SAILOR: Structural Augmentation Based Tail Node Representation Learning
PROCEEDINGS OF THE 32ND ACM INTERNATIONAL CONFERENCE ON INFORMATION AND KNOWLEDGE MANAGEMENT, CIKM 2023(2023)
摘要
Graph neural networks (GNNs) have achieved state-of-the-art performance in representation learning for graphs recently. However, the effectiveness of GNNs, which capitalize on the key operation of message propagation, highly depends on the quality of the topology structure. Most of the graphs in real-world scenarios follow a long-tailed distribution on their node degrees, that is, a vast majority of the nodes in the graph are tail nodes with only a few connected edges. GNNs produce inferior node representations for tail nodes due to the lack of sufficient structural information. In the pursuit of promoting the performance of GNNs for tail nodes, we explore how the deficiency of structural information deteriorates the performance of tail nodes and propose a general structural augmentation based tail node representation learning framework, dubbed as SAILOR, which can jointly learn to augment the graph structure and extract more informative representations for tail nodes. Extensive experiments on six public benchmark datasets demonstrate that SAILOR outperforms the state-of-the-art methods for tail node representation learning.
更多查看译文
关键词
Graph neural networks,long-tailed degree distribution,graph representation learning
AI 理解论文
溯源树
样例
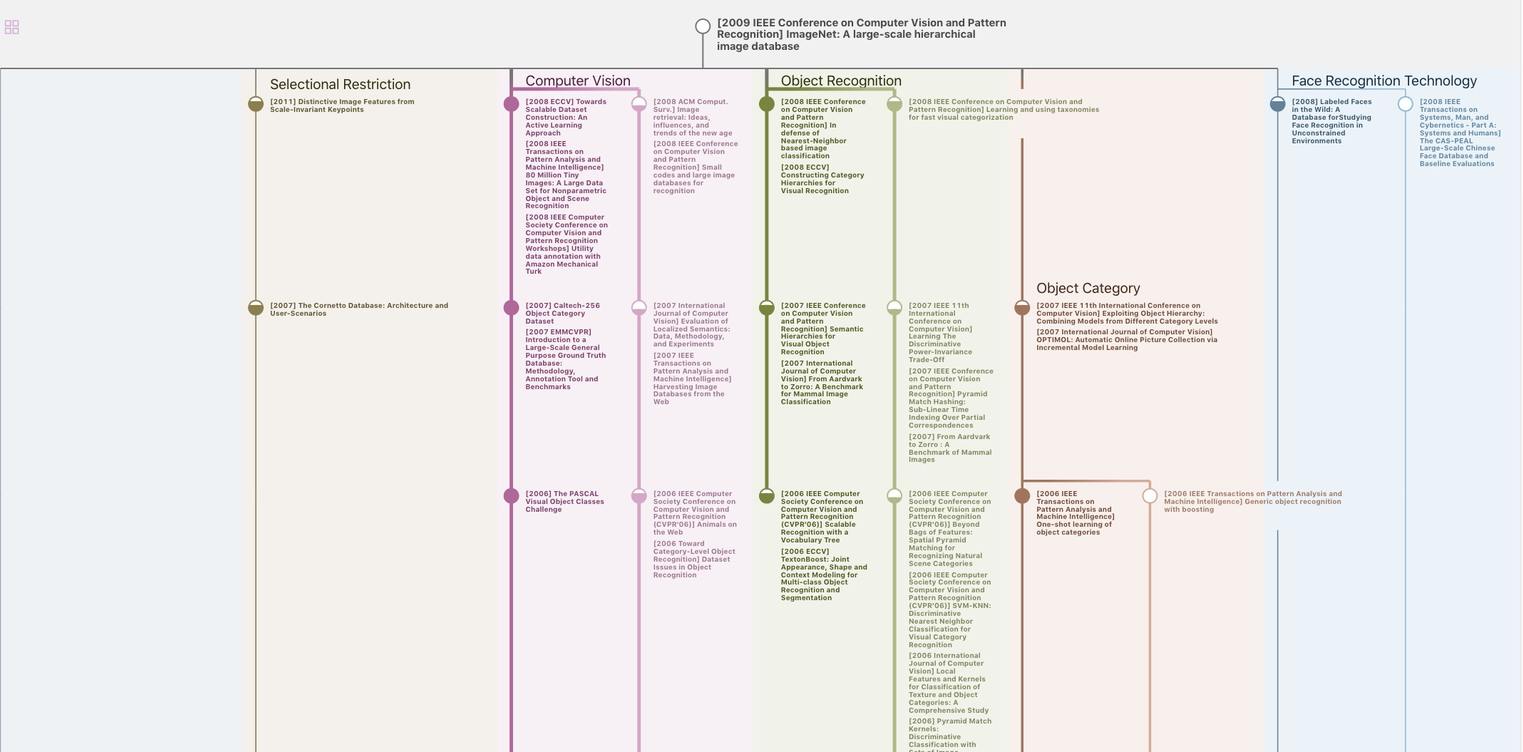
生成溯源树,研究论文发展脉络
Chat Paper
正在生成论文摘要