Deep learning reduces data requirements and allows real-time measurements in Imaging Fluorescence Correlation Spectroscopy
bioRxiv (Cold Spring Harbor Laboratory)(2023)
摘要
Imaging Fluorescence Correlation Spectroscopy (Imaging FCS) is a powerful tool to extract information on molecular mobilities, actions and interactions in live cells, tissues and organisms. Nevertheless, several limitations restrict its applicability. First, FCS is data hungry, requiring 50,000 frames at 1 ms time resolution to obtain accurate parameter estimates. Second, the data size makes evaluation slow. Thirdly, as FCS evaluation is model-dependent, data evaluation is significantly slowed unless analytic models are available. Here we introduce two convolutional neural networks (CNNs) – FCSNet and Im-FCSNet – for correlation and intensity trace analysis, respectively. FCSNet robustly predicts parameters in 2D and 3D live samples. ImFCSNet reduces the amount of data required for accurate parameter retrieval by at least one order of magnitude and makes correct estimates even in moderately defocused samples. Both CNNs are trained on simulated data, are model-agnostic, and allow autonomous, real-time evaluation of Imaging FCS measurements.
### Competing Interest Statement
The authors have declared no competing interest.
更多查看译文
关键词
fluorescence correlation,deep learning,spectroscopy,imaging,real-time
AI 理解论文
溯源树
样例
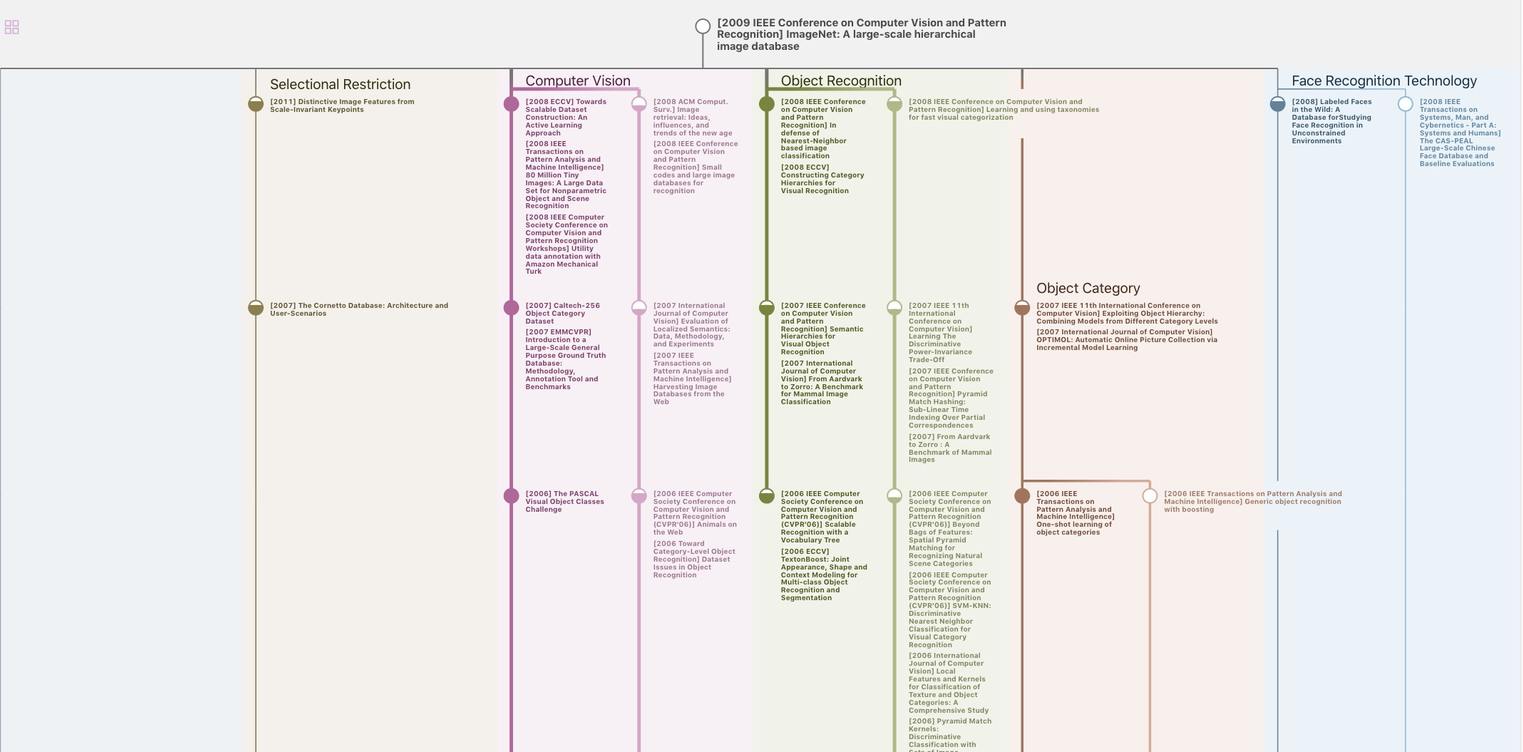
生成溯源树,研究论文发展脉络
Chat Paper
正在生成论文摘要