Community detection based on structural balance in signed networks
CoRR(2023)
摘要
In signed networks, some existing community detection methods treat negative connections as intercommunity links and positive ones as intracommunity links. However, it is important to recognize that negative links on real-world networks also play a key role in maintaining community stability. In this work, our aim is to identify communities that are not only densely connected but also harmonious or balanced in terms of the nature of their relationships. Such communities are more likely to be stable over time and less prone to conflicts. Consequently, we propose a motif-based method to identify communities by quantifying the importance of links in the local structural balance. The results in synthetic and real-world networks show that the proposed method has a higher performance in identifying the community. In addition, it demonstrates strong robustness, i.e., remains insensitive to the balance of the network, and accurately classifies communities in real-world networks.
更多查看译文
AI 理解论文
溯源树
样例
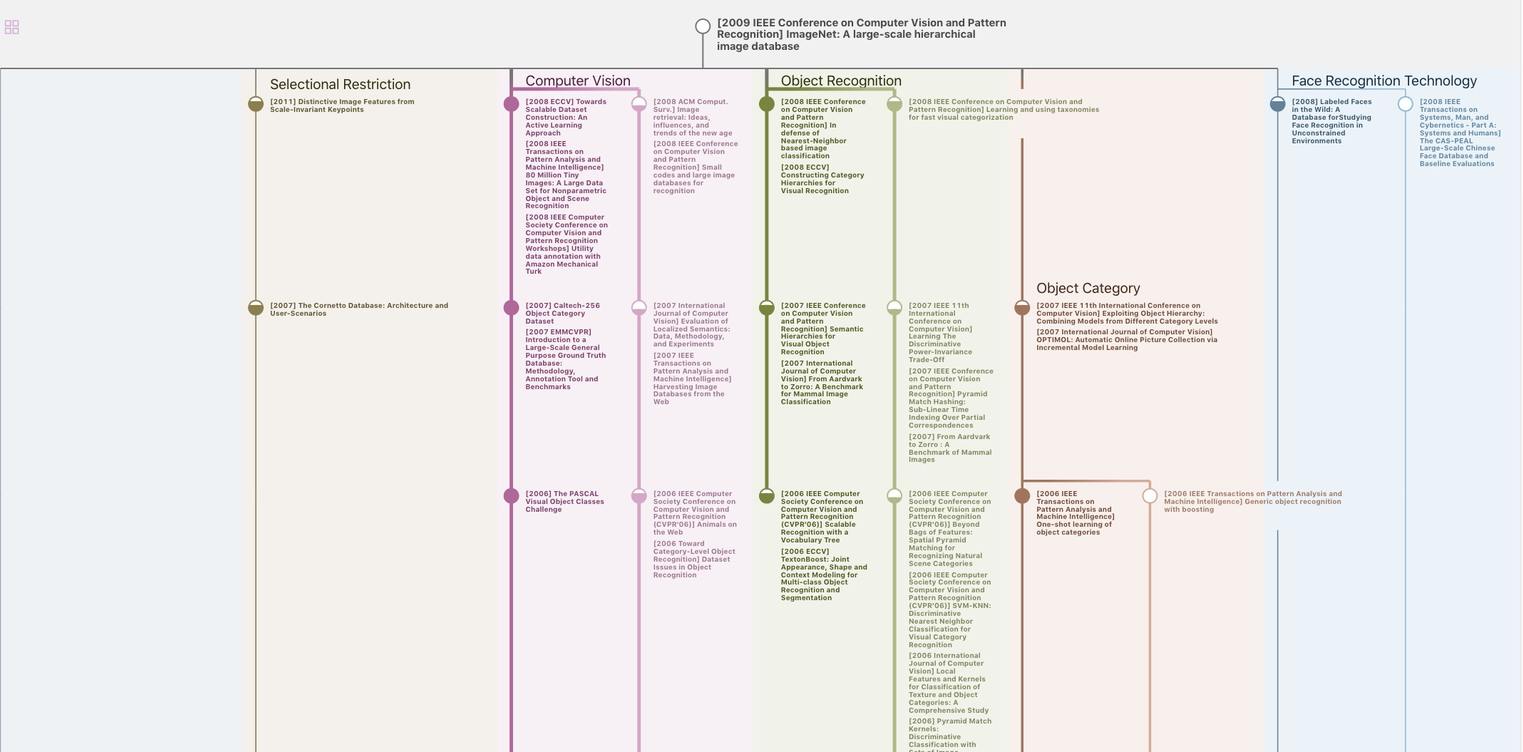
生成溯源树,研究论文发展脉络
Chat Paper
正在生成论文摘要