Modeling urban scale human mobility through big data analysis and machine learning
BUILDING SIMULATION(2024)
摘要
In the United States, the buildings sector consumes about 76% of electricity use and 40% of all primary energy use and associated greenhouse gas emissions. Occupant behavior has drawn increasing research interests due to its impacts on the building energy consumption. However, occupant behavior study at urban scale remains a challenge, and very limited studies have been conducted. As an effort to couple big data analysis with human mobility modeling, this study has explored urban scale human mobility utilizing three months Global Positioning System (GPS) data of 93,000 users at Phoenix Metropolitan Area. This research extracted stay points from raw data, and identified users’ home, work, and other locations by Density-Based Spatial Clustering algorithm. Then, daily mobility patterns were constructed using different types of locations. We propose a novel approach to predict urban scale daily human mobility patterns with 12-hour prediction horizon, using Long Short-Term Memory (LSTM) neural network model. Results shows the developed models achieved around 85% average accuracy and about 86% mean precision. The developed models can be further applied to analyze urban scale occupant behavior, building energy demand and flexibility, and contributed to urban planning.
更多查看译文
关键词
urban human mobility,big data analysis,urban scale occupant behavior,recurrent neural networks
AI 理解论文
溯源树
样例
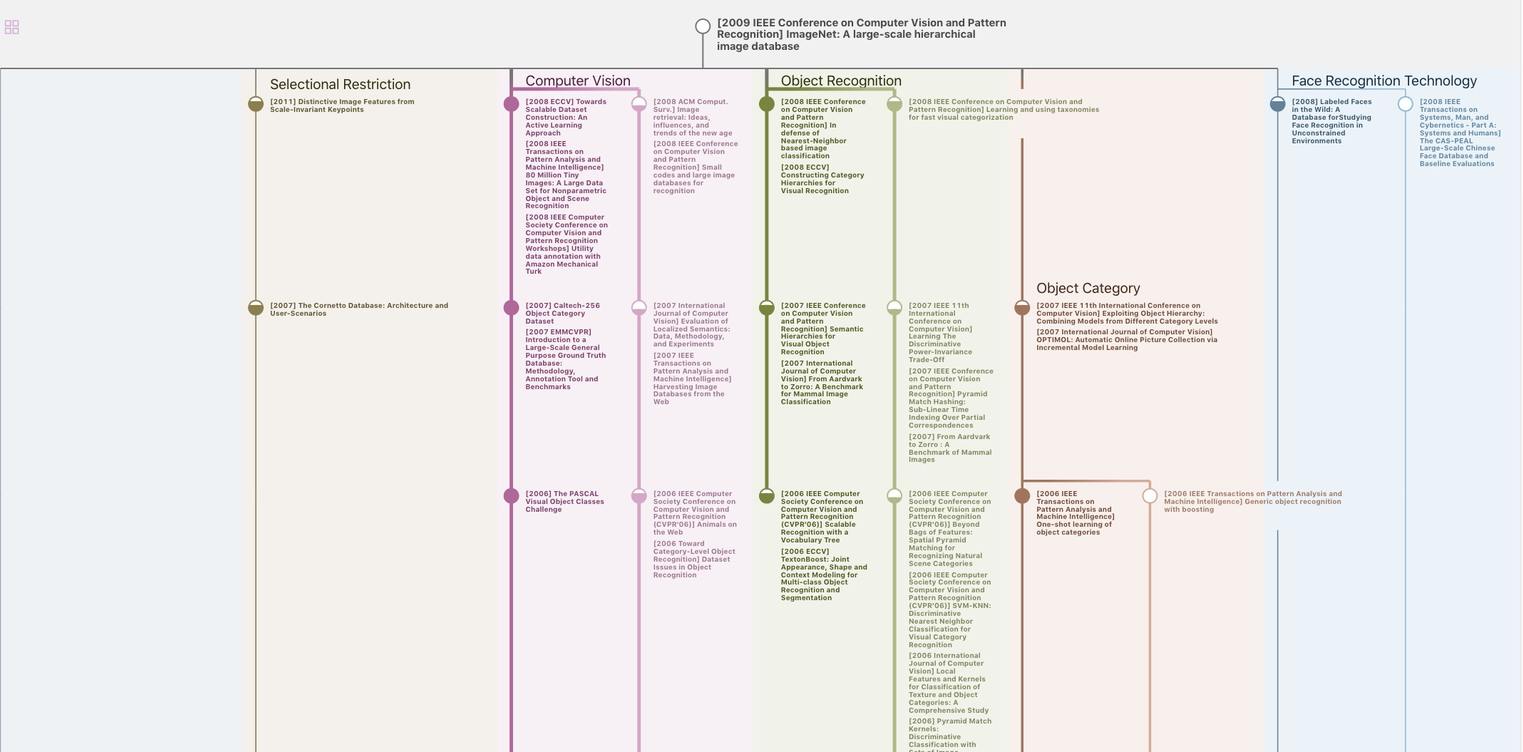
生成溯源树,研究论文发展脉络
Chat Paper
正在生成论文摘要