CBA: Improving Online Continual Learning via Continual Bias Adaptor
CoRR(2023)
摘要
Online continual learning (CL) aims to learn new knowledge and consolidate previously learned knowledge from non-stationary data streams. Due to the time-varying training setting, the model learned from a changing distribution easily forgets the previously learned knowledge and biases toward the newly received task. To address this problem, we propose a Continual Bias Adaptor (CBA) module to augment the classifier network to adapt to catastrophic distribution change during training, such that the classifier network is able to learn a stable consolidation of previously learned tasks. In the testing stage, CBA can be removed which introduces no additional computation cost and memory overhead. We theoretically reveal the reason why the proposed method can effectively alleviate catastrophic distribution shifts, and empirically demonstrate its effectiveness through extensive experiments based on four rehearsal-based baselines and three public continual learning benchmarks.
更多查看译文
关键词
online continual learning,continual bias adaptor
AI 理解论文
溯源树
样例
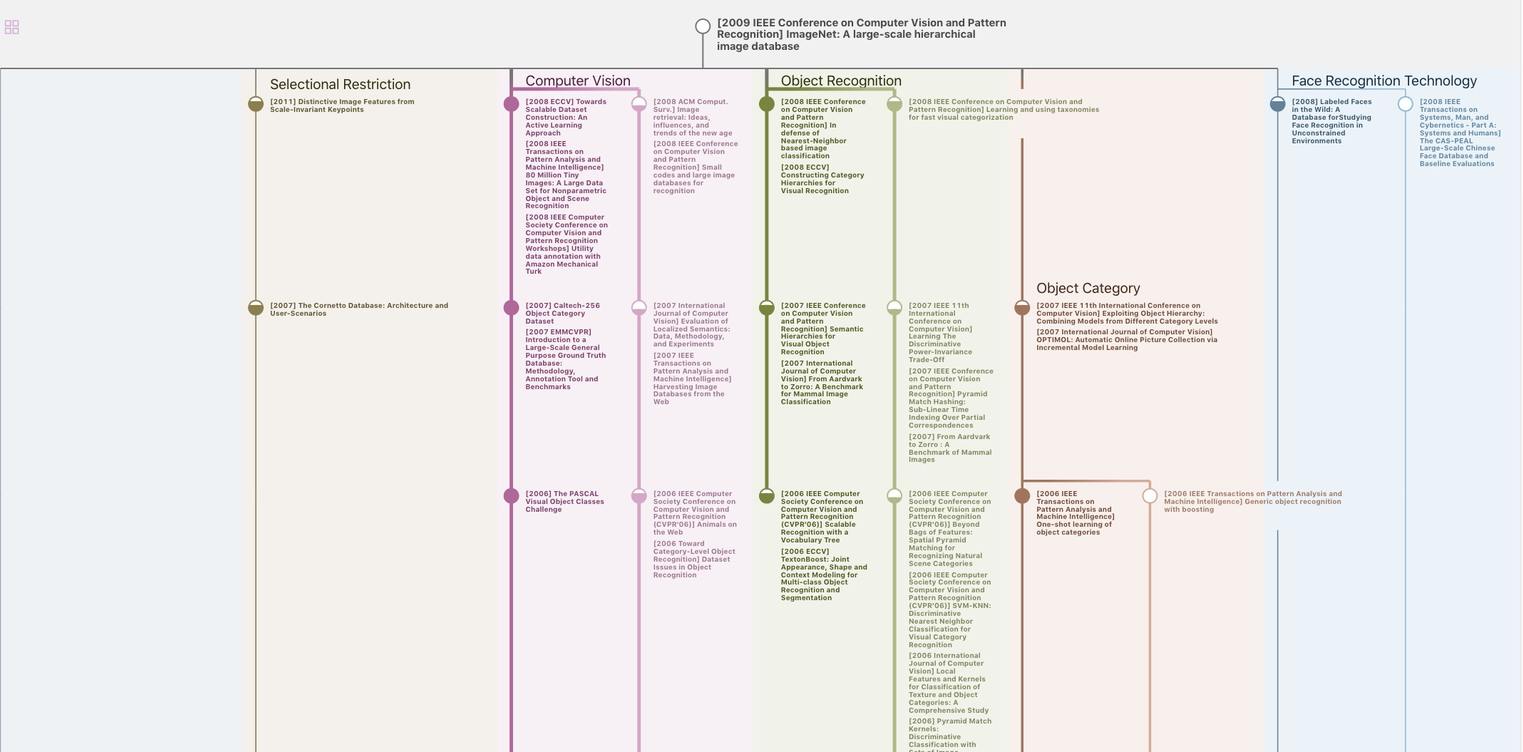
生成溯源树,研究论文发展脉络
Chat Paper
正在生成论文摘要