Bringing Artificial Intelligence to the operating room: edge computing for real-time surgical phase recognition
Surgical endoscopy(2023)
摘要
Background Automation of surgical phase recognition is a key effort toward the development of Computer Vision (CV) algorithms, for workflow optimization and video-based assessment. CV is a form of Artificial Intelligence (AI) that allows interpretation of images through a deep learning (DL)-based algorithm. The improvements in Graphic Processing Unit (GPU) computing devices allow researchers to apply these algorithms for recognition of content in videos in real-time. Edge computing, where data is collected, analyzed, and acted upon in close proximity to the collection source, is essential meet the demands of workflow optimization by providing real-time algorithm application. We implemented a real-time phase recognition workflow and demonstrated its performance on 10 Robotic Inguinal Hernia Repairs (RIHR) to obtain phase predictions during the procedure. Methods Our phase recognition algorithm was developed with 211 videos of RIHR originally annotated into 14 surgical phases. Using these videos, a DL model with a ResNet-50 backbone was trained and validated to automatically recognize surgical phases. The model was deployed to a GPU, the Nvidia® Jetson Xavier™ NX edge computing device. Results This model was tested on 10 inguinal hernia repairs from four surgeons in real-time. The model was improved using post-recording processing methods such as phase merging into seven final phases (peritoneal scoring, mesh placement, preperitoneal dissection, reduction of hernia, out of body, peritoneal closure, and transitionary idle) and averaging of frames. Predictions were made once per second with a processing latency of approximately 250 ms. The accuracy of the real-time predictions ranged from 59.8 to 78.2% with an average accuracy of 68.7%. Conclusion A real-time phase prediction of RIHR using a CV deep learning model was successfully implemented. This real-time CV phase segmentation system can be useful for monitoring surgical progress and be integrated into software to provide hospital workflow optimization. Graphical abstract
更多查看译文
关键词
operating room,edge,artificial intelligence,computing,recognition,real-time
AI 理解论文
溯源树
样例
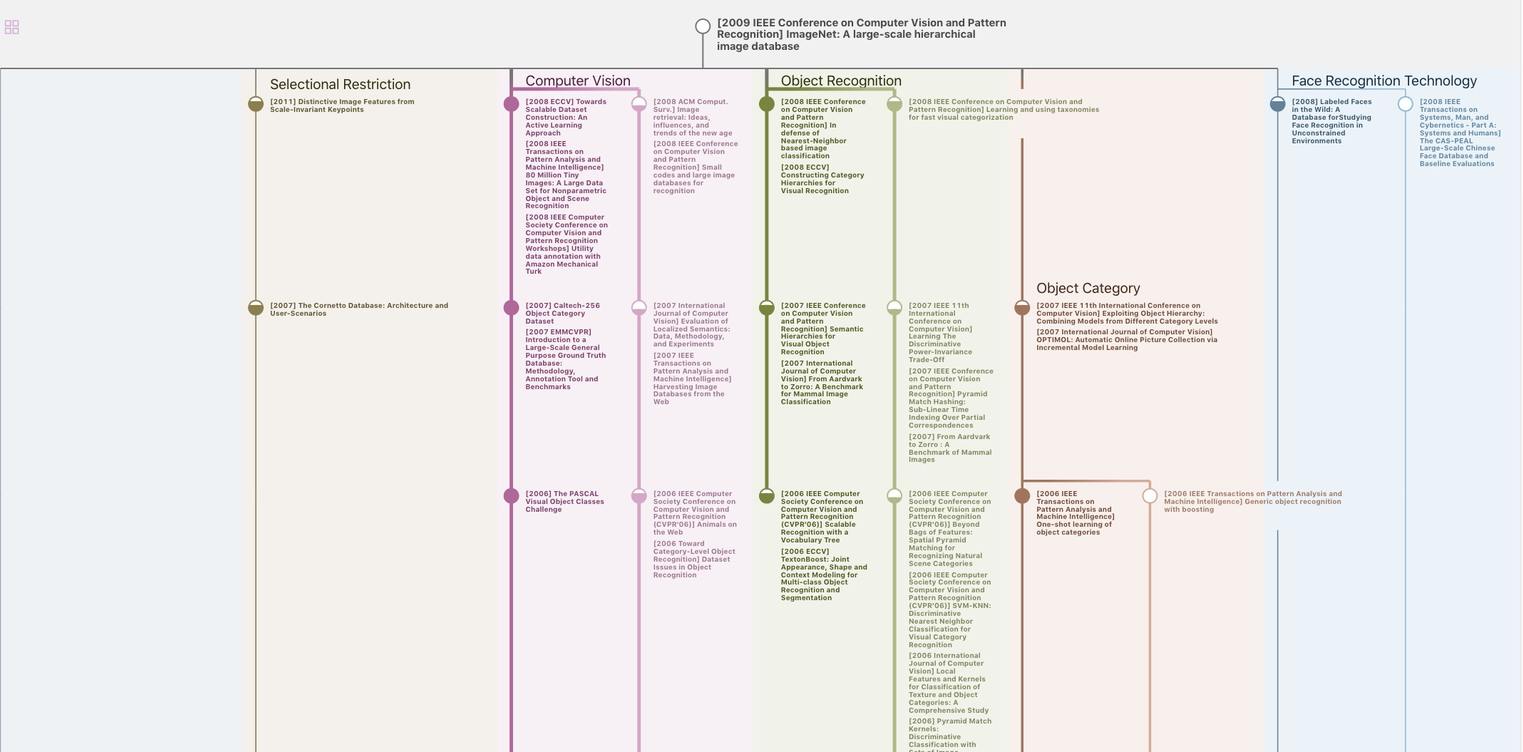
生成溯源树,研究论文发展脉络
Chat Paper
正在生成论文摘要