Active Inverse Learning in Stackelberg Trajectory Games
CoRR(2023)
摘要
Game-theoretic inverse learning is the problem of inferring the players' objectives from their actions. We formulate an inverse learning problem in a Stackelberg game between a leader and a follower, where each player's action is the trajectory of a dynamical system. We propose an active inverse learning method for the leader to infer which hypothesis among a finite set of candidates describes the follower's objective function. Instead of using passively observed trajectories like existing methods, the proposed method actively maximizes the differences in the follower's trajectories under different hypotheses to accelerate the leader's inference. We demonstrate the proposed method in a receding-horizon repeated trajectory game. Compared with uniformly random inputs, the leader inputs provided by the proposed method accelerate the convergence of the probability of different hypotheses conditioned on the follower's trajectory by orders of magnitude.
更多查看译文
关键词
active inverse learning
AI 理解论文
溯源树
样例
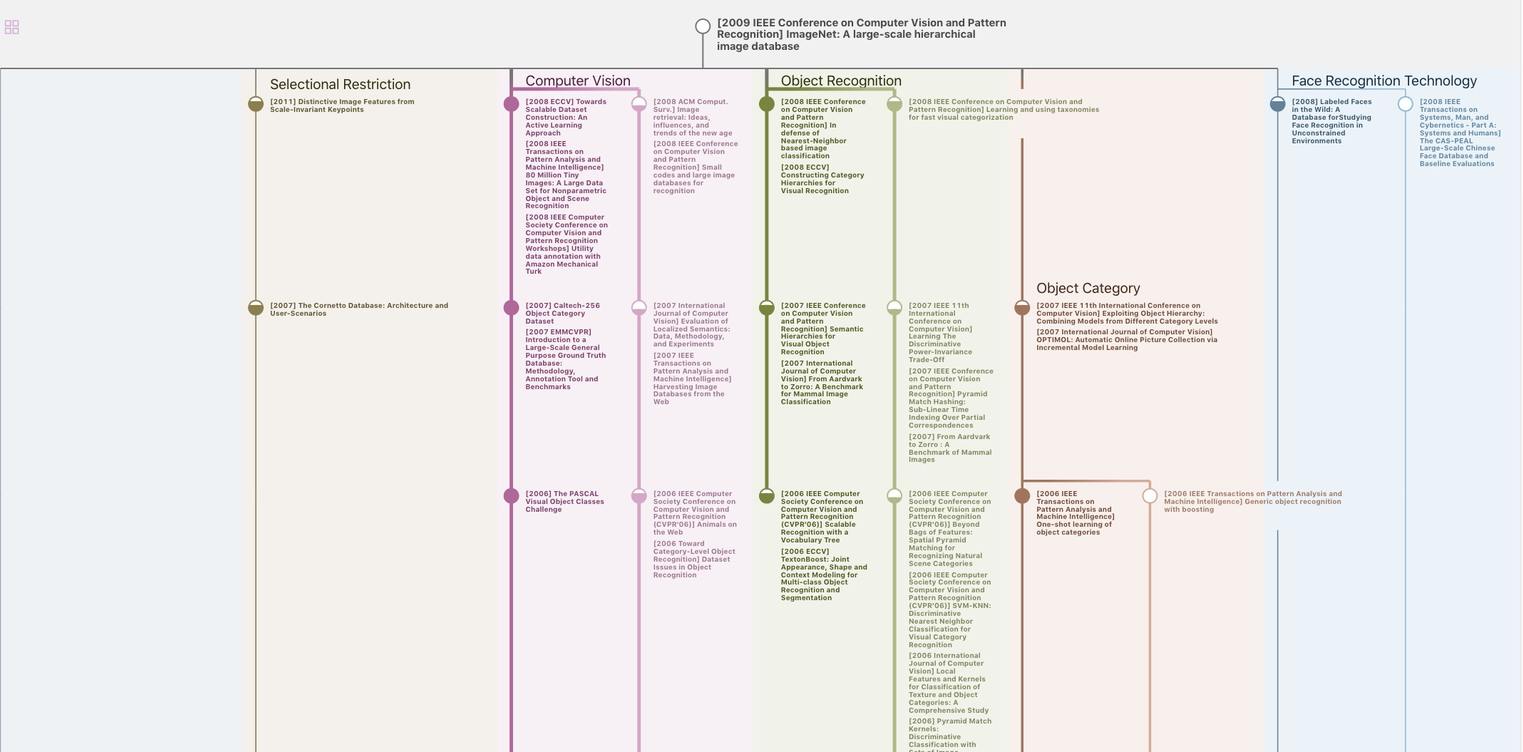
生成溯源树,研究论文发展脉络
Chat Paper
正在生成论文摘要