Correction: Wavelength and texture feature selection for hyperspectral imaging: a systematic literature review
Journal of Food Measurement and Characterization(2023)
摘要
Over the past two decades, hyperspectral imaging has become popular for non-destructive assessment of food quality, safety, and crop monitoring. Imaging delivers spatial information to complement the spectral information provided by spectroscopy. The key challenge with hyperspectral image data is the high dimensionality. Each image captures hundreds of wavelength bands. Reducing the number of wavelengths to an optimal subset is essential for speed and robustness due to the high multicollinearity between bands. However, there is yet to be a consensus on the best methods to find optimal subsets of wavelengths to predict attributes of samples. A systematic review procedure was developed and applied to review published research on hyperspectral imaging and wavelength selection. The review population included studies from all disciplines retrieved from the Scopus database that provided empirical results from hyperspectral images and applied wavelength selection. We found that 799 studies satisfied the defined inclusion criteria and investigated trends in their study design, wavelength selection, and machine learning techniques. For further analysis, we considered a subset of 71 studies published in English that incorporated spatial/texture features to understand how previous works combined spatial features with wavelength selection. This review ranks the wavelength selection techniques from each study to generate a table of the comparative performance of each selection method. Based on these findings, we suggest that future studies include spatial feature extraction methods to improve the predictive performance and compare them to a broader range of wavelength selection techniques, especially when proposing novel methods.
更多查看译文
关键词
Hyperspectral imaging,Multispectral imaging,Wavelength selection,Sensors,Spatial features,Food science,Computer vision,Agriculture
AI 理解论文
溯源树
样例
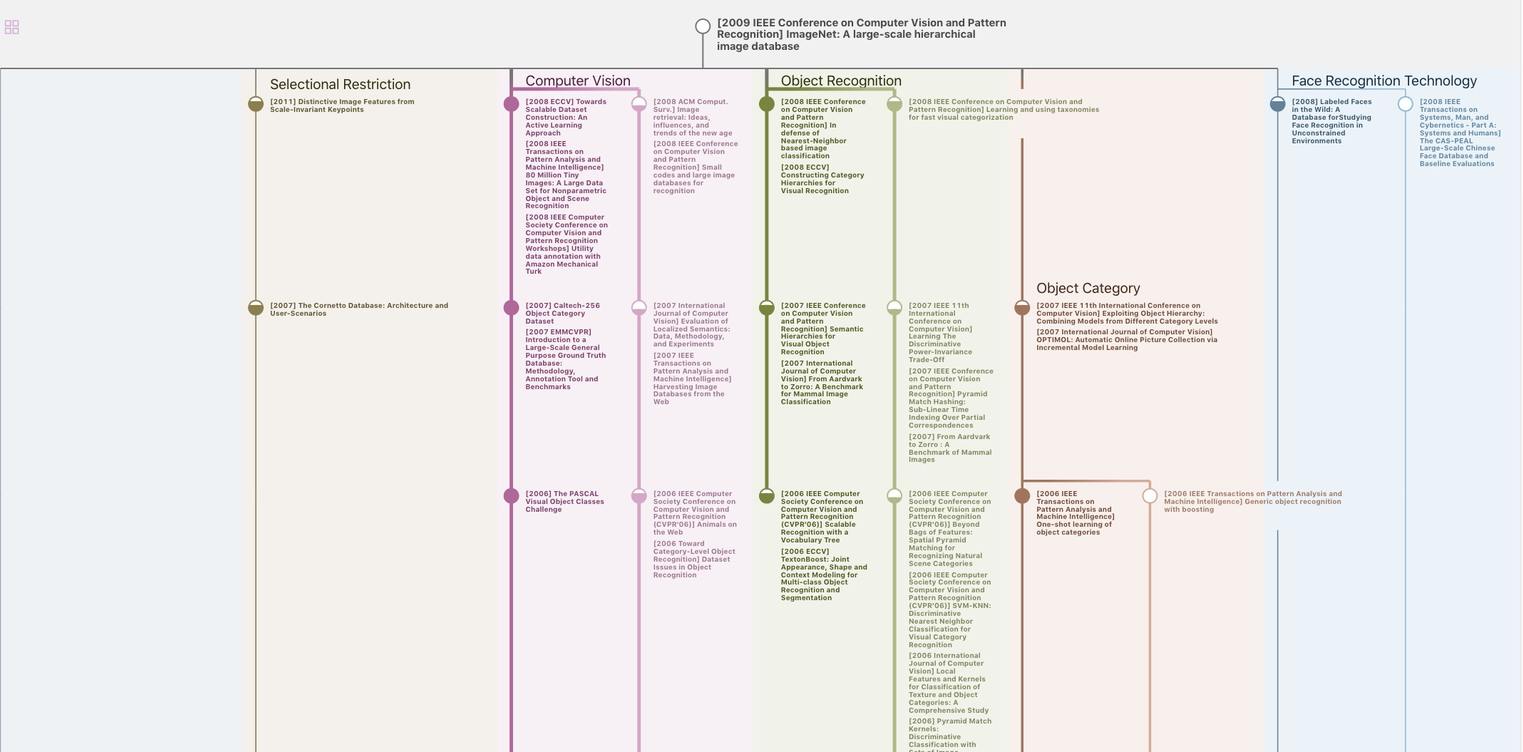
生成溯源树,研究论文发展脉络
Chat Paper
正在生成论文摘要