How complex is the microarray dataset? A novel data complexity metric for biological high-dimensional microarray data
CoRR(2023)
摘要
Data complexity analysis quantifies the hardness of constructing a predictive model on a given dataset. However, the effectiveness of existing data complexity measures can be challenged by the existence of irrelevant features and feature interactions in biological micro-array data. We propose a novel data complexity measure, depth, that leverages an evolutionary inspired feature selection algorithm to quantify the complexity of micro-array data. By examining feature subsets of varying sizes, the approach offers a novel perspective on data complexity analysis. Unlike traditional metrics, depth is robust to irrelevant features and effectively captures complexity stemming from feature interactions. On synthetic micro-array data, depth outperforms existing methods in robustness to irrelevant features and identifying complexity from feature interactions. Applied to case-control genotype and gene-expression micro-array datasets, the results reveal that a single feature of gene-expression data can account for over 90% of the performance of multi-feature model, confirming the adequacy of the commonly used differentially expressed gene (DEG) feature selection method for the gene expression data. Our study also demonstrates that constructing predictive models for genotype data is harder than gene expression data. The results in this paper provide evidence for the use of interpretable machine learning algorithms on microarray data.
更多查看译文
关键词
microarray dataset,novel dataset complexity,high-dimensional
AI 理解论文
溯源树
样例
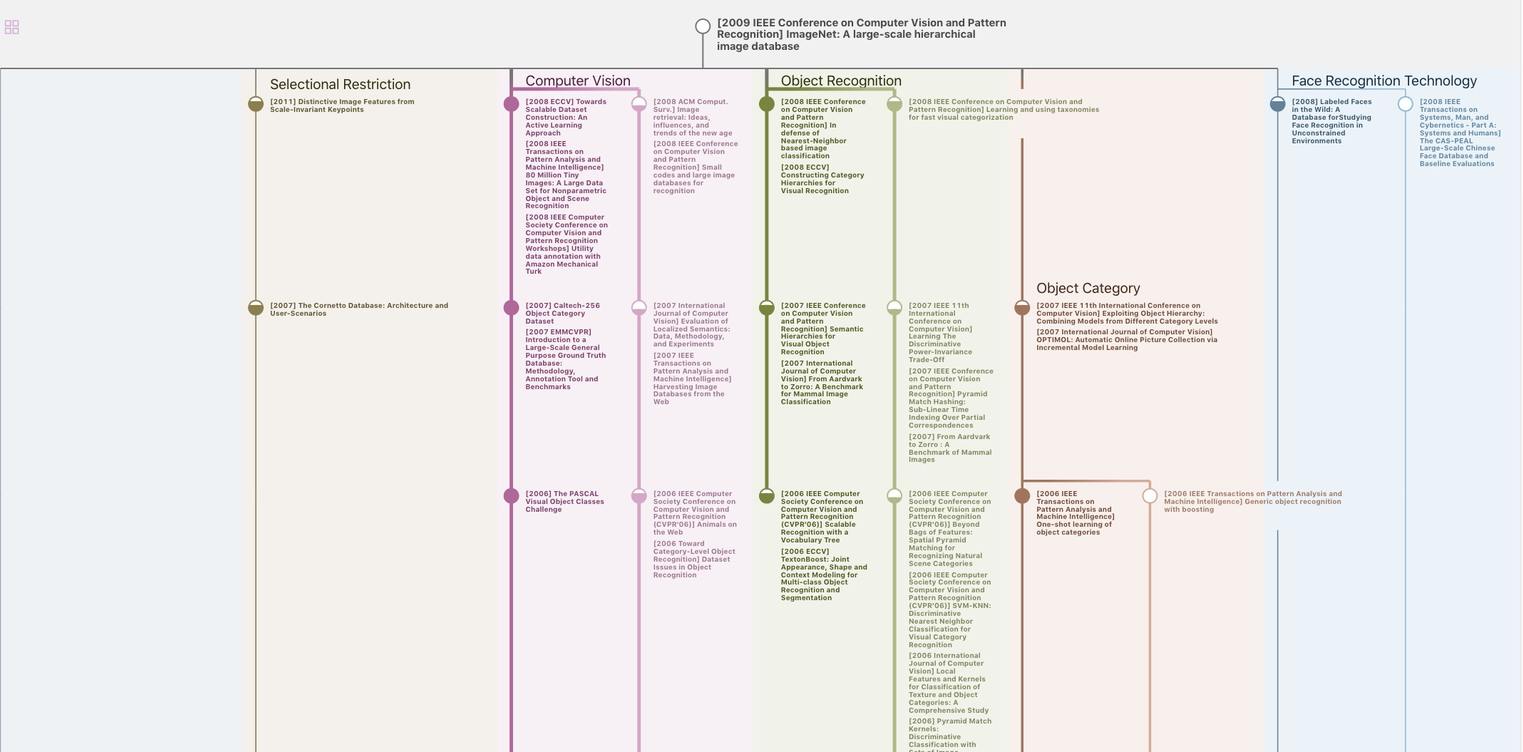
生成溯源树,研究论文发展脉络
Chat Paper
正在生成论文摘要