Scalable inference with Autoregressive Neural Ratio Estimation
arXiv (Cornell University)(2023)
摘要
In recent years, there has been a remarkable development of simulation-based inference (SBI) algorithms, and they have now been applied across a wide range of astrophysical and cosmological analyses. There are a number of key advantages to these methods, centred around the ability to perform scalable statistical inference without an explicit likelihood. In this work, we propose two technical building blocks to a specific sequential SBI algorithm, truncated marginal neural ratio estimation (TMNRE). In particular, first we develop autoregressive ratio estimation with the aim to robustly estimate correlated high-dimensional posteriors. Secondly, we propose a slice-based nested sampling algorithm to efficiently draw both posterior samples and constrained prior samples from ratio estimators, the latter being instrumental for sequential inference. To validate our implementation, we carry out inference tasks on three concrete examples: a toy model of a multi-dimensional Gaussian, the analysis of a stellar stream mock observation, and finally, a proof-of-concept application to substructure searches in strong gravitational lensing. In addition, we publicly release the code for both the autoregressive ratio estimator and the slice sampler.
更多查看译文
关键词
scalable inference,estimation,ratio
AI 理解论文
溯源树
样例
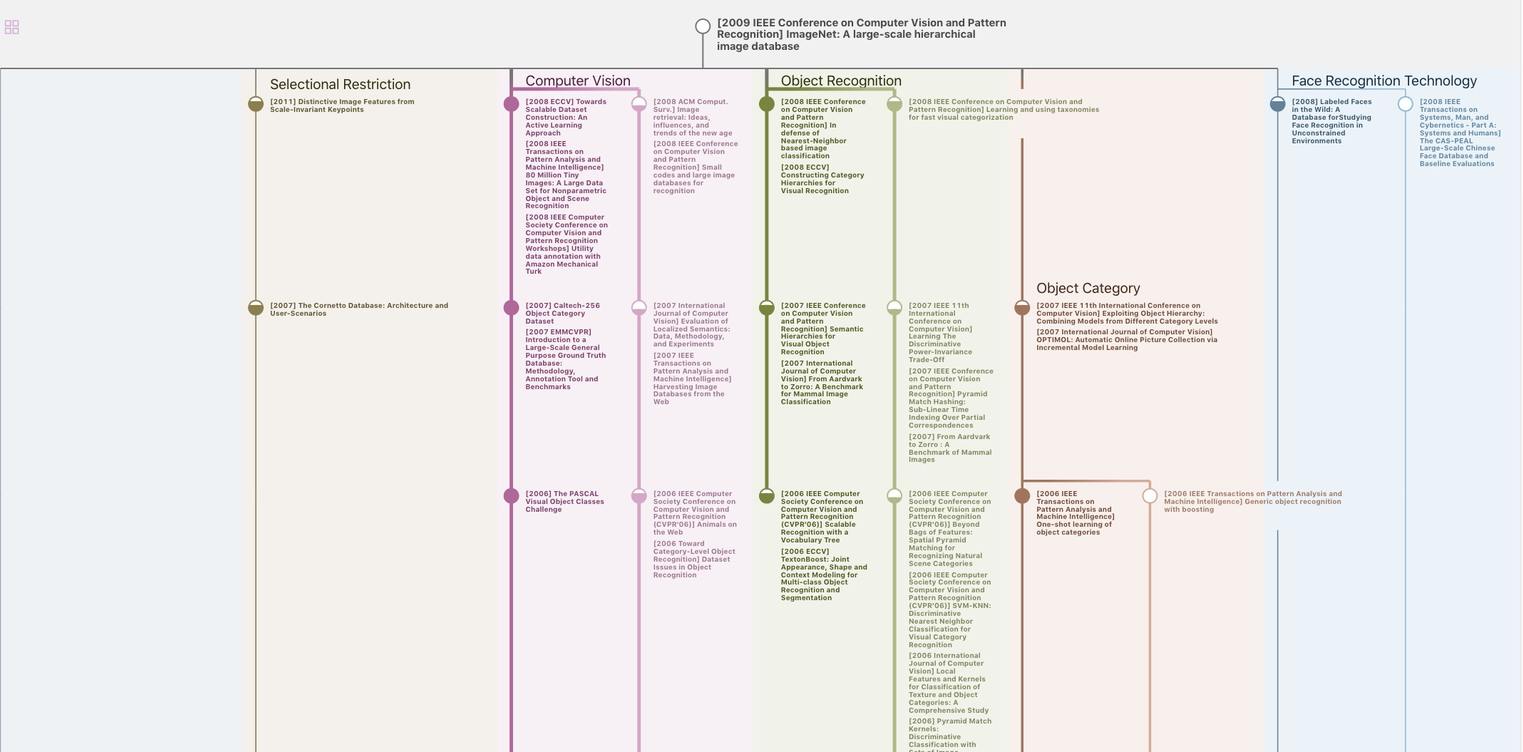
生成溯源树,研究论文发展脉络
Chat Paper
正在生成论文摘要