Syn_SegNet: A Joint Deep Neural Network for Ultrahigh-Field 7T MRI Synthesis and Hippocampal Subfield Segmentation in Routine 3T MRI
IEEE journal of biomedical and health informatics(2023)
摘要
Precise delineation of hippocampus subfields is crucial for the identification and management of various neurological and psychiatric disorders. However, segmenting these subfields automatically in routine 3T MRI is challenging due to their complex morphology and small size, as well as the limited signal contrast and resolution of the 3T images. This research proposes Syn_SegNet, an end-to-end, multitask joint deep neural network that leverages ultrahigh-field 7T MRI synthesis to improve hippocampal subfield segmentation in 3T MRI. Our approach involves two key components. First, we employ a modified Pix2PixGAN as the synthesis model, incorporating self-attention modules, image and feature matching loss, and ROI loss to generate high-quality 7T-like MRI around the hippocampal region. Second, we utilize a variant of 3D-U-Net with multiscale deep supervision as the segmentation subnetwork, incorporating an anatomic weighted cross-entropy loss that capitalizes on prior anatomical knowledge. We evaluate our method on hippocampal subfield segmentation in paired 3T MRI and 7T MRI with seven different anatomical structures. The experimental findings demonstrate that Syn_SegNet's segmentation performance benefits from integrating synthetic 7T data in an online manner and is superior to competing methods. Furthermore, we assess the generalizability of the proposed approach using a publicly accessible 3T MRI dataset. The developed method would be an efficient tool for segmenting hippocampal subfields in routine clinical 3T MRI.
更多查看译文
关键词
Generative adversarial network,hippocampal subfield segmentation,multiscale deep supervision,ultrahigh-field MRI synthesis
AI 理解论文
溯源树
样例
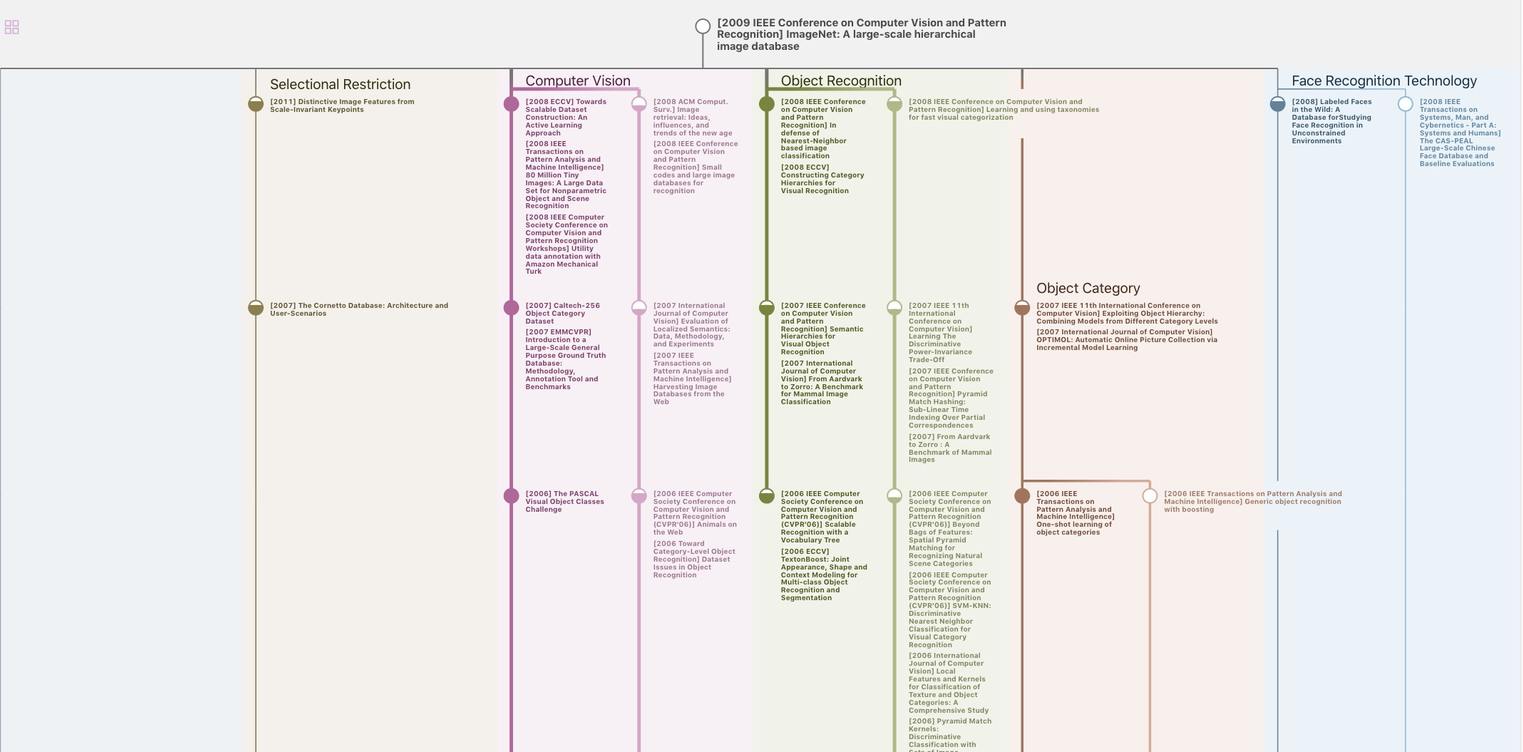
生成溯源树,研究论文发展脉络
Chat Paper
正在生成论文摘要