Attractor neural networks with double well synapses
PLOS COMPUTATIONAL BIOLOGY(2024)
摘要
It is widely believed that memory storage depends on activity-dependent synaptic modifications. Classical studies of learning and memory in neural networks describe synaptic efficacy either as continuous or discrete. However, recent results suggest an intermediate scenario in which synaptic efficacy can be described by a continuous variable, but whose distribution is peaked around a small set of discrete values. Motivated by these results, we explored a model in which each synapse is described by a continuous variable that evolves in a potential with multiple minima. External inputs to the network can switch synapses from one potential well to another. Our analytical and numerical results show that this model can interpolate between models with discrete synapses which correspond to the deep potential limit, and models in which synapses evolve in a single quadratic potential. We find that the storage capacity of the network with double well synapses exhibits a power law dependence on the network size, rather than the logarithmic dependence observed in models with single well synapses. In addition, synapses with deeper potential wells lead to more robust information storage in the presence of noise. When memories are sparsely encoded, the scaling of the capacity with network size is similar to previously studied network models in the sparse coding limit. A long-lasting question in neuroscience is whether synaptic efficacies should be described as continuous variable or discrete variables. Recent experiments indicate that it is a combination of both: synaptic efficacy changes continuously, but its distribution peaks at several discrete values. In this study, we introduce a synapse model described by a double well potential, and investigate the memory properties of networks of neurons connected with such synapses. Our results show in networks with a bimodal weight distribution, the storage capacity depends on network size as a power law. In addition, we demonstrate that networks with such synapses store information more robustly in the presence of noise, compared to networks with synapses with a single well potential.
更多查看译文
AI 理解论文
溯源树
样例
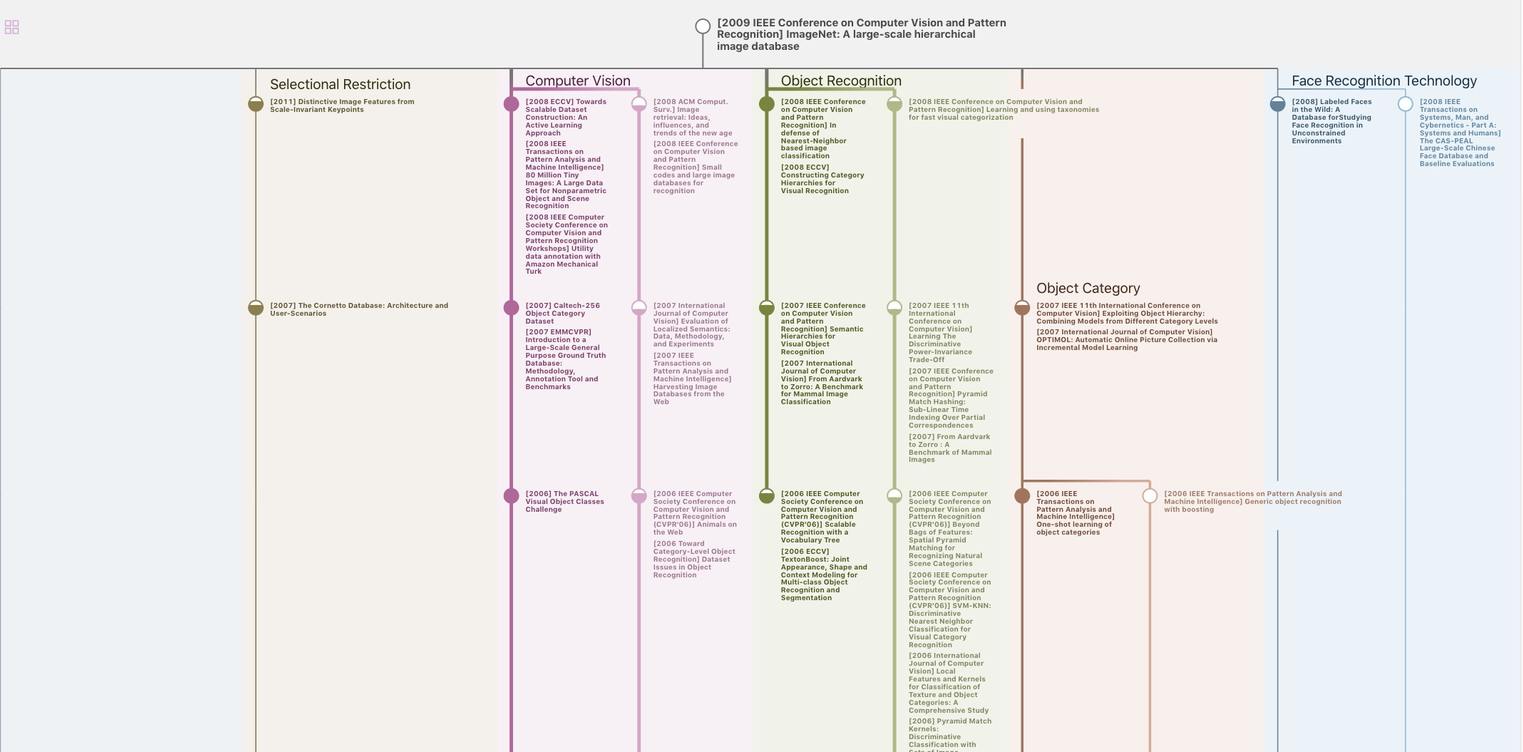
生成溯源树,研究论文发展脉络
Chat Paper
正在生成论文摘要