Fragle: Universal ctDNA quantification using deep learning of fragmentomic profiles
biorxiv(2024)
摘要
Quantification of circulating tumor DNA (ctDNA) levels in blood enables non-invasive surveillance of cancer progression. Fragle is an ultra-fast deep learning-based method for ctDNA quantification directly from cell-free DNA fragment length profiles. We developed Fragle using low-pass whole genome sequence (lpWGS) data from 8 cancer types and healthy control cohorts, demonstrating high accuracy, and improved lower limit of detection in independent cohorts as compared to existing tumor-naive methods. Uniquely, Fragle is also compatible with targeted sequencing data, exhibiting high accuracy across both research and commercial targeted gene panels. We used this method to study longitudinal plasma samples from colorectal cancer patients, identifying strong concordance of ctDNA dynamics and treatment response. Furthermore, prediction of minimal residual disease in resected lung cancer patients exhibited significant risk stratification beyond a tumor-naive targeted panel. Overall, Fragle is a versatile, fast, and accurate method for ctDNA quantification with potential for broad clinical utility.
### Competing Interest Statement
The authors have declared no competing interest.
更多查看译文
AI 理解论文
溯源树
样例
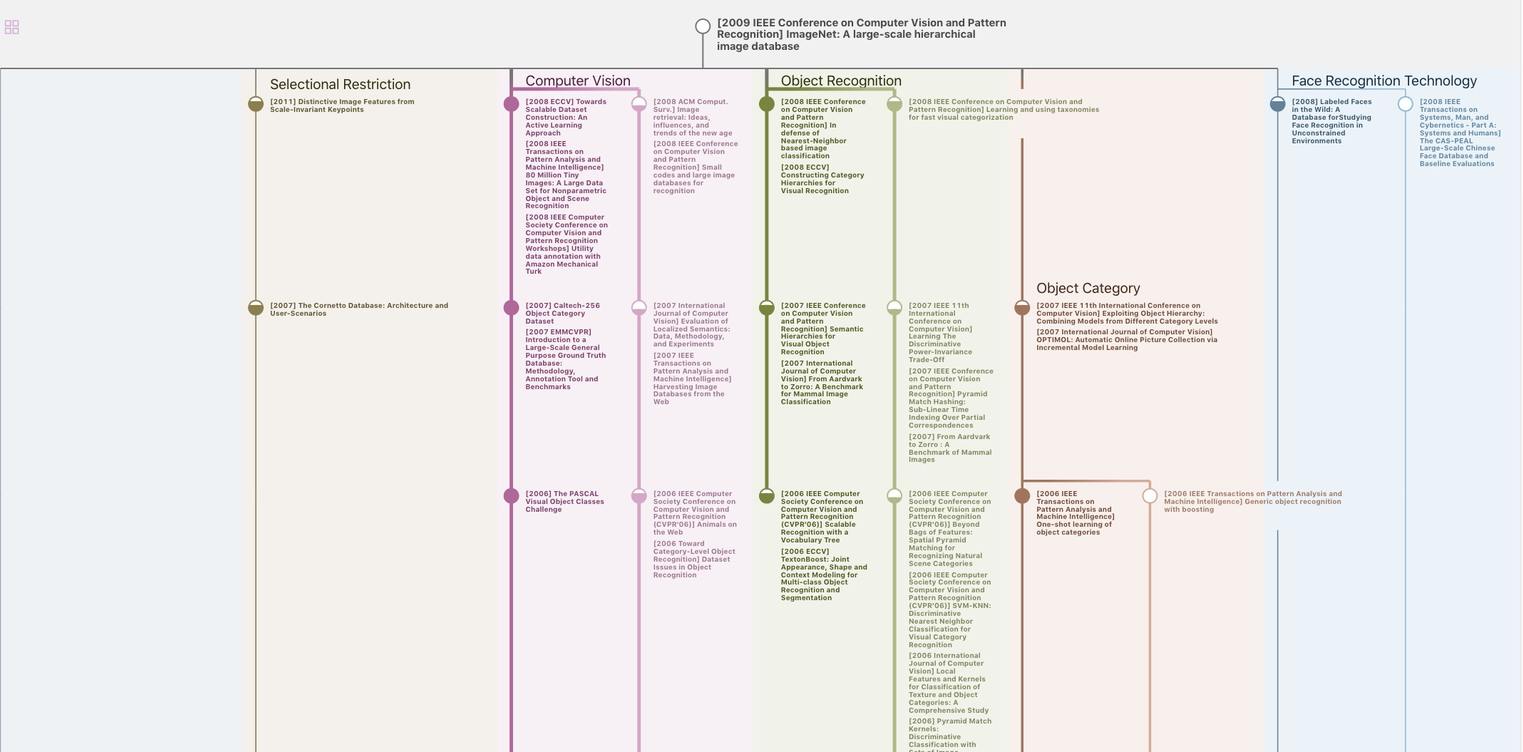
生成溯源树,研究论文发展脉络
Chat Paper
正在生成论文摘要