How to Mask in Error Correction Code Transformer: Systematic and Double Masking
CoRR(2023)
摘要
In communication and storage systems, error correction codes (ECCs) are pivotal in ensuring data reliability. As deep learning's applicability has broadened across diverse domains, there is a growing research focus on neural network-based decoders that outperform traditional decoding algorithms. Among these neural decoders, Error Correction Code Transformer (ECCT) has achieved the state-of-the-art performance, outperforming other methods by large margins. To further enhance the performance of ECCT, we propose two novel methods. First, leveraging the systematic encoding technique of ECCs, we introduce a new masking matrix for ECCT, aiming to improve the performance and reduce the computational complexity. Second, we propose a novel transformer architecture of ECCT called a double-masked ECCT. This architecture employs two different mask matrices in a parallel manner to learn more diverse features of the relationship between codeword bits in the masked self-attention blocks. Extensive simulation results show that the proposed double-masked ECCT outperforms the conventional ECCT, achieving the state-of-the-art decoding performance with significant margins.
更多查看译文
关键词
error correction code transformer
AI 理解论文
溯源树
样例
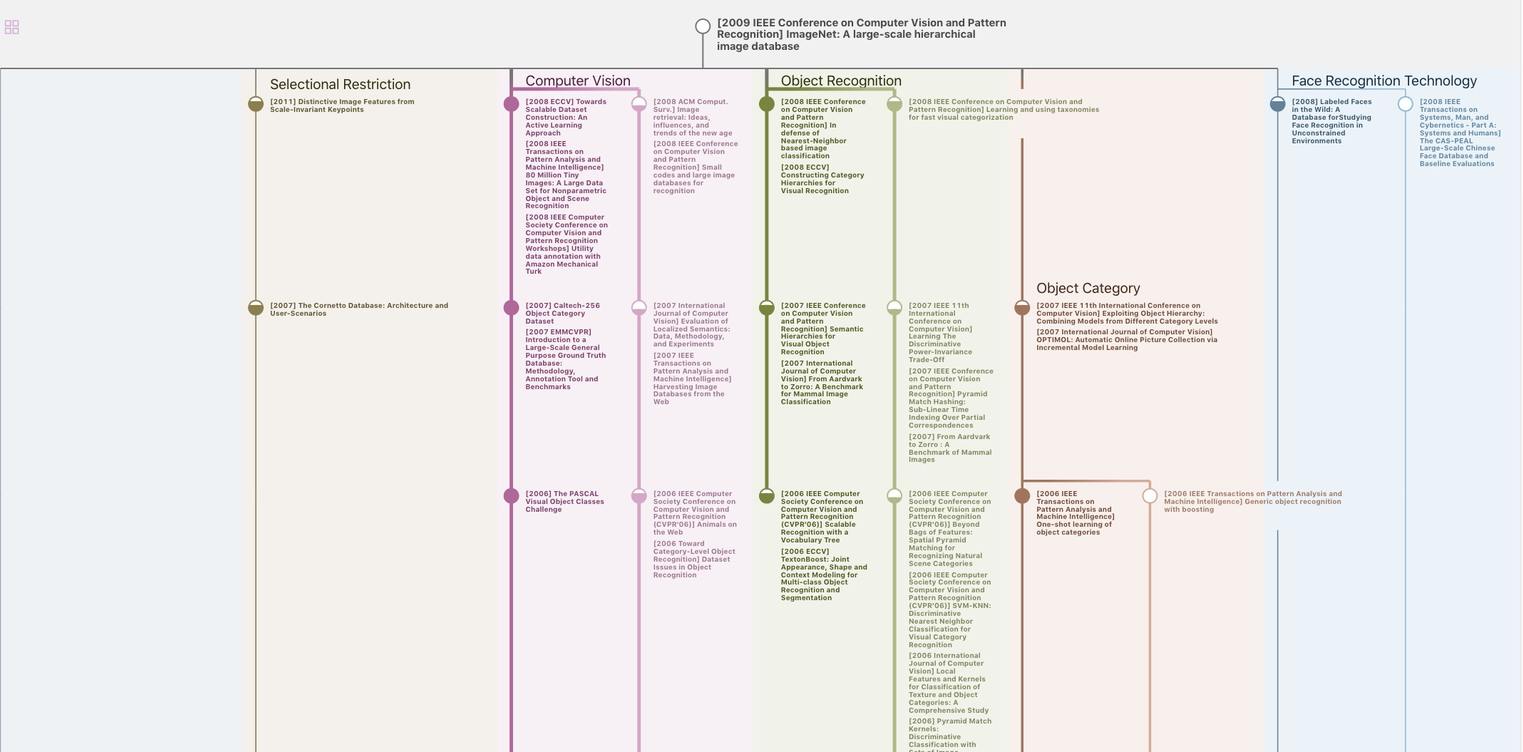
生成溯源树,研究论文发展脉络
Chat Paper
正在生成论文摘要