Nonlinear back-end optimization method for VSLAM with multi-convex combined maximum correntropy criterion
ISA TRANSACTIONS(2023)
摘要
Back-end optimization plays a key role in eliminating the accumulated error in Visual Simultaneous Localization And Mapping (VSLAM). Existing back-end optimization methods are usually premised on the Gaussian noise assumption which does not always hold true due to the non-convex nature of the image and the fact that non-Gaussian noises are often encountered in real scenes. In view of this, we propose a back-end optimization method based on Multi-Convex combined Maximum Correntropy Criterion (MCMCC). A MCMCC-based cost function is first tailored for nonlinear back-end optimization in the context of VSLAM and the optimization problem is solved through Levenberg-Marquardt algorithm iteratively. Then, the proposed method is applied to ORB-SLAM3 to test its performance on public indoor and outdoor datasets. The real time performance is also validated using a RaceBot platform in real indoor and outdoor environments. In addition, the reprojection error is statistically analyzed to demonstrate the non-Gaussian characteristics in the back-end optimization process. Finally, the suggestion parameters are also provided through experiments for further study.(c) 2023 ISA. Published by Elsevier Ltd. All rights reserved.
更多查看译文
关键词
Visual SLAM,Back-end optimization,Maximum correntropy criterion,Non-Gaussian noises
AI 理解论文
溯源树
样例
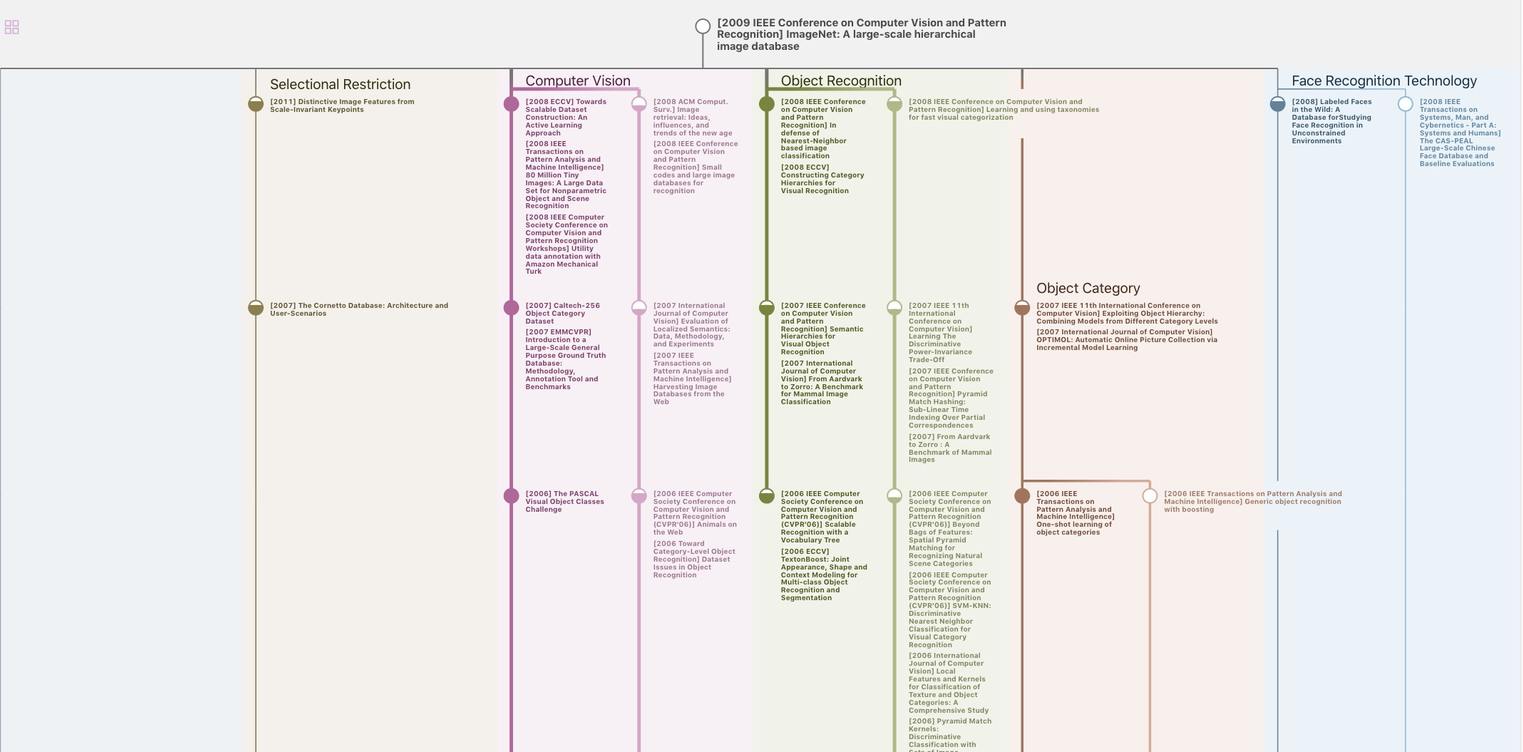
生成溯源树,研究论文发展脉络
Chat Paper
正在生成论文摘要