GTADT: Gated tone-sensitive acne grading via augmented domain transfer
Multimedia Tools and Applications(2024)
摘要
Automatic selfie facial acne grading plays a crucial role in treatment for facial care customers, and it attracts increasing attention with the revolution of telemedicine and virtual beauty. Unfortunately, the limited quantity and quality of the selfie facial dataset greatly challenge the learning of acne grading models. In this paper, we propose a G ated T one-sensitive A ugmented D omain T ransfer (GTADT) model to address the selfie facial acne grading problem. A “high-quality” clinical source domain and associated cross-domain data augmentation are introduced to generate sufficient data. Also, an aligned tone-sensitive model with multiple tone subnetworks is devised to bridge the domain gaps. In Addition, two gate networks are devised to capture the correlation between different tone subnetworks in both the label and feature spaces. We establish three selfie facial acne datasets which consist of people across different skin tones, ages, poses, etc . The experimental results on the newly established datasets demonstrate that: 1) both the cross-domain data augmentation, tone-sensitive module, and gate networks can enhance the performance; and 2) the proposed model performs favorably against state-of-the-art methods. We make both the code and datasets publicly available at https://github.com/WRLH/GTADT.git .
更多查看译文
关键词
Acne grading,Cross-domain augmentation,Domain transfer,Gate network,Skin tone
AI 理解论文
溯源树
样例
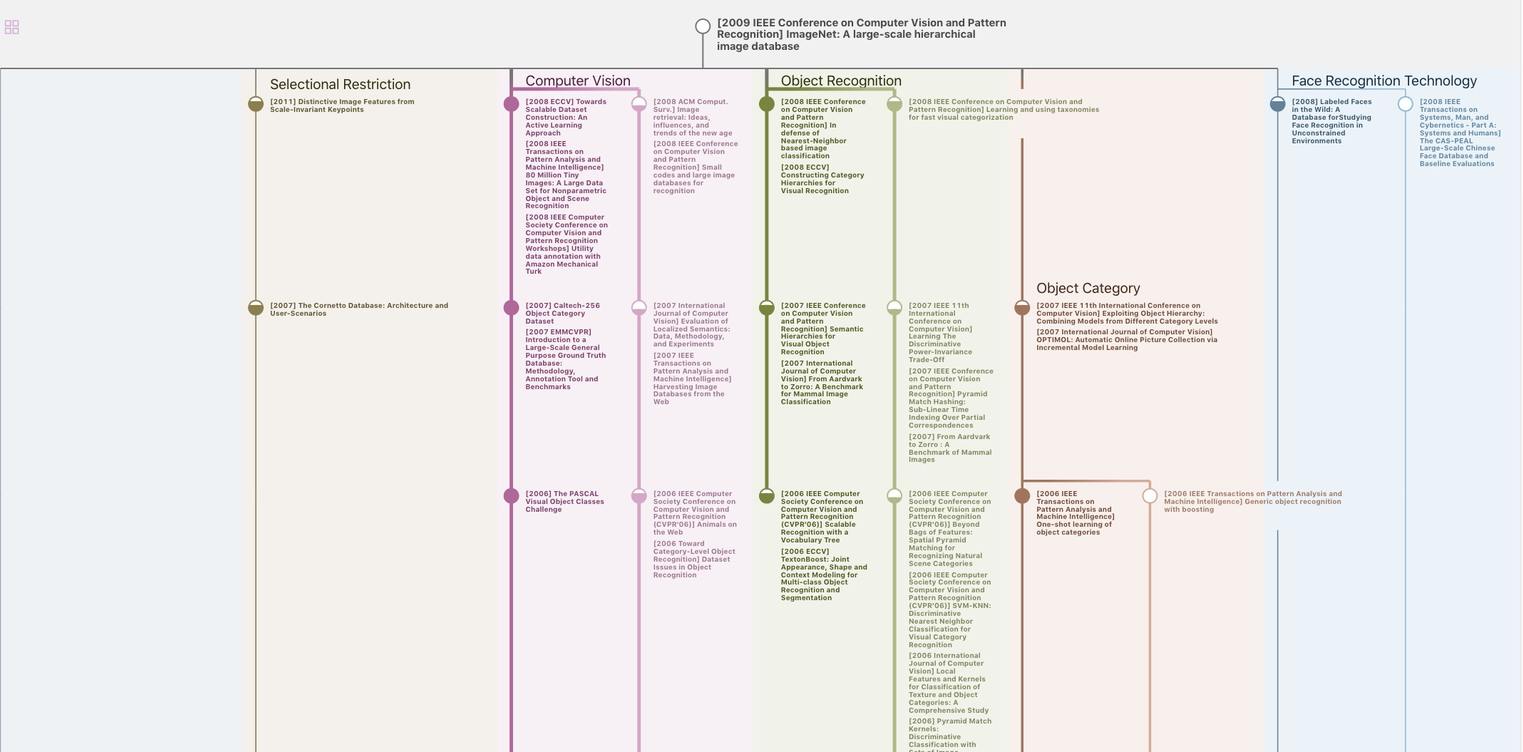
生成溯源树,研究论文发展脉络
Chat Paper
正在生成论文摘要