Energformer: A New Transformer Model for Energy Disaggregation
IEEE Transactions on Consumer Electronics(2023)
摘要
In recent years, a lot of progress has been reported in the field of energy disaggregation, also referred to as Non-Intrusive Load Monitoring (NILM). Despite the fact that there are many studies focusing on the residential sector, there is considerably less research interest for the industrial sector. In this paper, we present a deep neural network based on Transformers, targeted towards capturing complex patterns in long sequences of data. The proposed transformer architecture employs 1D spatial convolutions in self-attention, and modifications inside the attention computations manage to reduce computational complexity without any loss in predictive accuracy. In order to evaluate the performance of the proposed deep learning architecture, a set of experiments has been conducted using a publicly available dataset. The experimental results indicate that the proposed model achieves better disaggregation accuracy compared to other state-of-the-art NILM models.
更多查看译文
关键词
Non-intrusive load monitoring,transformer,deep learning,neural networks
AI 理解论文
溯源树
样例
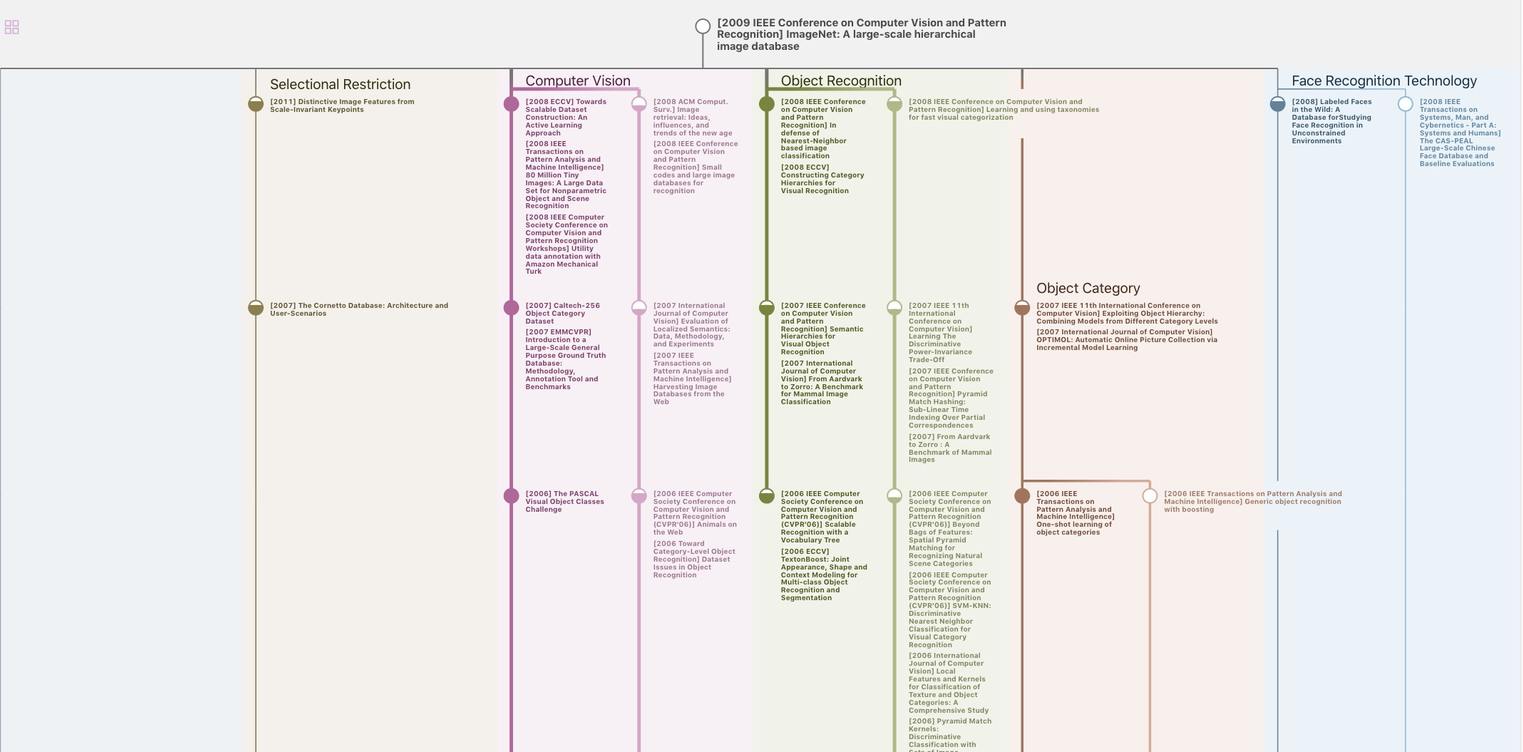
生成溯源树,研究论文发展脉络
Chat Paper
正在生成论文摘要