Descriptive complexity for neural networks via Boolean networks
CoRR(2023)
摘要
We investigate the descriptive complexity of a class of neural networks with unrestricted topologies and piecewise polynomial activation functions. We consider the general scenario where the running time is unlimited and floating-point numbers are used for simulating reals. We characterize a class of these neural networks with a rule-based logic for Boolean networks. In particular, we show that the sizes of the neural networks and the corresponding Boolean rule formulae are polynomially related. In fact, in the direction from Boolean rules to neural networks, the blow-up is only linear. We also analyze the delays in running times due to the translations. In the translation from neural networks to Boolean rules, the time delay is polylogarithmic in the neural network size and linear in time. In the converse translation, the time delay is linear in both factors.
更多查看译文
关键词
boolean networks,descriptive complexity,neural networks
AI 理解论文
溯源树
样例
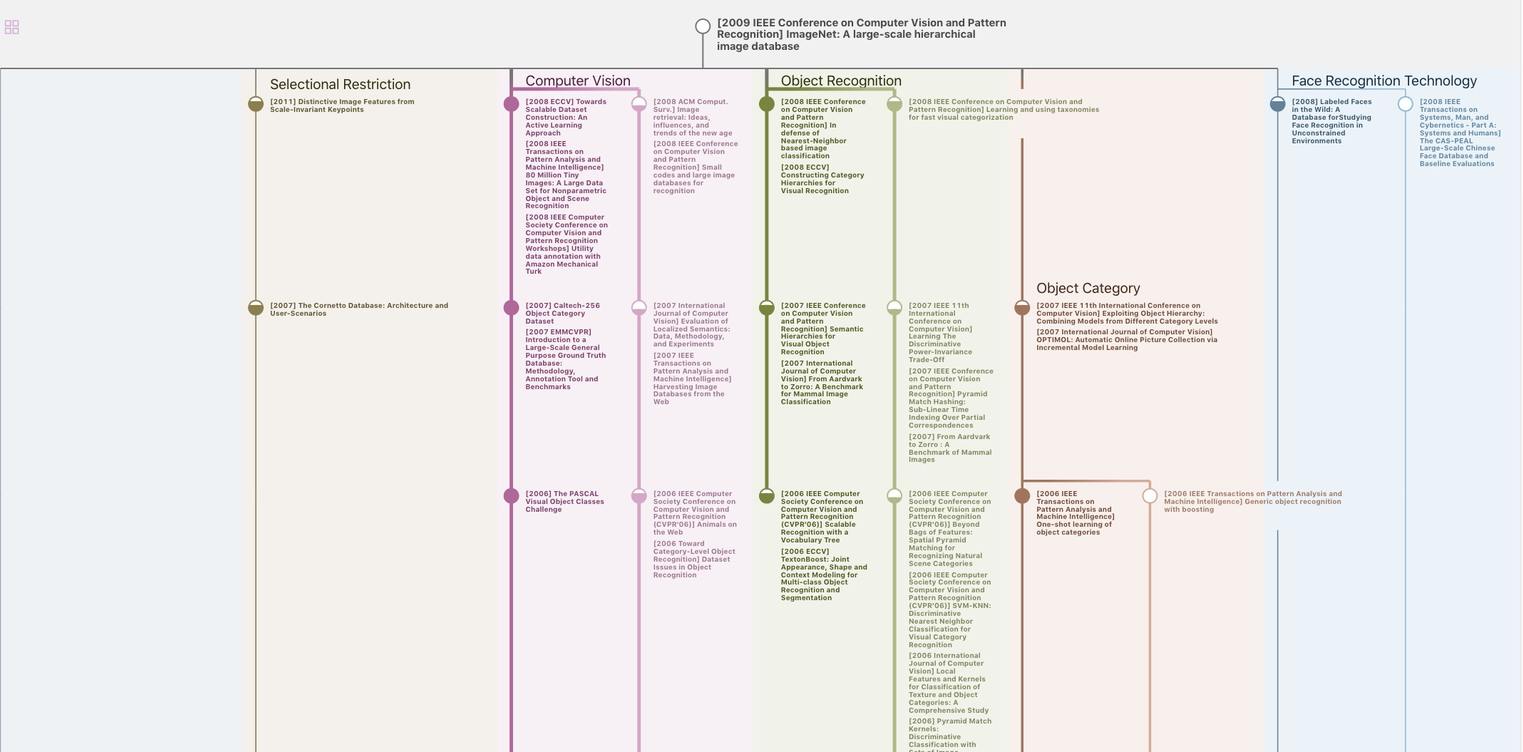
生成溯源树,研究论文发展脉络
Chat Paper
正在生成论文摘要