Enhancing Performance on Seen and Unseen Dialogue Scenarios using Retrieval-Augmented End-to-End Task-Oriented System
CoRR(2023)
摘要
End-to-end task-oriented dialogue (TOD) systems have achieved promising performance by leveraging sophisticated natural language understanding and natural language generation capabilities of pre-trained models. This work enables the TOD systems with more flexibility through a simple cache. The cache provides the flexibility to dynamically update the TOD systems and handle both existing and unseen dialogue scenarios. Towards this end, we first fine-tune a retrieval module to effectively retrieve the most relevant information entries from the cache. We then train end-to-end TOD models that can refer to and ground on both dialogue history and retrieved information during TOD generation. The cache is straightforward to construct, and the backbone models of TOD systems are compatible with existing pre-trained generative models. Extensive experiments demonstrate the superior performance of our framework, with a notable improvement in non-empty joint goal accuracy by 6.7% compared to strong baselines.
更多查看译文
关键词
unseen dialogue scenarios,retrieval-augmented,end-to-end,task-oriented
AI 理解论文
溯源树
样例
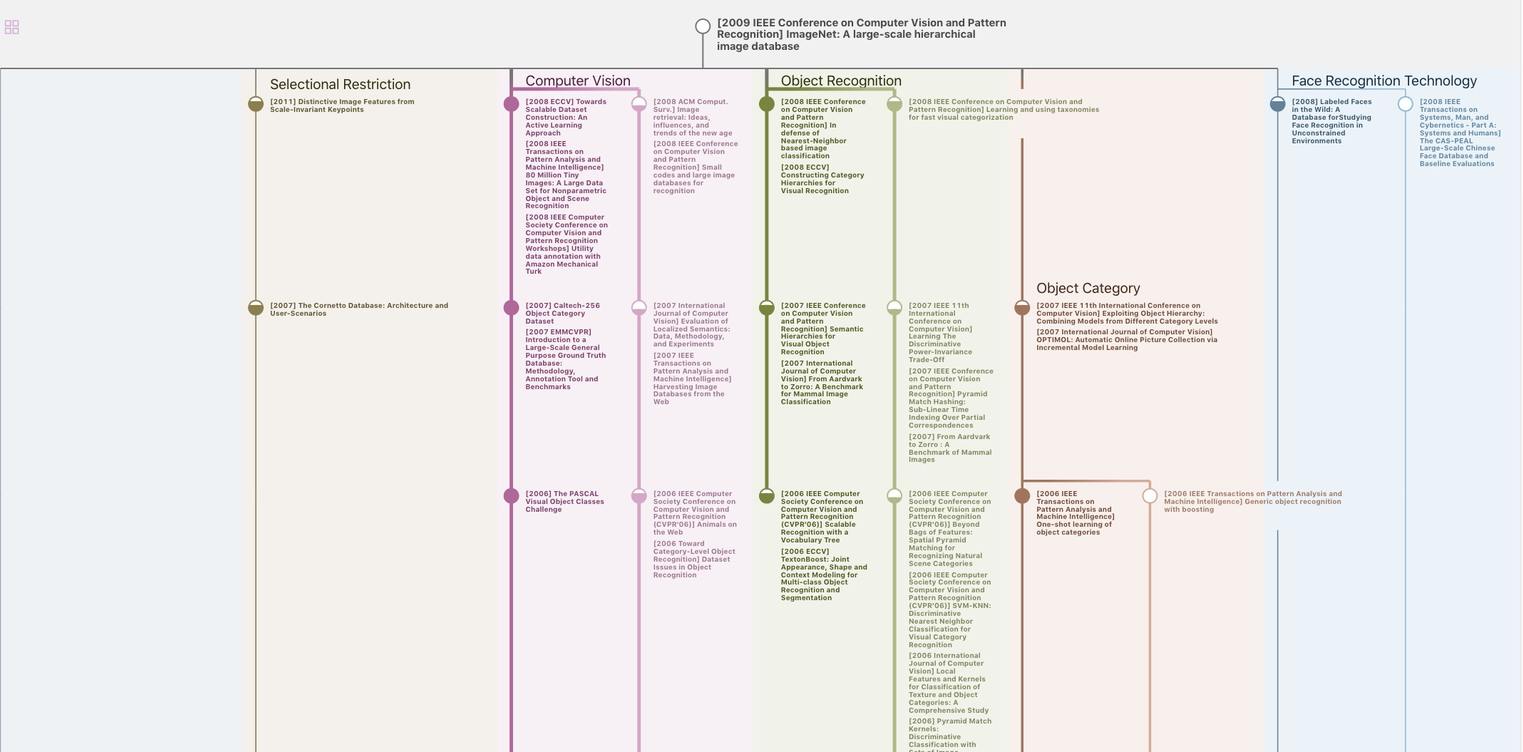
生成溯源树,研究论文发展脉络
Chat Paper
正在生成论文摘要