Hierarchical Uncertainty Estimation for Medical Image Segmentation Networks
CoRR(2023)
摘要
Learning a medical image segmentation model is an inherently ambiguous task, as uncertainties exist in both images (noise) and manual annotations (human errors and bias) used for model training. To build a trustworthy image segmentation model, it is important to not just evaluate its performance but also estimate the uncertainty of the model prediction. Most state-of-the-art image segmentation networks adopt a hierarchical encoder architecture, extracting image features at multiple resolution levels from fine to coarse. In this work, we leverage this hierarchical image representation and propose a simple yet effective method for estimating uncertainties at multiple levels. The multi-level uncertainties are modelled via the skip-connection module and then sampled to generate an uncertainty map for the predicted image segmentation. We demonstrate that a deep learning segmentation network such as U-net, when implemented with such hierarchical uncertainty estimation module, can achieve a high segmentation performance, while at the same time provide meaningful uncertainty maps that can be used for out-of-distribution detection.
更多查看译文
关键词
hierarchical uncertainty estimation,medical image segmentation
AI 理解论文
溯源树
样例
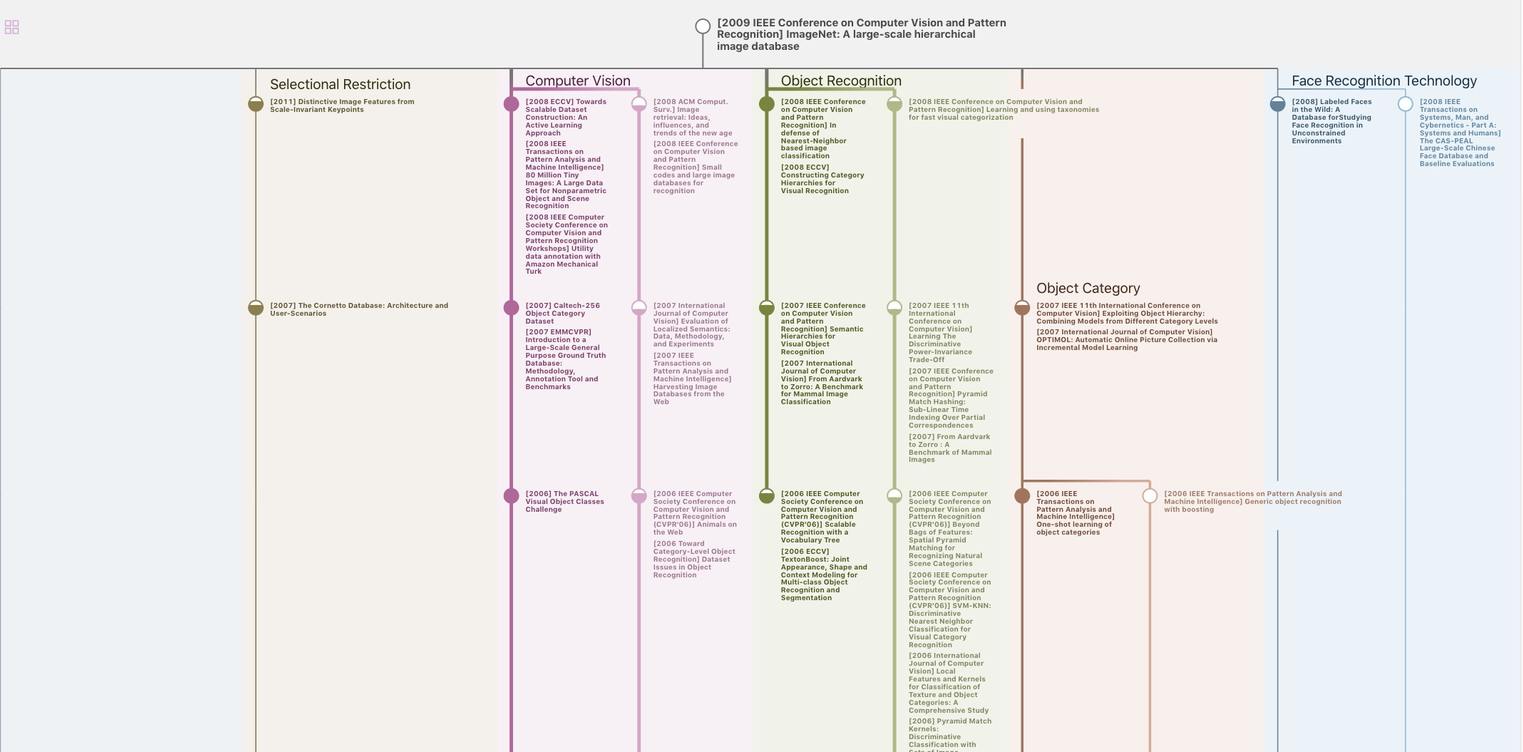
生成溯源树,研究论文发展脉络
Chat Paper
正在生成论文摘要