New User Intent Discovery With Robust Pseudo Label Training and Source Domain Joint Training
IEEE Intelligent Systems(2023)
摘要
Discovering new user intents based on existing intents from constantly incoming unlabeled data is an important task in many intelligent systems deployed in the real world (e.g., dialogue systems). Since data with new intents are completely unlabeled, most current approaches employ clustering methods to generate pseudo labels to train their models. However, due to intent gaps between existing and new intents, pseudo labels generated by these models are noisy, and prior knowledge from existing intents is not fully utilized. To mitigate these issues, we propose a robust pseudo label training and source domain joint-training network to refine the noisy pseudo labels and make full use of prior knowledge. Experimental results on three intent detection datasets show that our model is more effective and robust than state-of-the-art methods. The code and data are released at https://github.com/Lackel/PTJN.
更多查看译文
关键词
Feature extraction,Data models,Training,Noise measurement,Intelligent systems,Data mining,Knowledge engineering,Clustering methods,Labeling,User centered design,Recommender systems,Quality of service,Knowledge discovery
AI 理解论文
溯源树
样例
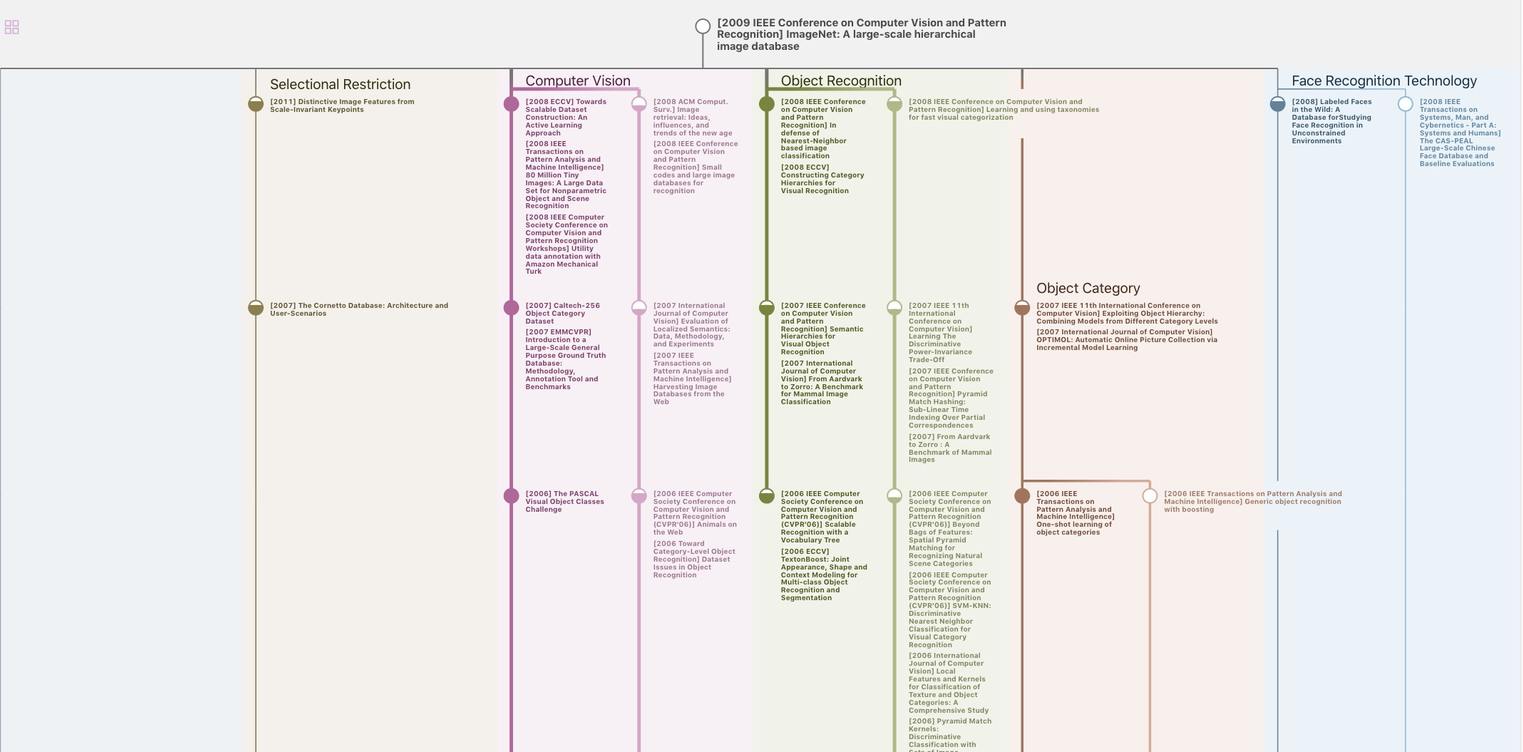
生成溯源树,研究论文发展脉络
Chat Paper
正在生成论文摘要