ADRMX: Additive Disentanglement of Domain Features with Remix Loss
CoRR(2023)
摘要
The common assumption that train and test sets follow similar distributions is often violated in deployment settings. Given multiple source domains, domain generalization aims to create robust models capable of generalizing to new unseen domains. To this end, most of existing studies focus on extracting domain invariant features across the available source domains in order to mitigate the effects of inter-domain distributional changes. However, this approach may limit the model's generalization capacity by relying solely on finding common features among the source domains. It overlooks the potential presence of domain-specific characteristics that could be prevalent in a subset of domains, potentially containing valuable information. In this work, a novel architecture named Additive Disentanglement of Domain Features with Remix Loss (ADRMX) is presented, which addresses this limitation by incorporating domain variant features together with the domain invariant ones using an original additive disentanglement strategy. Moreover, a new data augmentation technique is introduced to further support the generalization capacity of ADRMX, where samples from different domains are mixed within the latent space. Through extensive experiments conducted on DomainBed under fair conditions, ADRMX is shown to achieve state-of-the-art performance. Code will be made available at GitHub after the revision process.
更多查看译文
关键词
additive disentanglement,domain features,remix loss
AI 理解论文
溯源树
样例
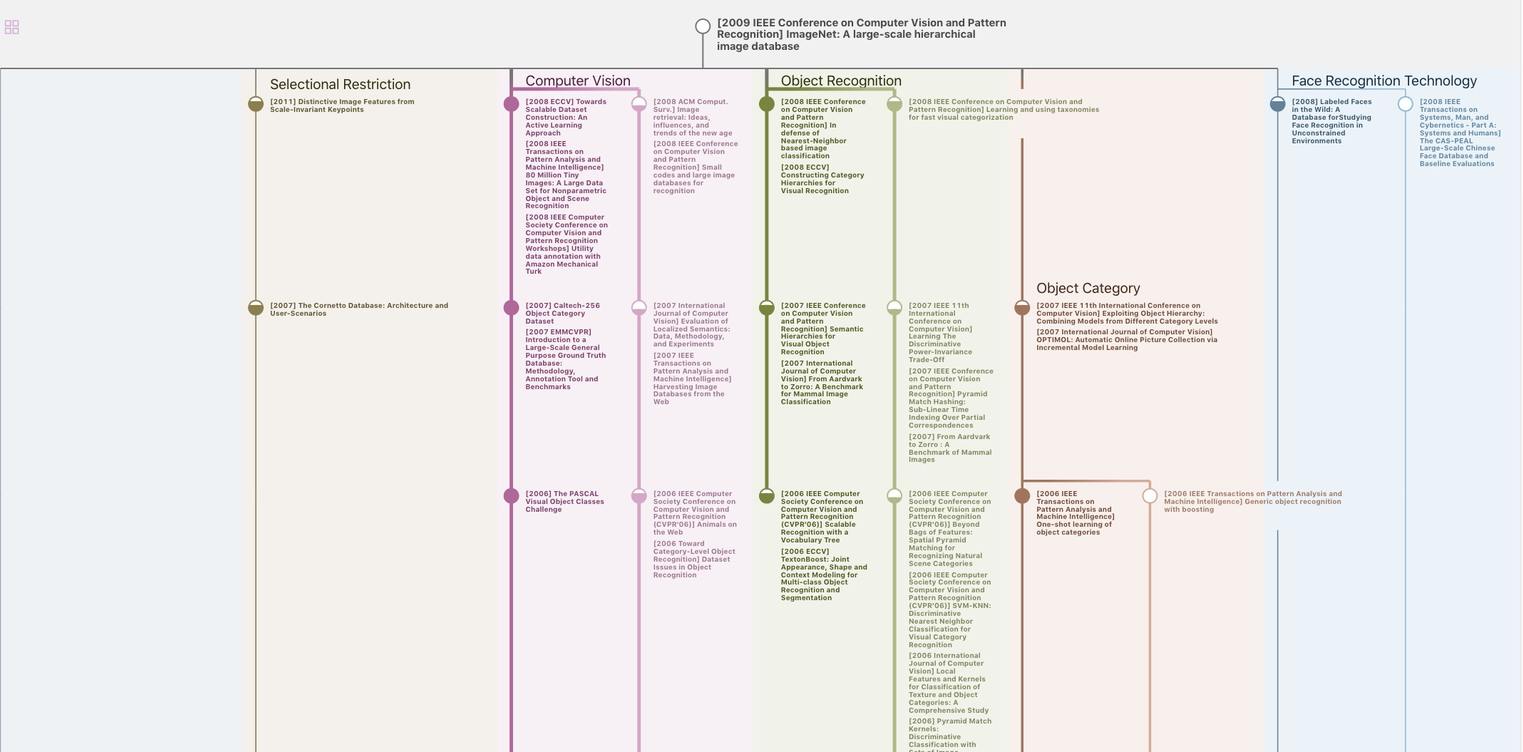
生成溯源树,研究论文发展脉络
Chat Paper
正在生成论文摘要