AnglesRefine: refinement of 3D protein structures using Transformer based on torsion angles
bioRxiv (Cold Spring Harbor Laboratory)(2023)
摘要
Motivation The goal of protein structure refinement is to enhance the precision of predicted protein models, particularly at the residue level of the local structure. Existing refinement approaches primarily rely on physics, whereas molecular simulation methods are resource-intensive and time-consuming. In this study, we employ deep learning methods to extract structural constraints from protein structure residues to assist in protein structure refinement. We introduce a novel method, AnglesRefine, which focuses on a protein’s secondary structure and employs a transformer model to refine various protein structure angles (psi, phi, omega, CA\_C\_N\_angle, C\_N\_CA\_angle, N\_CA\_C_angle), ultimately generating a superior protein model based on the refined angles.
Results We evaluate our approach against other cutting-edge protein structure refinement methods using the CASP11-14 and CASP15 datasets. Experimental outcomes indicate that our method generally surpasses other techniques on the CASP11-14 test dataset, while performing comparably or marginally better on the CASP15 test dataset. Our method consistently demonstrates the least likelihood of model quality degradation, e.g., the degradation percentage of our method is less than 10%, while other methods are about 50%. Furthermore, as our approach eliminates the need for conformational search and sampling, it significantly reduces computational time compared to existing protein structure refinement methods.
Availability
Contact caora{at}plu.edu
CCS CONCEPTS *Computing methodologies → Machine learning .
ACM Reference Format Lei Zhang, Junyong Zhu, Sheng Wang, Jie Hou, Dong Si, and Renzhi Cao. 2023. AnglesRefine: refinement of 3D protein structures using Transformer based on torsion angles. In Proceedings of 22nd International Workshop on Data Mining in Bioinformatics (BIOKDD 2023) (BIOKDD ‘2023) . ACM, New York, NY, USA, 10 pages. https://doi.org/XXXXXXX.XXXXXXX
### Competing Interest Statement
The authors have declared no competing interest.
更多查看译文
关键词
3d protein structures,transformer
AI 理解论文
溯源树
样例
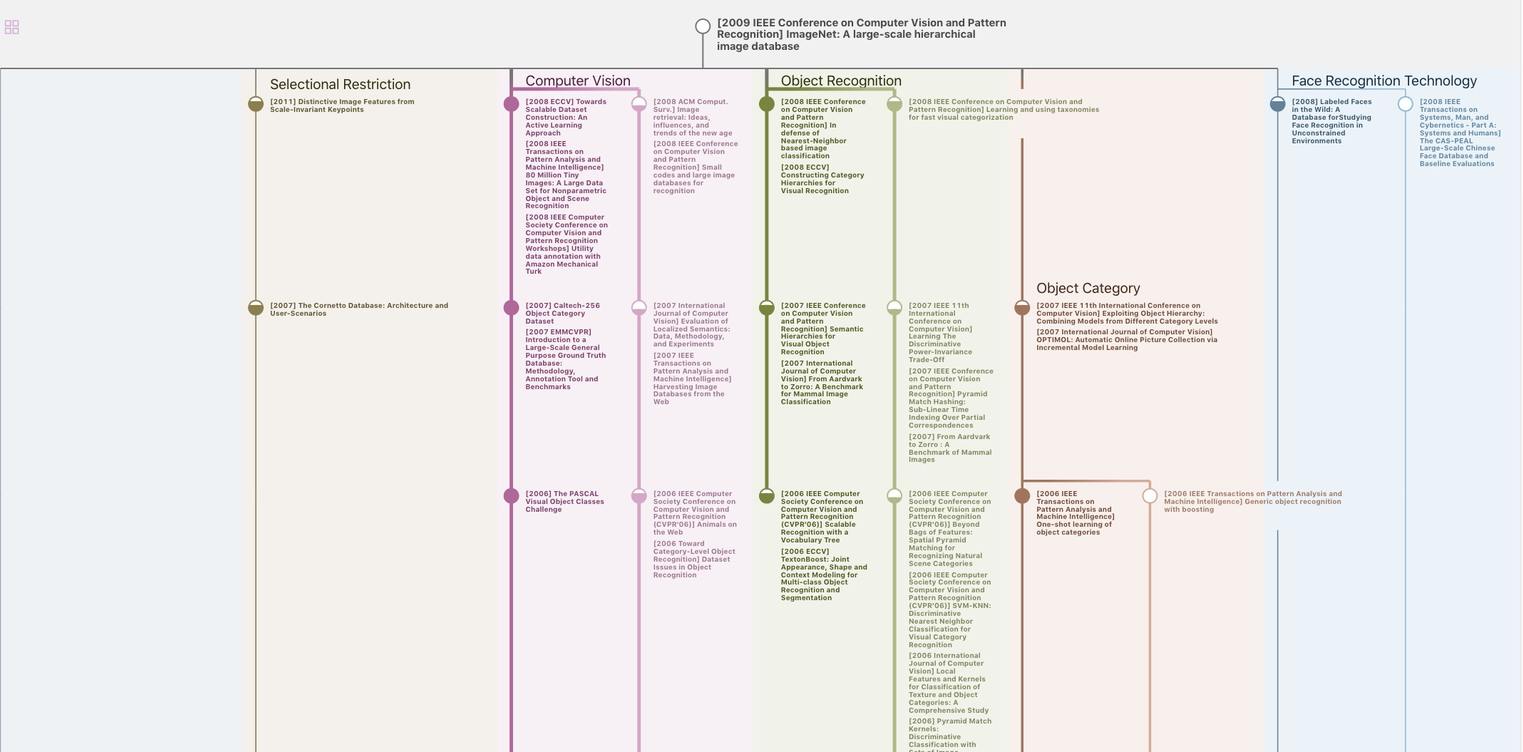
生成溯源树,研究论文发展脉络
Chat Paper
正在生成论文摘要