Shuffled ARSH-FATI: A Novel Meta-Heuristic for Lifetime Maximization of Range-Adjustable Wireless Sensor Networks
IEEE Transactions on Green Communications and Networking(2023)
摘要
Wireless Sensor Network (WSN) is an essential component of the Internet of Things (IoT), enabling the creation of a vast network of interconnected devices and systems that can interact with each other and with the physical world. WSNs are widely used for a variety of applications, including environmental monitoring, healthcare, industry 4.0, agriculture, transportation, and surveillance. WSN consists of devices with limited residual energy therefore, the lifetime improvement of WSN is critical for achieving the full potential of IoT and enabling the creation of more robust and reliable connected systems. This paper explores the problem of Lifetime Maximization of Range Adjustable Sensors (LM-RAS). The goal is to optimize the lifetime of the WSN consisting of range-adjustable sensors while satisfying two constraints. Firstly, all targets in the network must be monitored simultaneously. Secondly, the activation time of each sensor should be limited by its battery energy. A novel meta-heuristic called Shuffled ARSH-FATI has been proposed that embeds ARSH-FATI into a multi-population method. Shuffled ARSH-FATI solves the LM-RAS problem by splitting it into two sub-problem. The first sub-problem deals with the creation of energy-efficient coverage schemes and the second sub-problem deal with the schedule of these schemes. Shuffled ARSH-FATI utilizes a Linear Programming (LP) model to generate an optimal schedule for the coverage schemes but its performance greatly depends on the quality of the coverage schemes. Shuffled ARSH-FATI simultaneously explores several regions of the search space by partitioning the coverage schemes in population into several sub-populations. It evolves each sub-population (in this problem a sub-set of coverage schemes) independently using ARSH-FATI. It incorporates the shuffling process via Merge, Shuffle, and Partition (MSP) algorithm that merges the sub-populations, shuffles, and then partitions the merged population into new sub-populations. The shuffling process along with the strategy to fine-tune the coverage scheme into energy-efficient coverage schemes maintains population diversity throughout the optimization. The diverse high-quality coverage scheme improves the performance of the LP model.
更多查看译文
关键词
IoT,WSN,shuffled ARSH-FATI,LM-RAS,sensor activity scheduling,lifetime
AI 理解论文
溯源树
样例
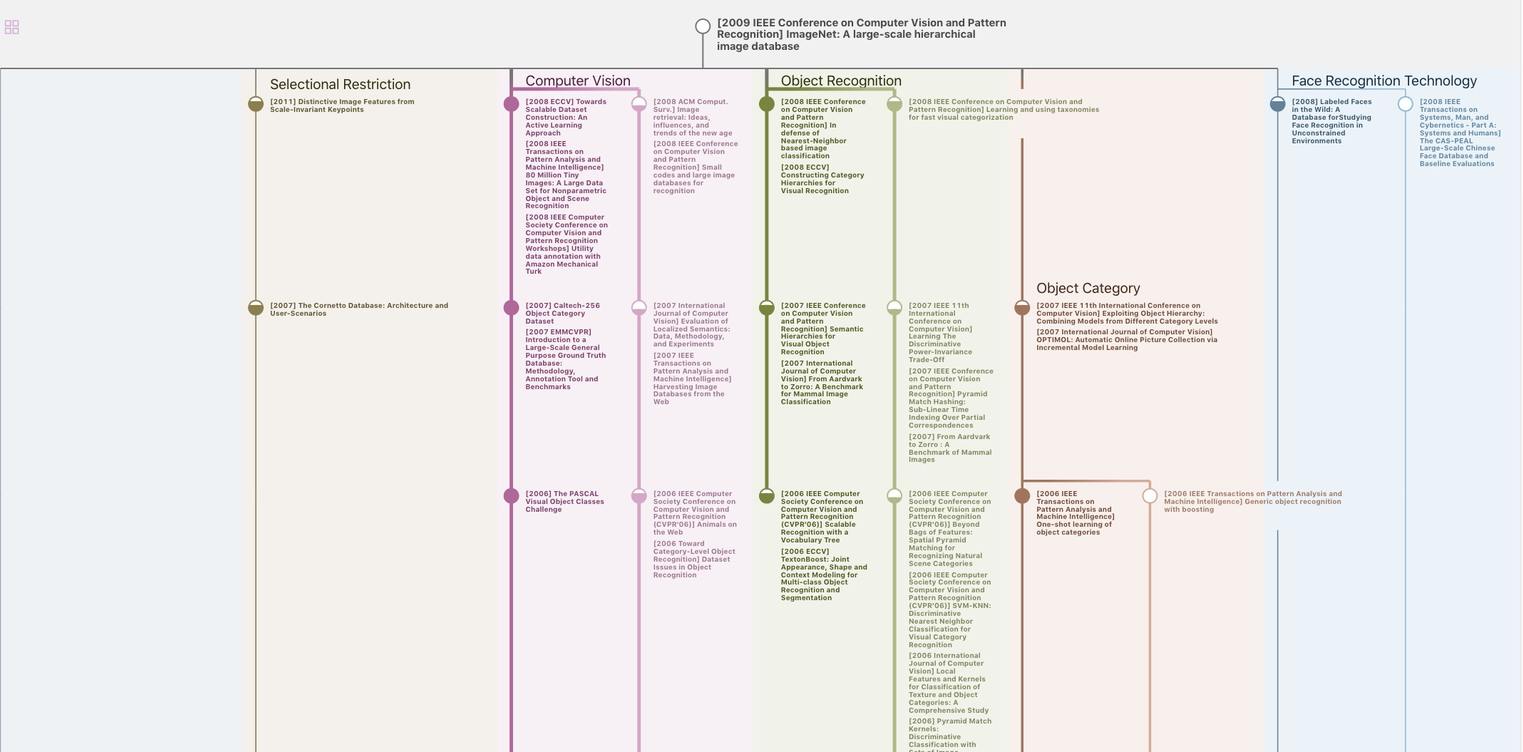
生成溯源树,研究论文发展脉络
Chat Paper
正在生成论文摘要