AttMOT: Improving Multiple-Object Tracking by Introducing Auxiliary Pedestrian Attributes
IEEE TRANSACTIONS ON NEURAL NETWORKS AND LEARNING SYSTEMS(2024)
摘要
Multiobject tracking (MOT) is a fundamental problem in computer vision with numerous applications, such as intelligent surveillance and automated driving. Despite the significant progress made in MOT, pedestrian attributes, such as gender, hairstyle, body shape, and clothing features, which contain rich and high-level information, have been less explored. To address this gap, we propose a simple, effective, and generic method to predict pedestrian attributes to support general reidentification (Re-ID) embedding. We first introduce attribute multi-object tracking (AttMOT), a large, highly enriched synthetic dataset for pedestrian tracking, containing over 80k frames and six million pedestrian identity switches (IDs) with different times, weather conditions, and scenarios. To the best of authors' knowledge, AttMOT is the first MOT dataset with semantic attributes. Subsequently, we explore different approaches to fuse Re-ID embedding and pedestrian attributes, including attention mechanisms, which we hope will stimulate the development of attribute-assisted MOT. The proposed method attribute-assisted method (AAM) demonstrates its effectiveness and generality on several representative pedestrian MOT benchmarks, including MOT17 and MOT20, through experiments on the AttMOT dataset. When applied to the state-of-the-art trackers, AAM achieves consistent improvements in multi-object tracking accuracy (MOTA), higher order tracking accuracy (HOTA), association accuracy (AssA), IDs, and IDF1 scores. For instance, on MOT17, the proposed method yields a + 1.1 MOTA, + 1.7 HOTA, and + 1.8 IDF1 improvement when used with FairMOT. To further encourage related research, we release the data and code at https://github.com/HengLan/AttMOT.
更多查看译文
关键词
Pedestrians,Target tracking,Synthetic data,Tracking,Task analysis,Feature extraction,Annotations,Attribute assistance,multiobject tracking (MOT),pedestrian attributes,synthetic dataset
AI 理解论文
溯源树
样例
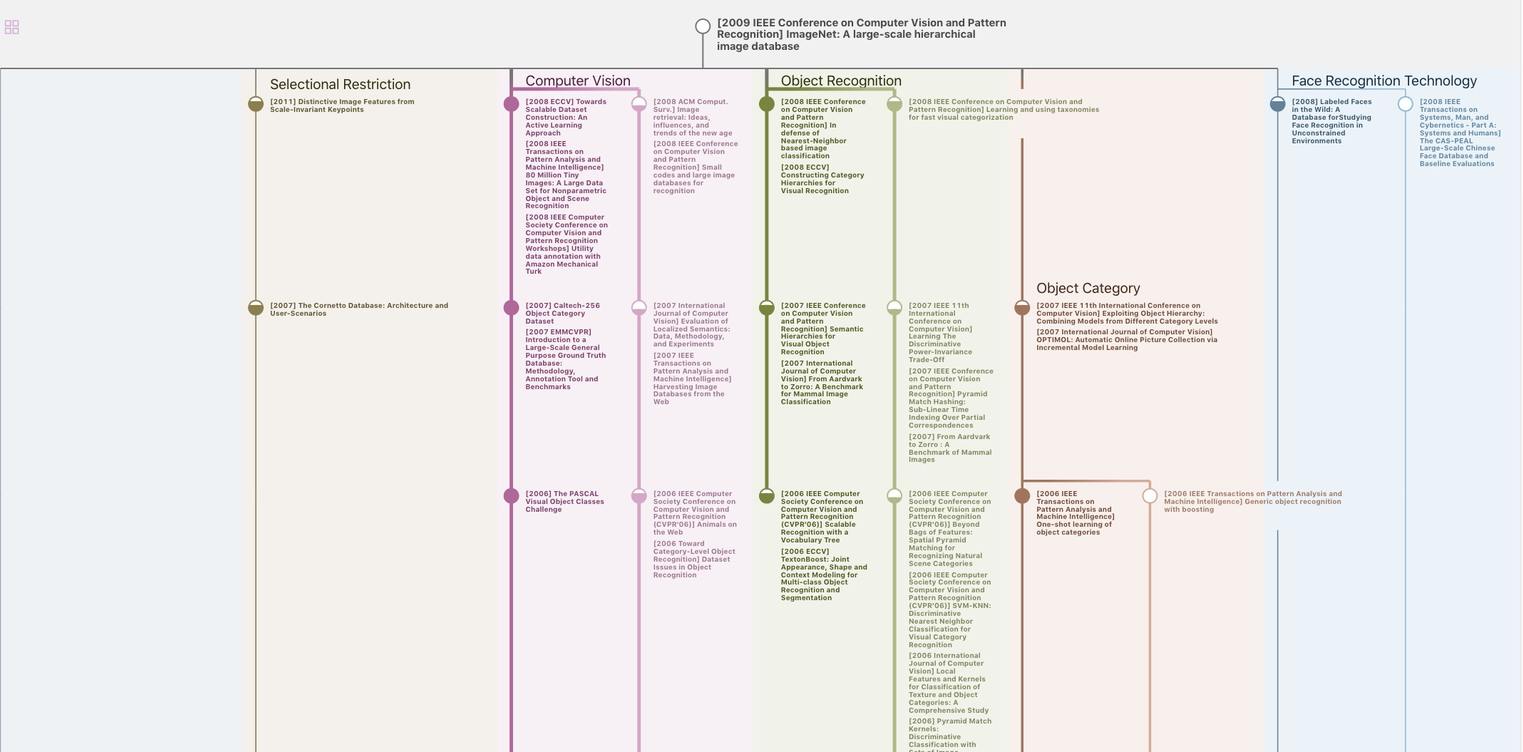
生成溯源树,研究论文发展脉络
Chat Paper
正在生成论文摘要