A Siamese Network With Node Convolution for Individualized Predictions Based on Connectivity Maps Extracted From Resting-State fMRI Data
IEEE JOURNAL OF BIOMEDICAL AND HEALTH INFORMATICS(2023)
摘要
Deep learning has demonstrated great potential for objective diagnosis of neuropsychiatric disorders based on neuroimaging data, which includes the promising resting-state functional magnetic resonance imaging (RS-fMRI). However, the insufficient sample size has long been a bottleneck for deep model training for the purpose. In this study, we proposed a Siamese network with node convolution (SNNC) for individualized predictions based on RS-fMRI data. With the involvement of Siamese network, which uses sample pair (rather than a single sample) as input, the problem of insufficient sample size can largely be alleviated. To adapt to connectivity maps extracted from RS-fMRI data, we applied node convolution to each of the two branches of the Siamese network. For regression purposes, we replaced the contrastive loss in classic Siamese network with the mean square error loss and thus enabled Siamese network to quantitatively predict label differences. The label of a test sample can be predicted based on any of the training samples, by adding the label of the training sample to the predicted label difference between them. The final prediction for a test sample in this study was made by averaging the predictions based on each of the training samples. The performance of the proposed SNNC was evaluated with age and IQ predictions based on a public dataset (Cam-CAN). The results indicated that SNNC can make effective predictions even with a sample size of as small as 40, and SNNC achieved state-of-the-art accuracy among a variety of deep models and standard machine learning approaches.
更多查看译文
关键词
RS-fMRI,siamese network,small sample size,individualized prediction,node convolution
AI 理解论文
溯源树
样例
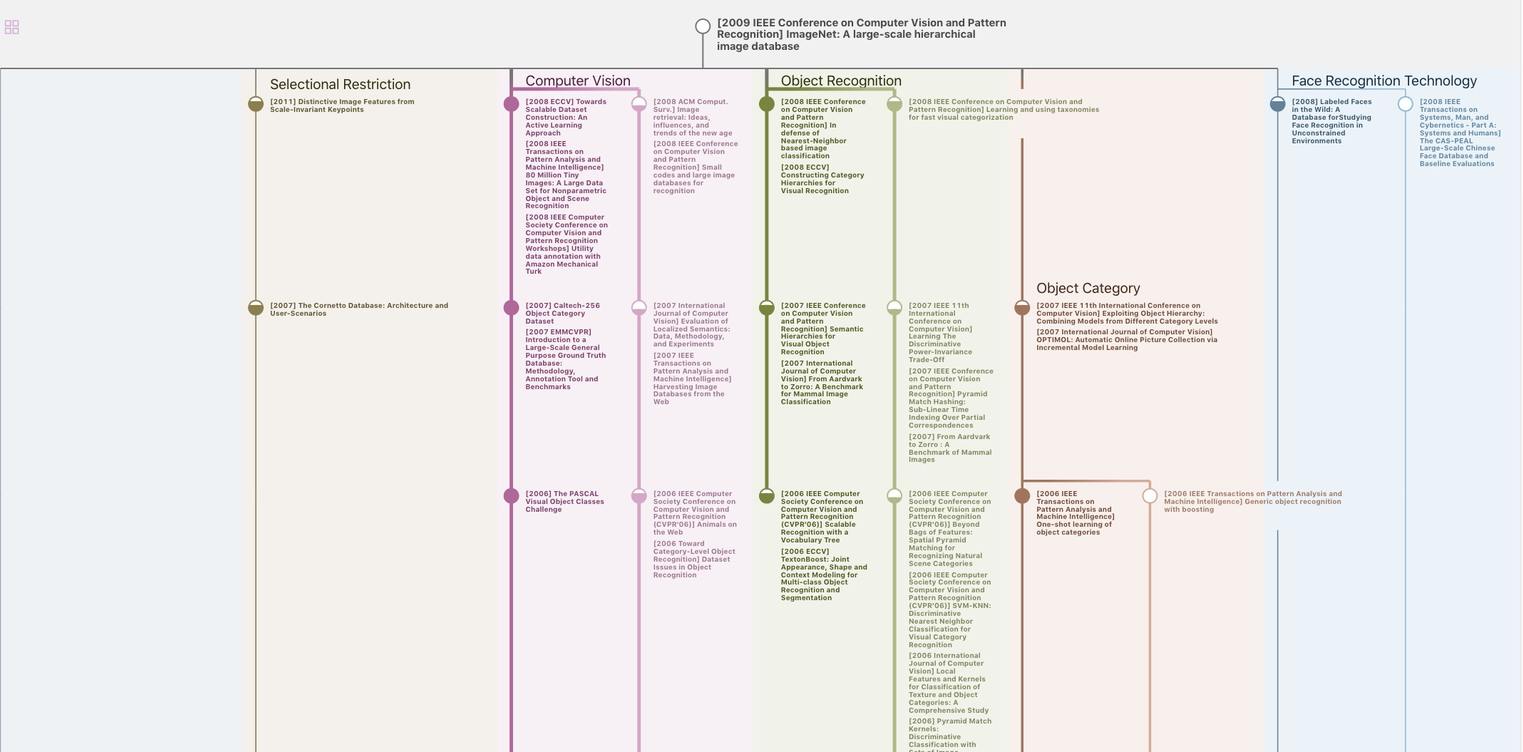
生成溯源树,研究论文发展脉络
Chat Paper
正在生成论文摘要