Alternative Pseudo-Labeling for Semi-Supervised Automatic Speech Recognition
CoRR(2023)
摘要
When labeled data is insufficient, semi-supervised learning with the pseudo-labeling technique can significantly improve the performance of automatic speech recognition. However, pseudo-labels are often noisy, containing numerous incorrect tokens. Taking noisy labels as ground-truth in the loss function results in suboptimal performance. Previous works attempted to mitigate this issue by either filtering out the nosiest pseudo-labels or improving the overall quality of pseudo-labels. While these methods are effective to some extent, it is unrealistic to entirely eliminate incorrect tokens in pseudo-labels. In this work, we propose a novel framework named alternative pseudo-labeling to tackle the issue of noisy pseudo-labels from the perspective of the training objective. The framework comprises several components. Firstly, a generalized CTC loss function is introduced to handle noisy pseudo-labels by accepting alternative tokens in the positions of incorrect tokens. Applying this loss function in pseudo-labeling requires detecting incorrect tokens in the predicted pseudo-labels. In this work, we adopt a confidence-based error detection method that identifies the incorrect tokens by comparing their confidence scores with a given threshold, thus necessitating the confidence score to be discriminative. Hence, the second proposed technique is the contrastive CTC loss function that widens the confidence gap between the correctly and incorrectly predicted tokens, thereby improving the error detection ability. Additionally, obtaining satisfactory performance with confidence-based error detection typically requires extensive threshold tuning. Instead, we propose an automatic thresholding method that uses labeled data as a proxy for determining the threshold, thus saving the pain of manual tuning.
更多查看译文
关键词
speech,recognition,pseudo-labeling,semi-supervised
AI 理解论文
溯源树
样例
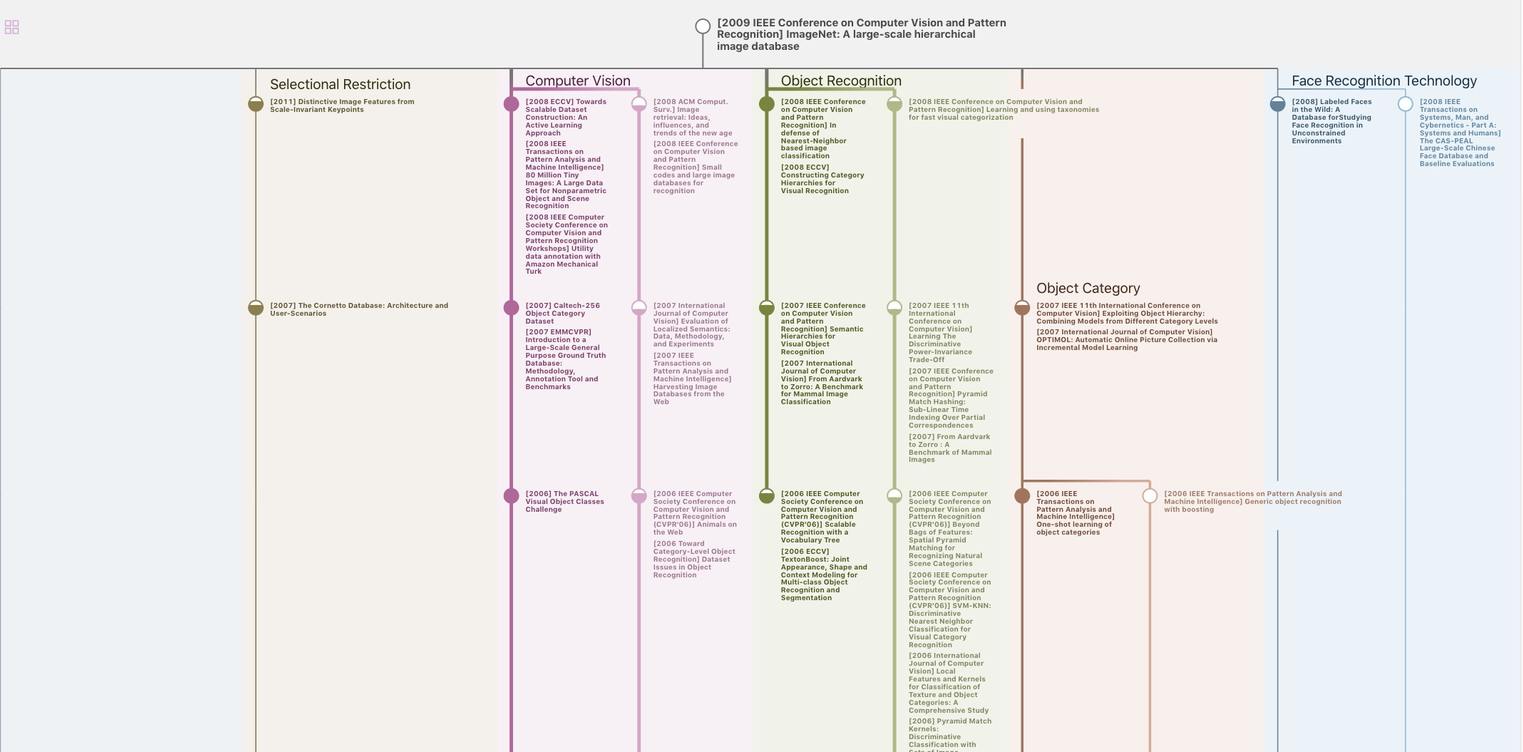
生成溯源树,研究论文发展脉络
Chat Paper
正在生成论文摘要