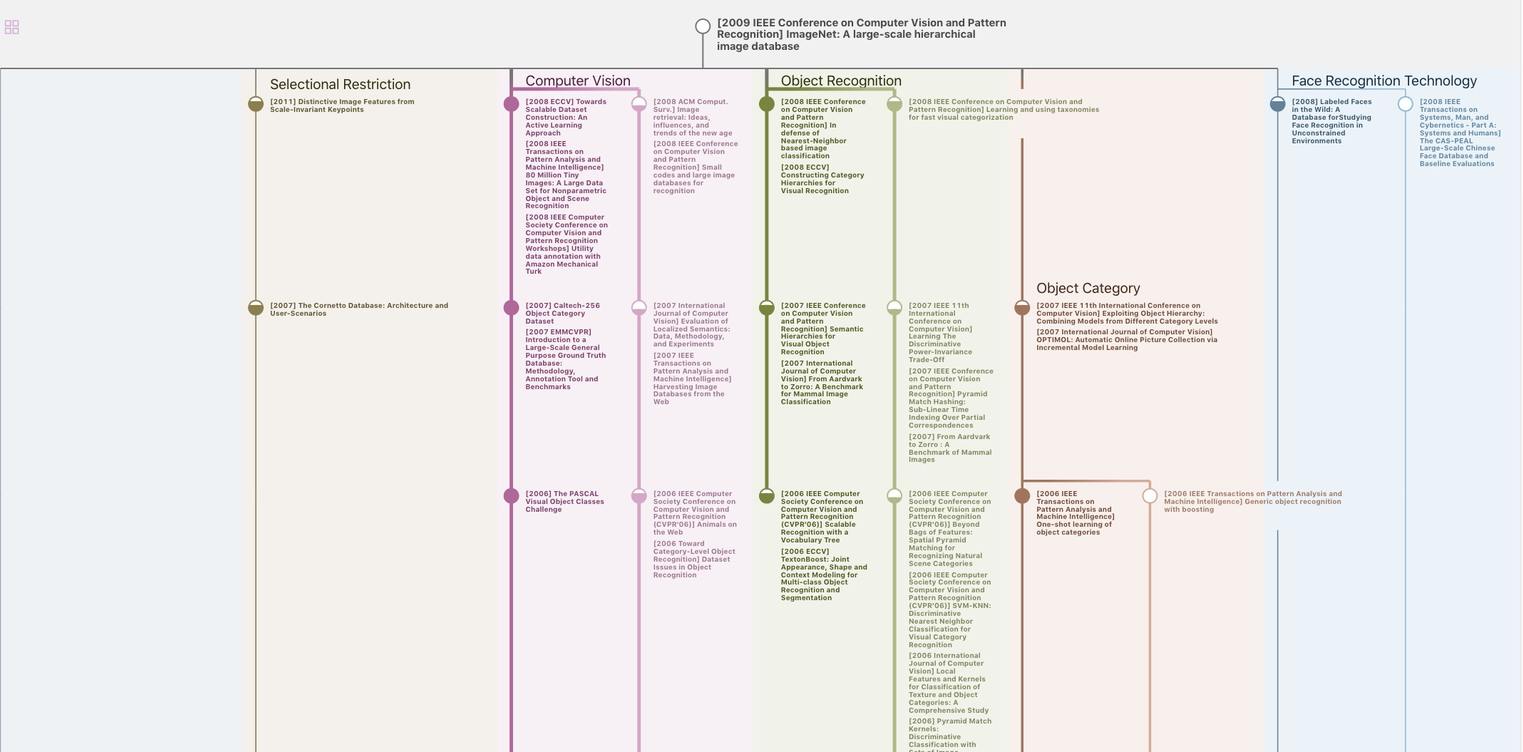
生成溯源树,研究论文发展脉络
Chat Paper
正在生成论文摘要