Informed Named Entity Recognition Decoding for Generative Language Models
CoRR(2023)
摘要
Ever-larger language models with ever-increasing capabilities are by now well-established text processing tools. Alas, information extraction tasks such as named entity recognition are still largely unaffected by this progress as they are primarily based on the previous generation of encoder-only transformer models. Here, we propose a simple yet effective approach, Informed Named Entity Recognition Decoding (iNERD), which treats named entity recognition as a generative process. It leverages the language understanding capabilities of recent generative models in a future-proof manner and employs an informed decoding scheme incorporating the restricted nature of information extraction into open-ended text generation, improving performance and eliminating any risk of hallucinations. We coarse-tune our model on a merged named entity corpus to strengthen its performance, evaluate five generative language models on eight named entity recognition datasets, and achieve remarkable results, especially in an environment with an unknown entity class set, demonstrating the adaptability of the approach.
更多查看译文
关键词
named entity recognition,entity recognition,generative language
AI 理解论文
溯源树
样例
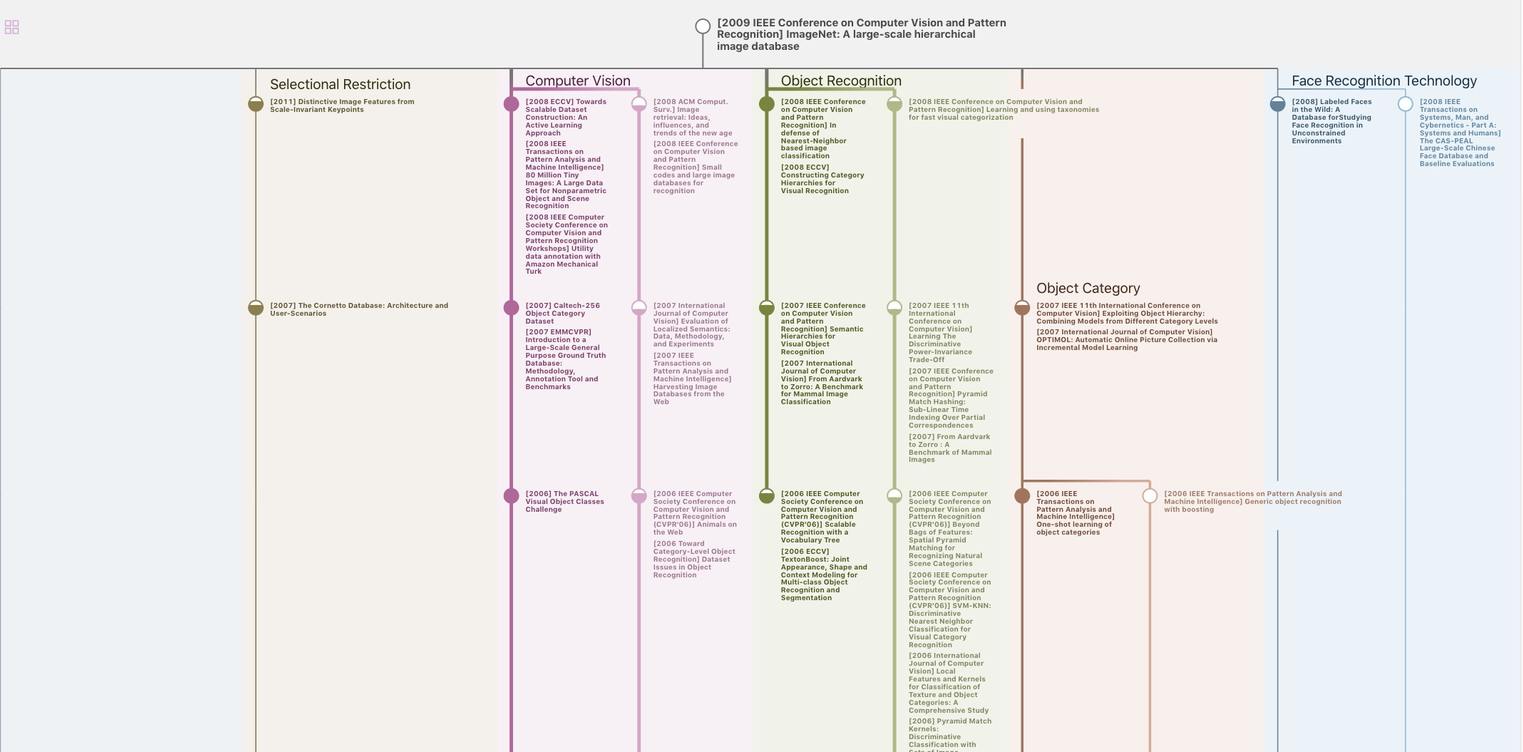
生成溯源树,研究论文发展脉络
Chat Paper
正在生成论文摘要