GRU-D-Weibull: A novel real-time individualized endpoint prediction
ARTIFICIAL INTELLIGENCE IN MEDICINE(2023)
摘要
Background: In the era of healthcare digital transformation, using electronic health record (EHR) data to generate various endpoint estimates for active monitoring is highly desirable in chronic disease management. However, traditional predictive modeling strategies leveraging well-curated data sets can have limited real-world imple-mentation potential due to various data quality issues in EHR data.Methods: We propose a novel predictive modeling approach, GRU-D-Weibull, which models Weibull distribution leveraging gated recurrent units with decay (GRU-D), for real-time individualized endpoint prediction and population level risk management using EHR data.Experiments: We systematically evaluated the performance and showcased the real-world implementability of the proposed approach through individual level endpoint prediction using a cohort of patients with chronic kidney disease stage 4 (CKD4). A total of 536 features including ICD/CPT codes, medications, lab tests, vital mea-surements, and demographics were retrieved for 6879 CKD4 patients. The performance metrics including C-index, L1-loss, Parkes' error, and predicted survival probability at time of event were compared between GRU-D -Weibull and other alternative approaches including accelerated failure time model (AFT), XGBoost based AFT (XGB(AFT)), random survival forest (RSF), and Nnet-survival. Both in-process and post-process calibrations were experimented on GRU-D-Weibull generated survival probabilities.Results: GRU-D-Weibull demonstrated C-index of-0.7 at index date, which increased to-0.77 at 4.3 years of follow-up, comparable to that of RSF. GRU-D-Weibull achieved absolute L1-loss of-1.1 years (sdR:10.95) at CKD4 index date, and a minimum of-0.45 year (sdR:10.3) at 4 years of follow-up, comparing to second-ranked RSF of-1.4 years (sdR:11.1) at index date and-0.64 years (sdR:10.26) at 4 years. Both significantly outperform competing approaches. GRU-D-Weibull constrained predicted survival probability at time of event to smaller and more fixed range than competing models throughout follow-up. Significant correlations were observed between prediction error and missing proportions of all major categories of input features at index date (Corr-0.1 to-0.3), which faded away within 1 year after index date as more data became available. Through post training recalibration, we achieved a close alignment between the predicted and observed survival probabilities across multiple prediction horizons at different time points during follow-up.Conclusion: GRU-D-Weibull shows advantages over competing methods in handling missingness commonly encountered in EHR data and providing both probability and point estimates for diverse prediction horizons during follow-up. The experiment highlights the potential of GRU-D-Weibull as a suitable candidate for individualized endpoint risk management, utilizing real-time clinical data to generate various endpoint estimates for monitoring. Additional research is warranted to evaluate the influence of different data quality aspects on prediction performance. Furthermore, collaboration with clinicians is essential to explore the integration of this approach into clinical workflows and evaluate its effects on decision-making processes and patient outcomes.
更多查看译文
关键词
Chronic kidney disease (CKD),Gated recurrent units with decay (GRU -D),Electronic health record (EHR),Deep learning,Real -time endpoint prediction,Individualized risk management
AI 理解论文
溯源树
样例
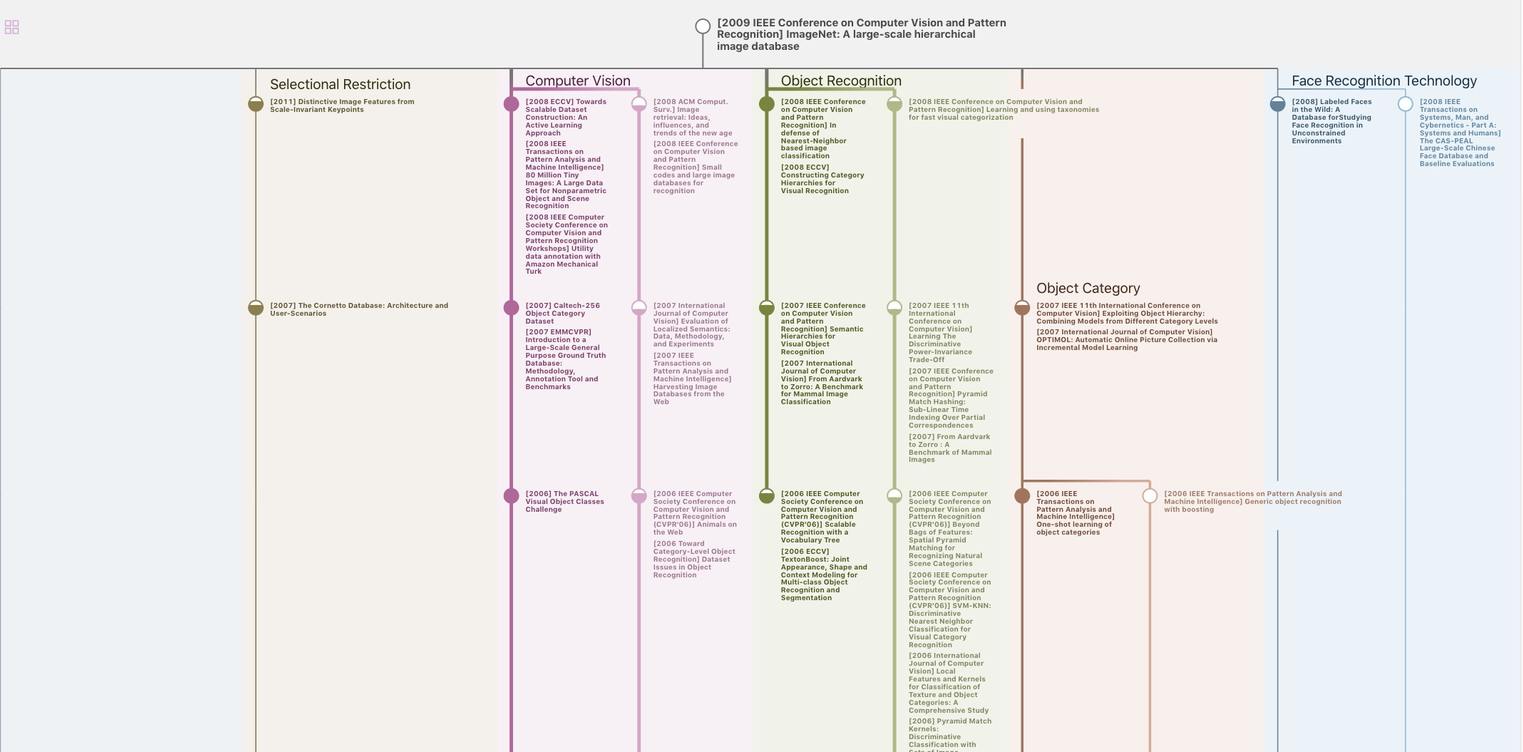
生成溯源树,研究论文发展脉络
Chat Paper
正在生成论文摘要