Teach LLMs to Personalize -- An Approach inspired by Writing Education
CoRR(2023)
摘要
Personalized text generation is an emerging research area that has attracted much attention in recent years. Most studies in this direction focus on a particular domain by designing bespoke features or models. In this work, we propose a general approach for personalized text generation using large language models (LLMs). Inspired by the practice of writing education, we develop a multistage and multitask framework to teach LLMs for personalized generation. In writing instruction, the task of writing from sources is often decomposed into multiple steps that involve finding, evaluating, summarizing, synthesizing, and integrating information. Analogously, our approach to personalized text generation consists of multiple stages: retrieval, ranking, summarization, synthesis, and generation. In addition, we introduce a multitask setting that helps the model improve its generation ability further, which is inspired by the observation in education that a student's reading proficiency and writing ability are often correlated. We evaluate our approach on three public datasets, each of which covers a different and representative domain. Our results show significant improvements over a variety of baselines.
更多查看译文
关键词
writing,personalize,llms,education
AI 理解论文
溯源树
样例
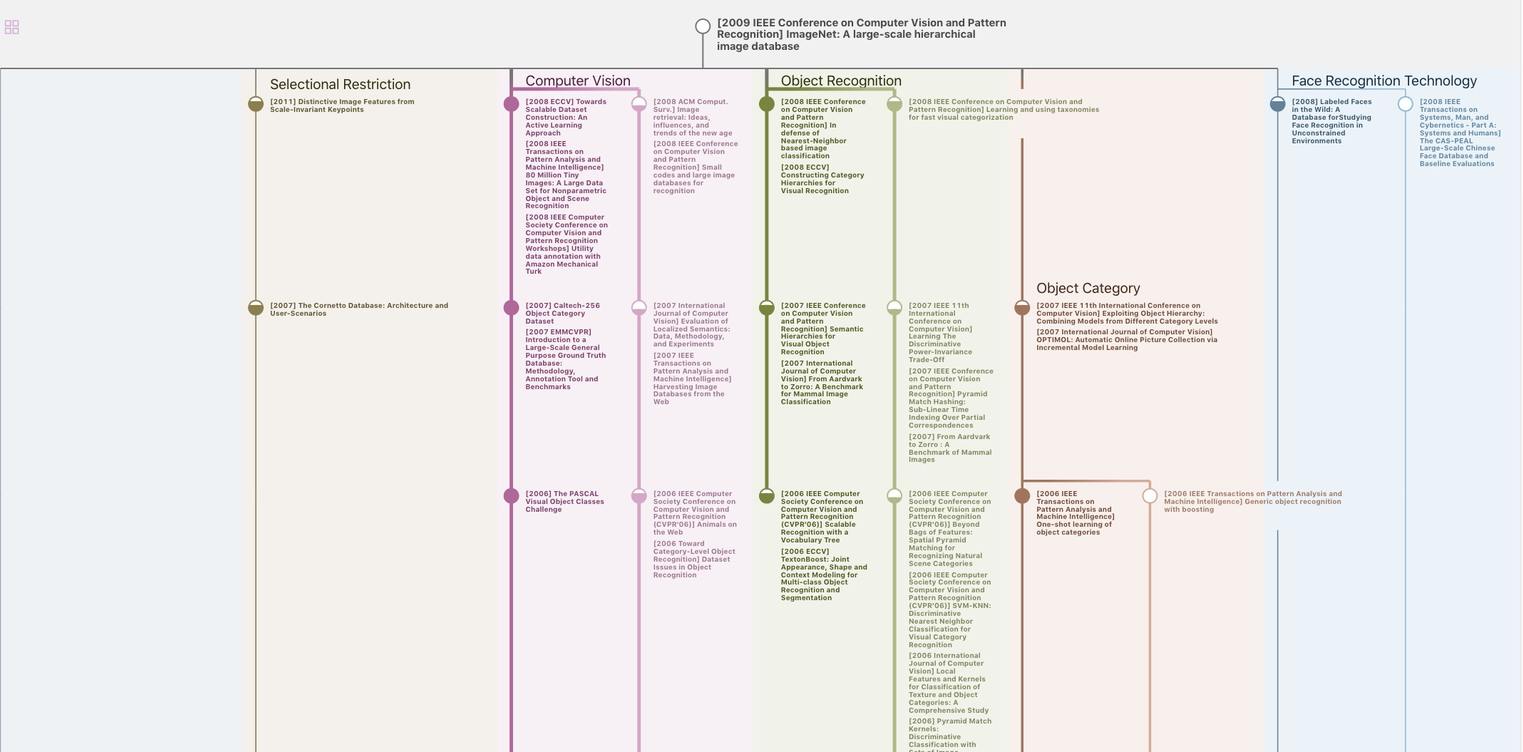
生成溯源树,研究论文发展脉络
Chat Paper
正在生成论文摘要