Max-affine regression via first-order methods
CoRR(2023)
摘要
We consider regression of a max-affine model that produces a piecewise linear model by combining affine models via the max function. The max-affine model ubiquitously arises in applications in signal processing and statistics including multiclass classification, auction problems, and convex regression. It also generalizes phase retrieval and learning rectifier linear unit activation functions. We present a non-asymptotic convergence analysis of gradient descent (GD) and mini-batch stochastic gradient descent (SGD) for max-affine regression when the model is observed at random locations following the sub-Gaussianity and an anti-concentration with additive sub-Gaussian noise. Under these assumptions, a suitably initialized GD and SGD converge linearly to a neighborhood of the ground truth specified by the corresponding error bound. We provide numerical results that corroborate the theoretical finding. Importantly, SGD not only converges faster in run time with fewer observations than alternating minimization and GD in the noiseless scenario but also outperforms them in low-sample scenarios with noise.
更多查看译文
关键词
regression,max-affine,first-order
AI 理解论文
溯源树
样例
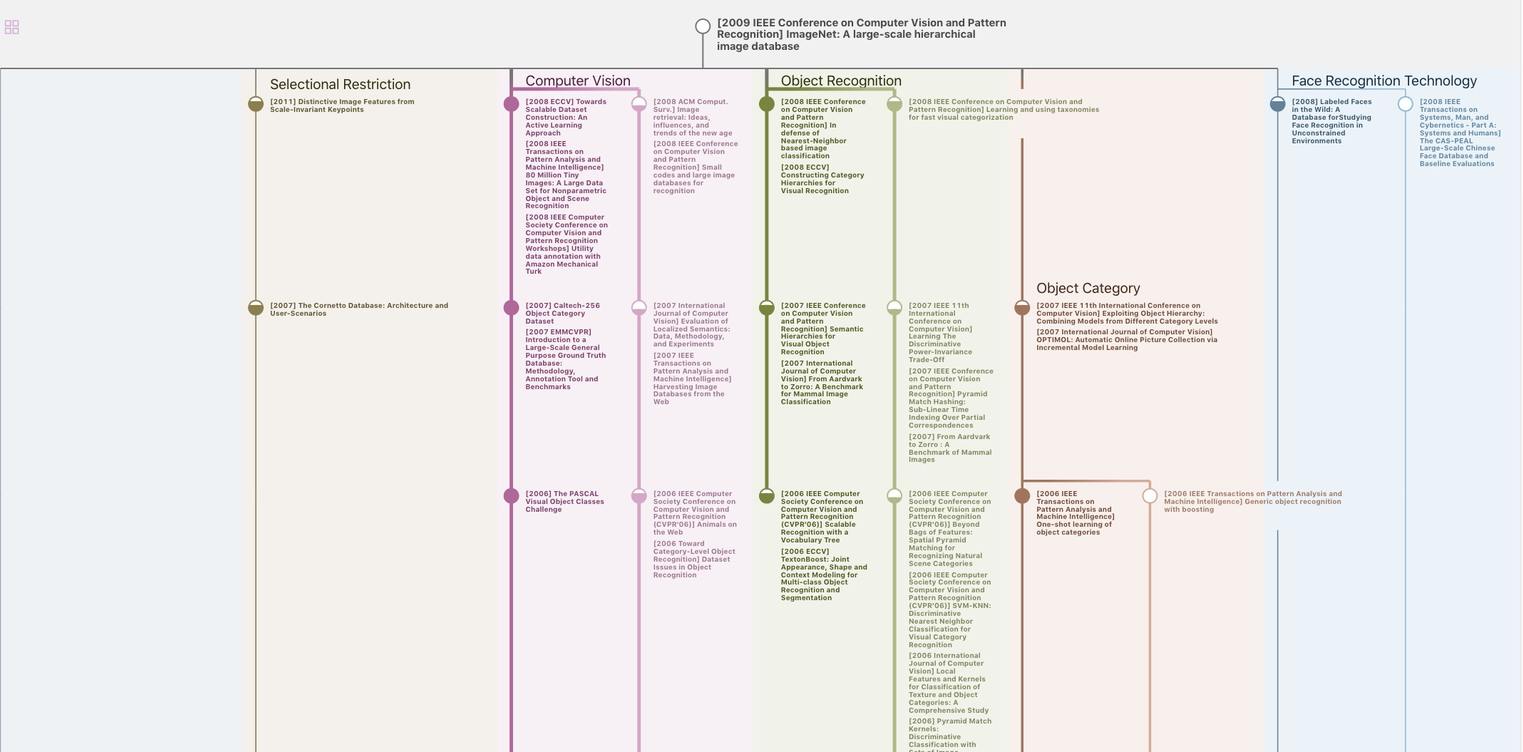
生成溯源树,研究论文发展脉络
Chat Paper
正在生成论文摘要