SoK: Realistic Adversarial Attacks and Defenses for Intelligent Network Intrusion Detection
CoRR(2023)
摘要
Machine Learning (ML) can be incredibly valuable to automate anomaly detection and cyber-attack classification, improving the way that Network Intrusion Detection (NID) is performed. However, despite the benefits of ML models, they are highly susceptible to adversarial cyber-attack examples specifically crafted to exploit them. A wide range of adversarial attacks have been created and researchers have worked on various defense strategies to safeguard ML models, but most were not intended for the specific constraints of a communication network and its communication protocols, so they may lead to unrealistic examples in the NID domain. This Systematization of Knowledge (SoK) consolidates and summarizes the state-of-the-art adversarial learning approaches that can generate realistic examples and could be used in real ML development and deployment scenarios with real network traffic flows. This SoK also describes the open challenges regarding the use of adversarial ML in the NID domain, defines the fundamental properties that are required for an adversarial example to be realistic, and provides guidelines for researchers to ensure that their future experiments are adequate for a real communication network.
更多查看译文
关键词
Realistic adversarial examples,Adversarial robustness,Cybersecurity,Intrusion detection,Machine learning
AI 理解论文
溯源树
样例
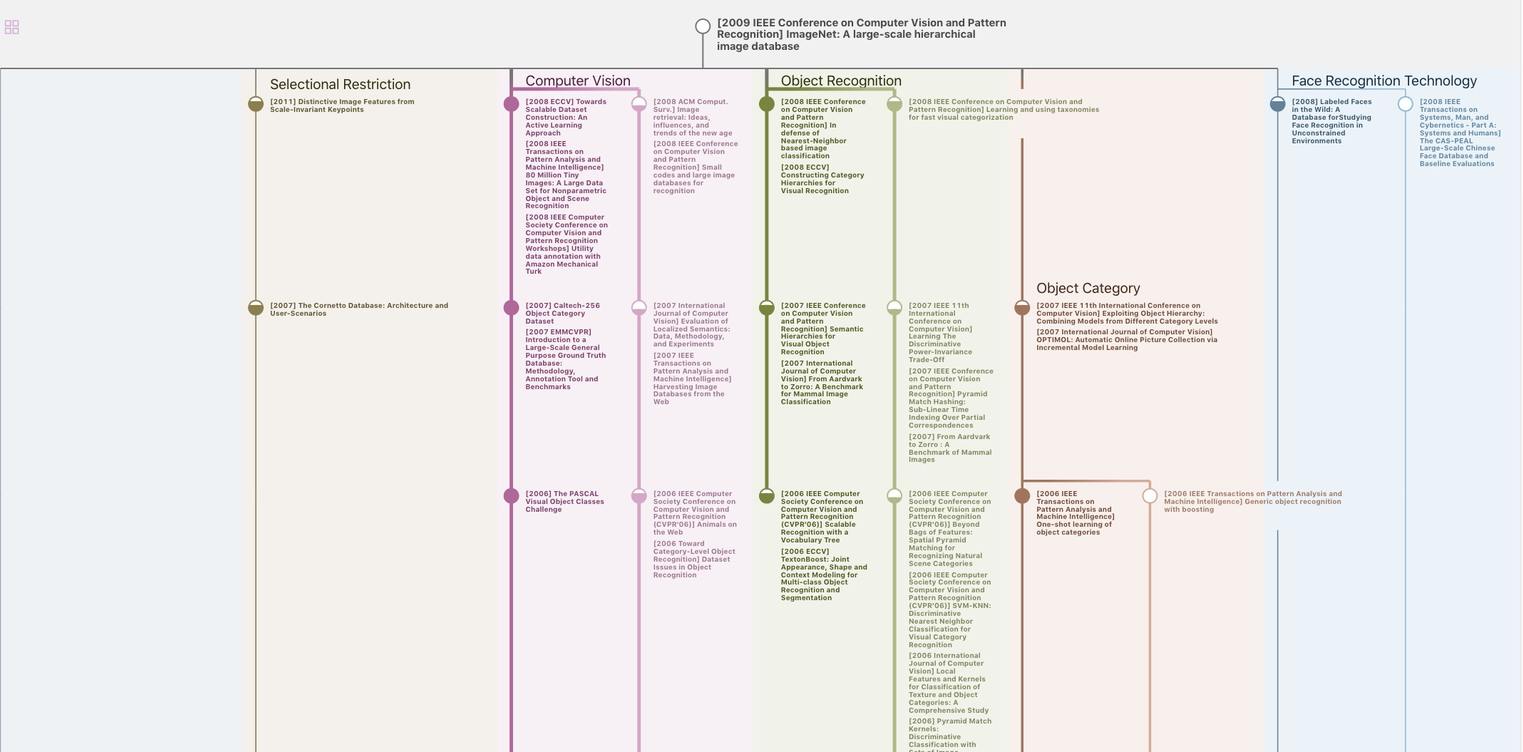
生成溯源树,研究论文发展脉络
Chat Paper
正在生成论文摘要