Designing Expert-Augmented Clinical Decision Support Systems to Predict Mortality Risk in ICUs
KUNSTLICHE INTELLIGENZ(2023)
摘要
One of the most critical infrastructures during the COVID-19 pandemic are intensive care units (ICU). ICU’s crucial task is to preserve the lives of patients and mitigate the pandemic’s impact on the population. However, most ICUs plan only one day ahead. This short-term planning becomes an obstacle during disaster situations since physicians need to decide efficiently and ensure the timely treatment of high-risk patients. Integrating machine learning (ML) systems for clinical decision support could improve this process by predicting the mortality risk of critically ill patients. Several ML approaches tackling this problem have already shown promising results. However, these systems mostly neglect the integration of explicit domain knowledge, which is crucial to ensure prediction quality and adaptability. Otherwise, black-box systems might base their decision on confounding variables and improper relationships. Following design science research, we utilize a unique dataset of patients diagnosed with SARS-CoV-2 in ICU care to design a clinical decision support system by combining ML and expert knowledge in the form of a severity score. We show that by augmenting the system with expert knowledge, its overall performance improves compared to the baseline approach.
更多查看译文
关键词
Expert knowledge,Machine learning,Clinical decision support system,Design science
AI 理解论文
溯源树
样例
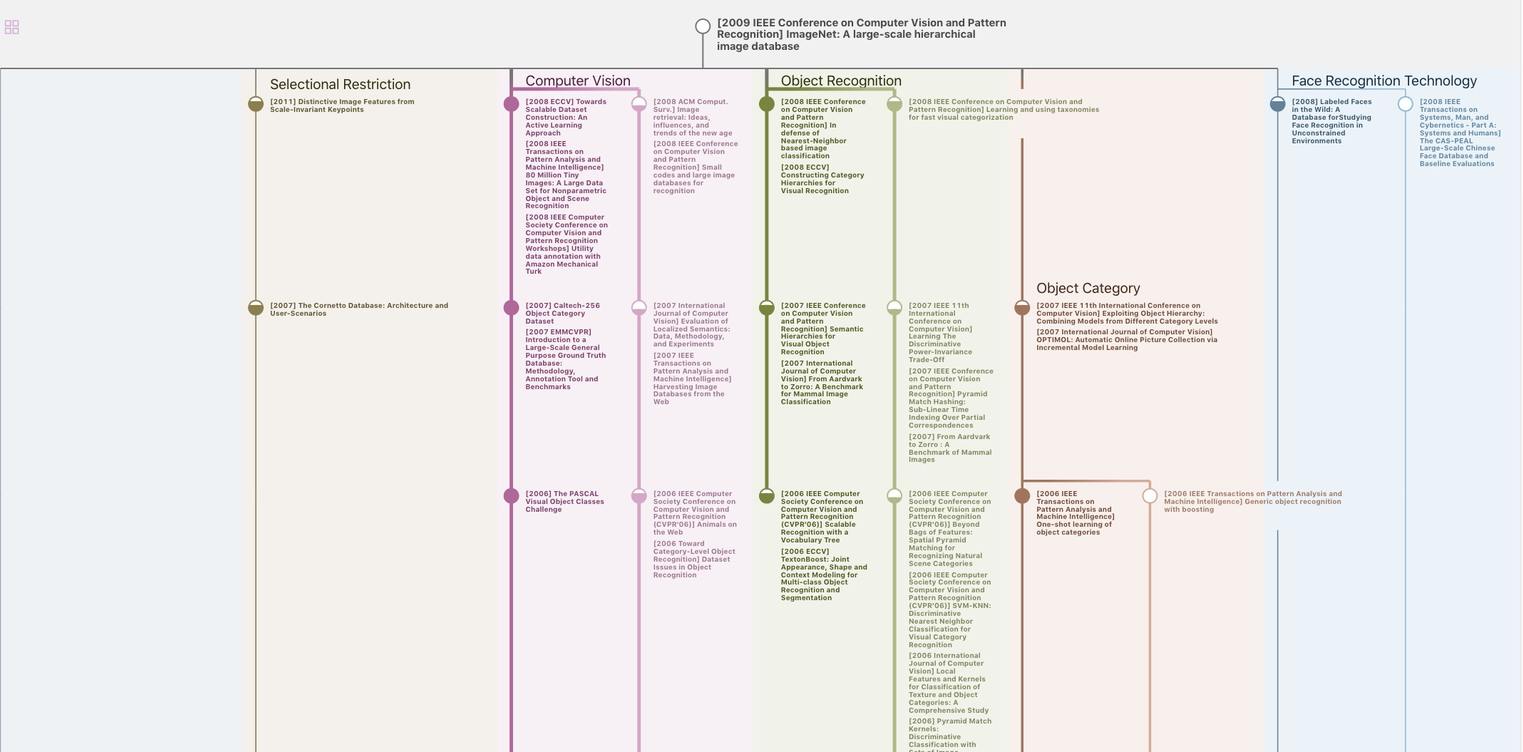
生成溯源树,研究论文发展脉络
Chat Paper
正在生成论文摘要